A Multi-Factor Data-Driven Prediction Model For Cyanobacteria Blooms In Lakes And Reservoirs
DESALINATION AND WATER TREATMENT(2020)
摘要
The generation process of cyanobacteria blooms in lakes and reservoirs is complex. Many influencing factors with time-varying characteristics make predictions more difficult. This study proposed a systematic multifactor data-driven prediction model. Through this systematic method, a prediction model could be built with fewer key influencing factors for cyanobacteria bloom in lakes and reservoirs. First, we introduced a definition of the correlation coefficient and synthesized the consistency of the changing trend on a time series and the structural similarity of state characteristics to select the key factors influencing cyanobacteria blooms in lakes and reservoirs. We used an improved wavelet threshold method to filter the data noise involved in modeling. After the nonlinear time-series analysis method of phase-space reconstruction, we realized a clustering method according to a comprehensive metric scale of similarity. Then, we determined the partial neighbor information of current points in each time series to predict cyanobacteria blooms in lakes and reservoirs using a local linear regression prediction model. Compared with conventional prediction models and the classical long short-term memory model, the results of the trend consistency and the accuracy demonstrated that the proposed model was superior. This verified the validity of the systematized model construction method. This model can be used to predict cyanobacteria blooms in lakes and reservoirs and supports the treatment of the water environment.
更多查看译文
关键词
Lakes and reservoirs, Cyanobacteria bloom, Key influencing factors, Correlation coefficient, Prediction, Metric scale of similarity
AI 理解论文
溯源树
样例
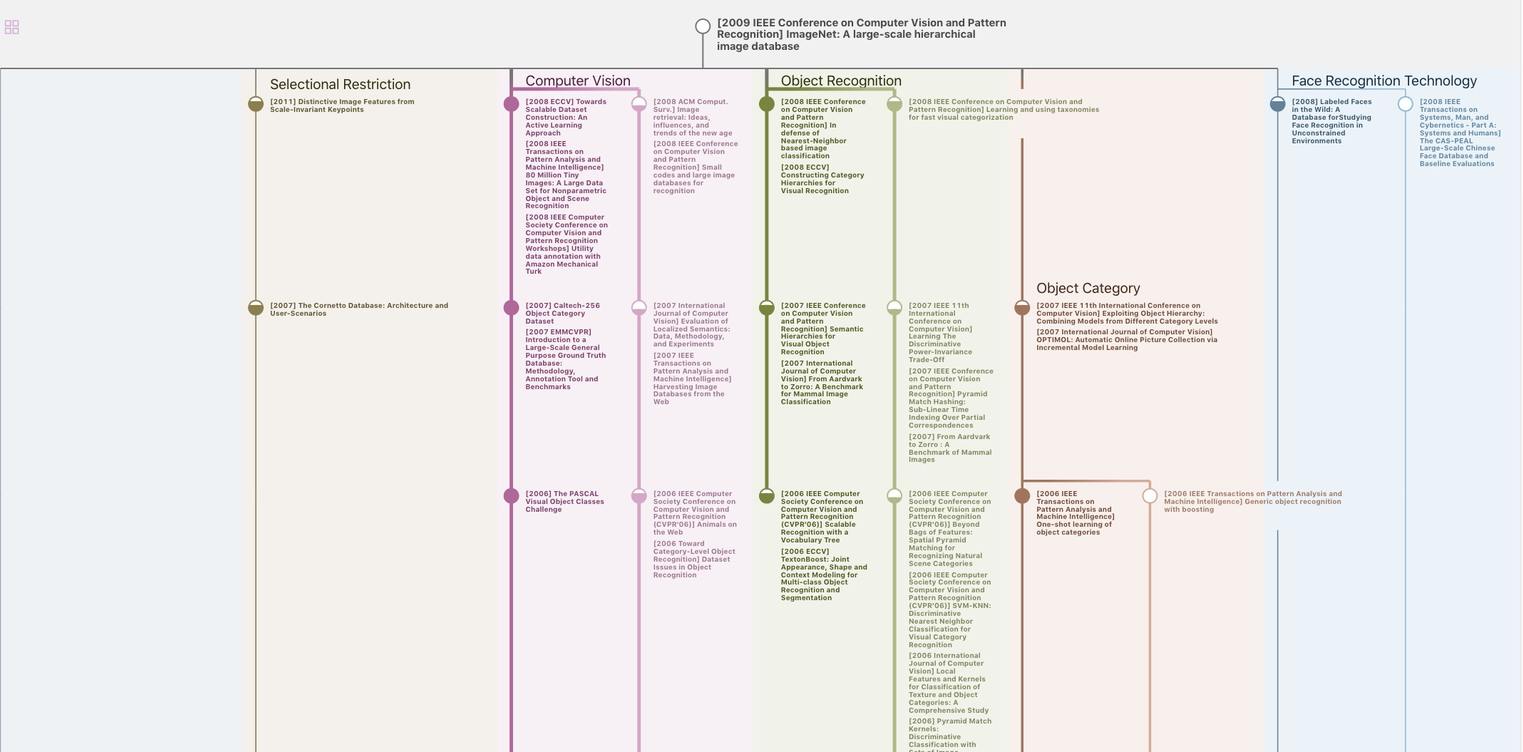
生成溯源树,研究论文发展脉络
Chat Paper
正在生成论文摘要