Double-Step U-Net: A Deep Learning-Based Approach for the Estimation of Wildfire Damage Severity through Sentinel-2 Satellite Data
APPLIED SCIENCES-BASEL(2020)
Abstract
Wildfire damage severity census is a crucial activity for estimating monetary losses and for planning a prompt restoration of the affected areas. It consists in assigning, after a wildfire, a numerical damage/severity level, between 0 and 4, to each sub-area of the hit area. While burned area identification has been automatized by means of machine learning algorithms, the wildfire damage severity census operation is usually still performed manually and requires a significant effort of domain experts through the analysis of imagery and, sometimes, on-site missions. In this paper, we propose a novel supervised learning approach for the automatic estimation of the damage/severity level of the hit areas after the wildfire extinction. Specifically, the proposed approach, leveraging on the combination of a classification algorithm and a regression one, predicts the damage/severity level of the sub-areas of the area under analysis by processing a single post-fire satellite acquisition. Our approach has been validated in five different European countries and on 21 wildfires. It has proved to be robust for the application in several geographical contexts presenting similar geological aspects.
MoreTranslated text
Key words
copernicus,sentinel,satellite,wildfire,severity,U-Net,convolutional neural network,artificial intelligence
AI Read Science
Must-Reading Tree
Example
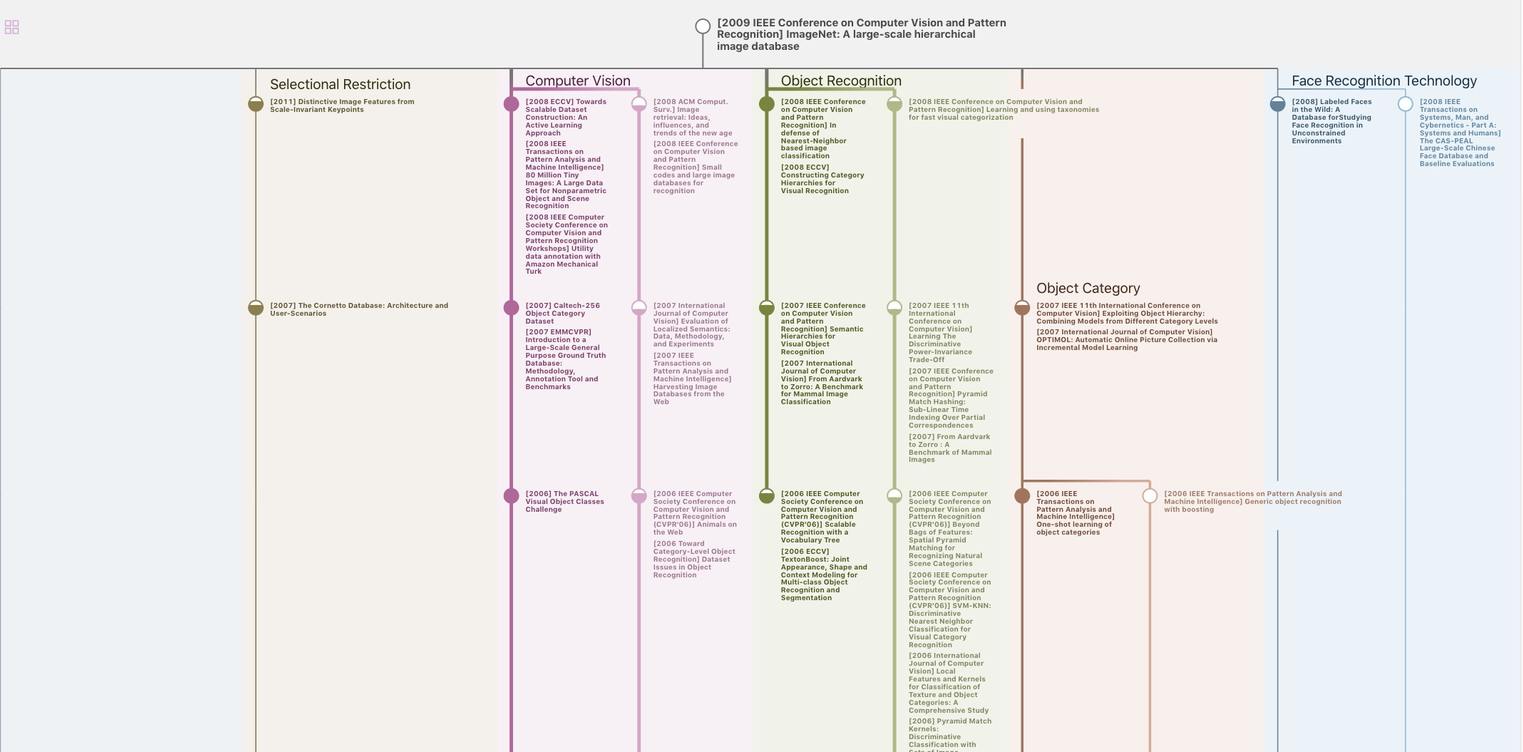
Generate MRT to find the research sequence of this paper
Chat Paper
Summary is being generated by the instructions you defined