Application of MRI Radiomics-Based Machine Learning Model to Improve Contralateral BI-RADS 4 Lesion Assessment
FRONTIERS IN ONCOLOGY(2020)
摘要
Objective This study aimed to explore the potential of magnetic resonance imaging (MRI) radiomics-based machine learning to improve assessment and diagnosis of contralateral Breast Imaging Reporting and Data System (BI-RADS) category 4 lesions in women with primary breast cancer. Materials and Methods A total of 178 contralateral BI-RADS 4 lesions (97 malignant and 81 benign) collected from 178 breast cancer patients were involved in our retrospective dataset. T1 + C and T2 weighted images were used for radiomics analysis. These lesions were randomly assigned to the training (n = 124) dataset and an independent testing dataset (n = 54). A three-dimensional semi-automatic segmentation method was performed to segment lesions depicted on T2 and T1 + C images, 1,046 radiomic features were extracted from each segmented region, and a least absolute shrinkage and operator feature selection method reduced feature dimensionality. Three support vector machine (SVM) classifiers were trained to build classification models based on the T2, T1 + C, and fusion image features, respectively. The diagnostic performance of each model was evaluated and tested using the independent testing dataset. The area under the receiver operating characteristic curve (AUC) was used as a performance metric. Results The T1+C image feature-based model and T2 image feature-based model yielded AUCs of 0.71 +/- 0.07 and 0.69 +/- 0.07 respectively, and the difference between them was not significant (P > 0.05). After fusing T1 + C and T2 imaging features, the proposed model's AUC significantly improved to 0.77 +/- 0.06 (P < 0.001). The fusion model yielded an accuracy of 74.1%, which was higher than that of the T1 + C (66.7%) and T2 (59.3%) image feature-based models. Conclusion The MRI radiomics-based machine learning model is a feasible method to assess contralateral BI-RADS 4 lesions. T2 and T1 + C image features provide complementary information in discriminating benign and malignant contralateral BI-RADS 4 lesions.
更多查看译文
关键词
MRI,contralateral breast cancer,radiomics,machine learning,Breast Imaging Reporting and Data System category 4
AI 理解论文
溯源树
样例
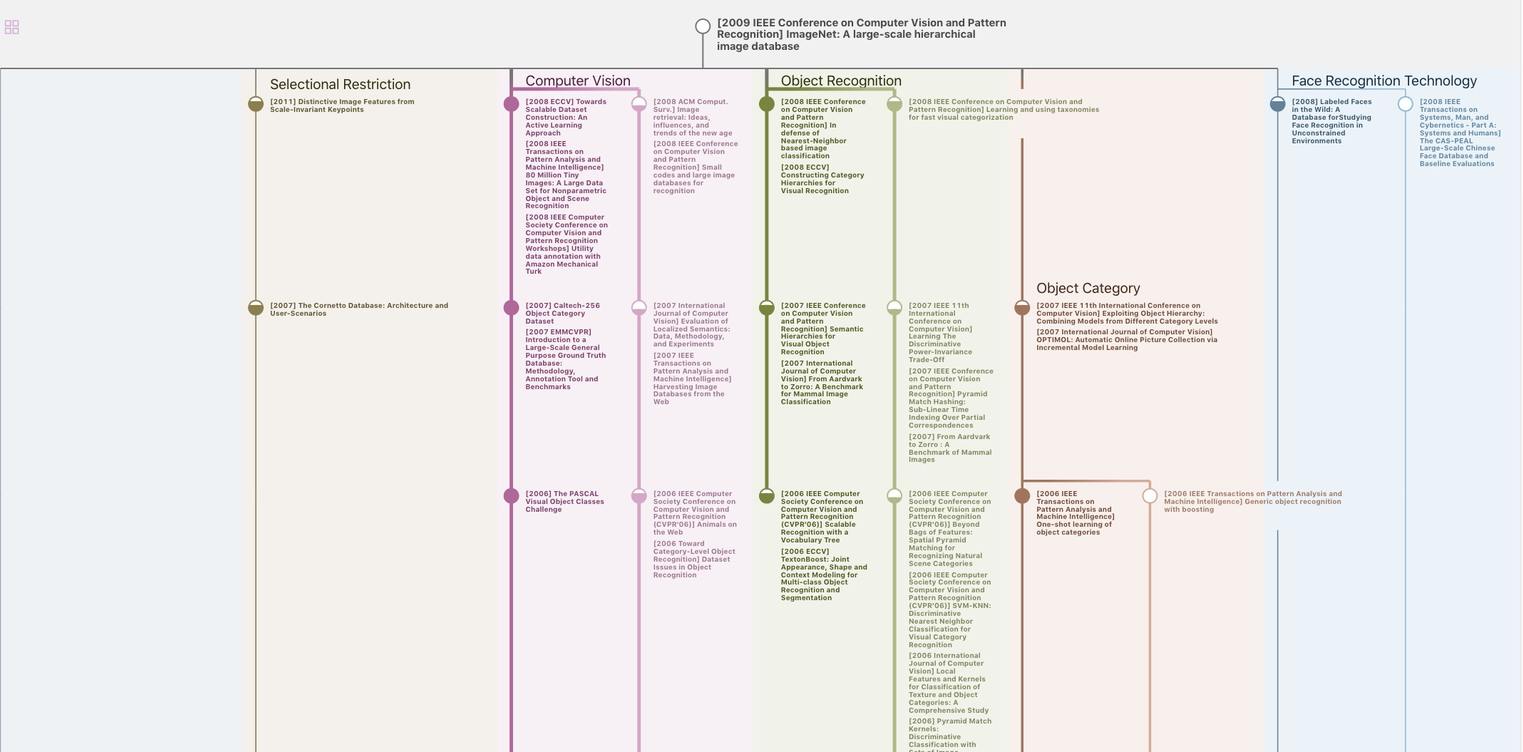
生成溯源树,研究论文发展脉络
Chat Paper
正在生成论文摘要