Automatic Target Recognition Based on Alignments of Three-Dimensional Interferometric ISAR Images and CAD Models
IEEE Transactions on Aerospace and Electronic Systems(2020)
摘要
Inverse synthetic aperture radar (ISAR) is capable of producing 2-D and 3-D images of non-cooperative targets. Compared with 2-D ISAR images, 3-D ISAR reconstructions can provide not only range and cross-range information, but also the information about the third dimension of the target, which is of great significance for automatic target recognition (ATR) and even more specifically to the case of non-cooperative target recognition. The alignment between the point-like 3-D ISAR reconstructions of targets and the targets' models, such as computer aided design (CAD) models, becomes one of the essential issues in ATR that uses 3-D ISAR reconstructions. In this article, we propose an approach to address the alignment problem. The alignment problem can be decomposed into two steps, a coarse alignment and an accurate alignment. The coarse alignment can be accomplished by means of the principal component analysis (PCA), whereas the accurate alignment can be achieved by iterative closest point algorithm. In this article, the 180° ambiguity issue in PCA is discussed, and a corresponding robust solution is proposed. Moreover, the k-d tree is utilized to accelerate the searching for the pairs of correspondences, both in coarse and accurate alignment steps. In order to simulate real radar scenarios, target self-occlusion is also taken into consideration. The experimental results based on target's scattering point model simulation as well as the electromagnetic simulation in different radar scenarios verify the validity of the proposed method.
更多查看译文
关键词
Automatic target recognition (ATR),CAD model,inverse synthetic aperture radar (ISAR),iterative closest point (ICP),non-cooperative target recognition (NCTR),point cloud alignment,principal component analysis (PCA),three-dimensional (3-D) ISAR reconstruction
AI 理解论文
溯源树
样例
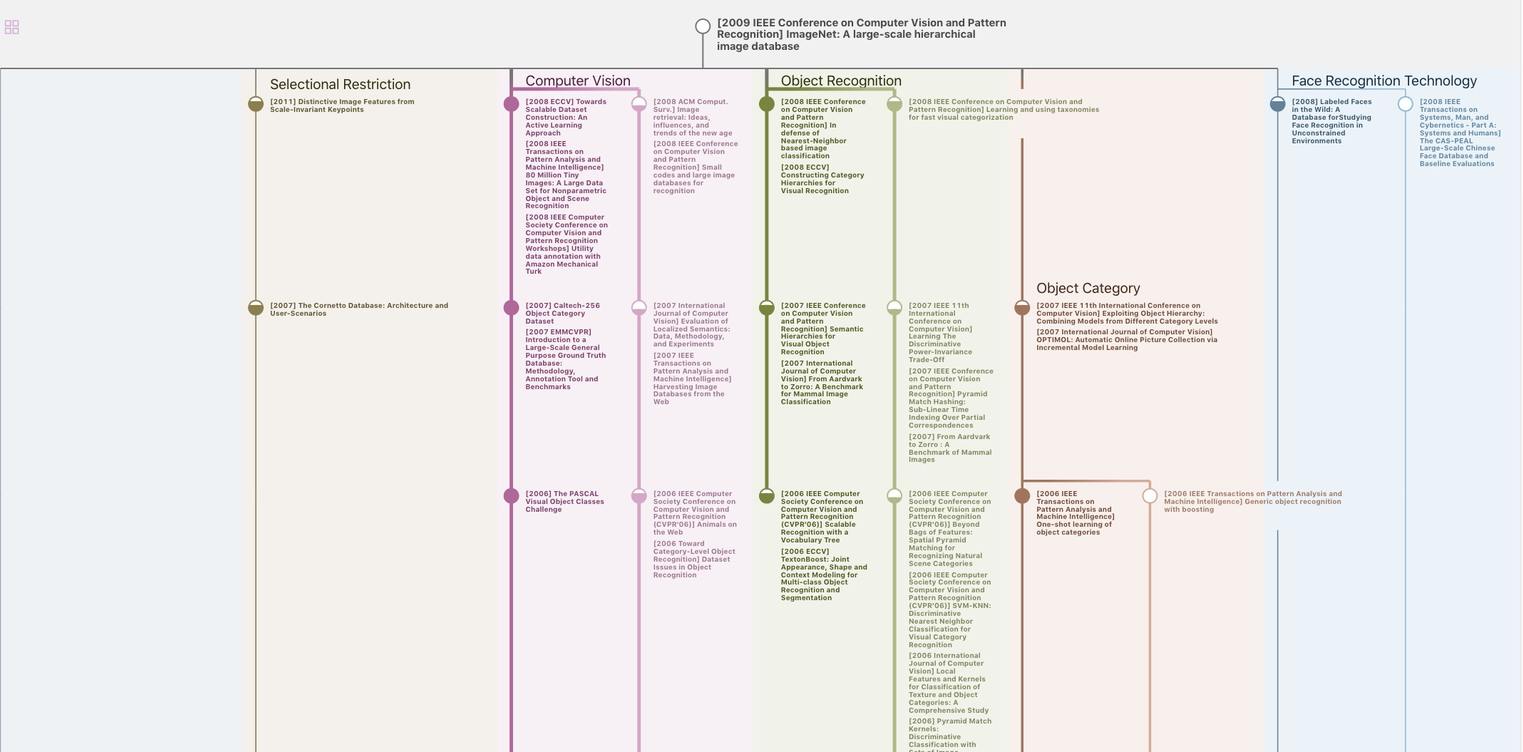
生成溯源树,研究论文发展脉络
Chat Paper
正在生成论文摘要