Early Adaptive Evaluation Scheme for Data-Driven Calibration in Forest Fire Spread Prediction.
international conference on conceptual structures(2020)
摘要
Forest fires severally affect many ecosystems every year, leading to large environmental damages, casualties and economic losses. Established and emerging technologies are used to help wildfire analysts determine fire behavior and spread aiming at a more accurate prediction results and efficient use of resources in fire fighting. Natural hazards simulations need to deal with data input uncertainty and their impact on prediction results, usually resorting to compute-intensive calibration techniques. In this paper, we propose a new evaluation technique capable of reducing the overall calibration time by 60% when compared to the current data-driven approaches. This is achieved by means of the proposed adaptive evaluation technique based on a periodic monitoring of the fire spread prediction error epsilon estimated by the normalized symmetric difference for each simulation run. Our new strategy avoid wasting too much computing time running unfit individuals thanks to an early adaptive evaluation.
更多查看译文
关键词
Forest fires, Urgent computing, Data uncertainty, Data driven prediction
AI 理解论文
溯源树
样例
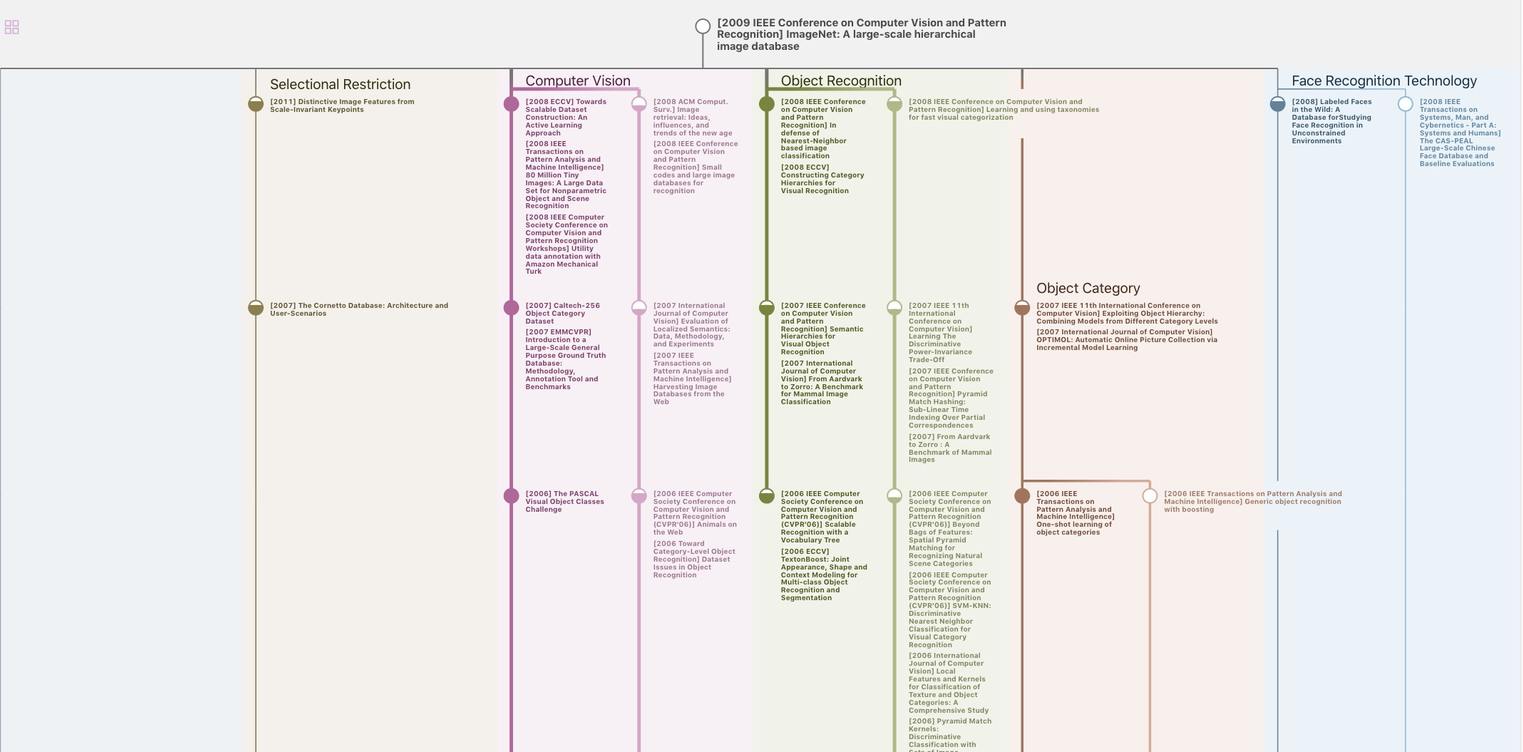
生成溯源树,研究论文发展脉络
Chat Paper
正在生成论文摘要