Machine learning (ML) predictive algorithm for childhood-onset chronic pruritic dermatoses (CPD) identifies acrylamide and glycidamide as itch modulators
JOURNAL OF INVESTIGATIVE DERMATOLOGY(2020)
摘要
The factors responsible for childhood-onset CPD are not fully known. We hypothesize that environmental factors play a role and ML algorithms can increase understanding of childhood-onset CPD. We employ the classification random forest (RF) algorithm to systematically evaluate the association between CPD and environmental exposures in children (ages 16 and younger) in the 2005-2006 NHANES. We define incident CPD as presence of an itchy rash for at least 6 months with first rash occurrence within the past year. Variables in the analysis include demographics and environmental exposures, as described in NHANES demographics and laboratory data sections. Analyses were conducted using nhanesA and randomForestSRC R packages. We identified 69 individuals aged 16 years or younger with CPD developing within the past year. With random sampling of pediatric controls, the RF training data (80% of overall dataset) included 110 individuals (mean age 7.17 +/- 5.47) with 55 CPD cases and the test data (remaining 20% of dataset) included 28 individuals (mean age 5.50 +/- 5.10) with 14 CPD cases. Using RF for feature selection, we identified the top predictors as acrylamide, glycidamide, and age. Afterwards, we constructed the RF model using only these predictors. The performance of the final RF model is as follows: accuracy: 0.89, sensitivity: 0.86, specificity: 0.93, positive predictive value: 0.92, and negative predictive value: 0.86. Our results demonstrate the ability to predict CPD in the NHANES pediatric population and to identify top predictors of potential clinical importance. Extensions can further increase understanding of how interactions between environmental exposures, genetics, and lifestyle factors contribute to CPD. Overall, our approach has implications for improving prediction and prevention of CPD.
更多查看译文
AI 理解论文
溯源树
样例
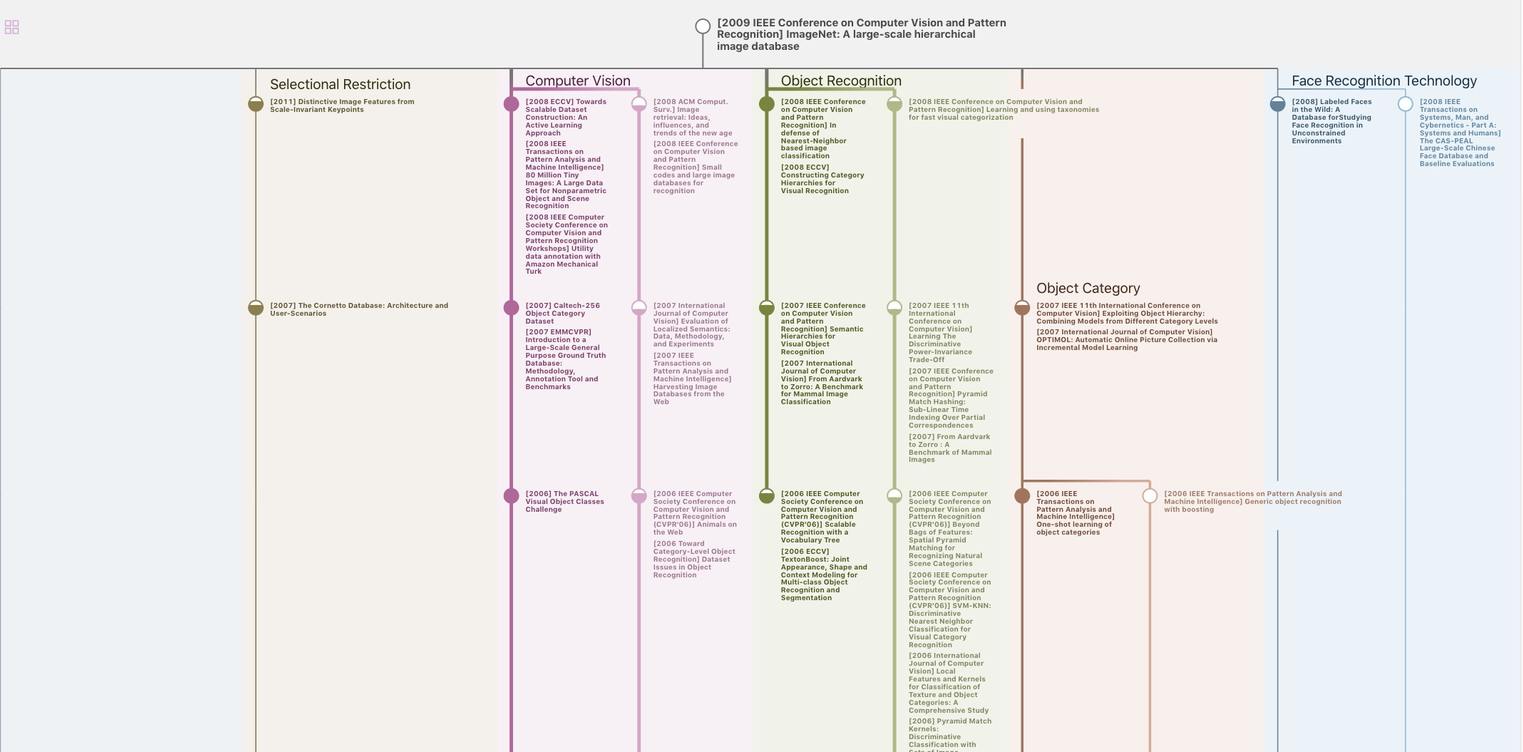
生成溯源树,研究论文发展脉络
Chat Paper
正在生成论文摘要