Randomized Multi-Label Subproblems Concatenation Via Error Correcting Output Codes.
Neurocomputing(2020)
摘要
In this paper, we study the error correcting output codes for multi-label classification problems. Imbalance problem is very common in multi-label task and it has not been effectively solved yet. In previous works, base classifiers are learned from the same pool of training data which may lead to similar classifiers, especially when a large amount of labels exist. To tackle these challenges, we propose a novel model called Randomized Multi-Label Subproblems Concatenation via Error Correcting Output Codes (RMSC). In order to reduce the imbalance problem and enhance the diversity between base classifiers, in our model, the original multi-label problem is transformed into an ensemble of many multi-label sub-problems and the one-vs-all encoding strategy is conducted on these sub-problems and finally corresponding coding matrices are concatenated into a whole coding matrix. Moreover, we provide a proof of enhanced diversity in our model, together with some discussions about the parameter determination and time complexity. Extensive experimental results on various kinds of data sets well demonstrate that the proposed model consistently outperforms the state-of-the-art methods.
更多查看译文
关键词
Multi-label classification,Error correcting output codes,Imbalance problem,Diversity
AI 理解论文
溯源树
样例
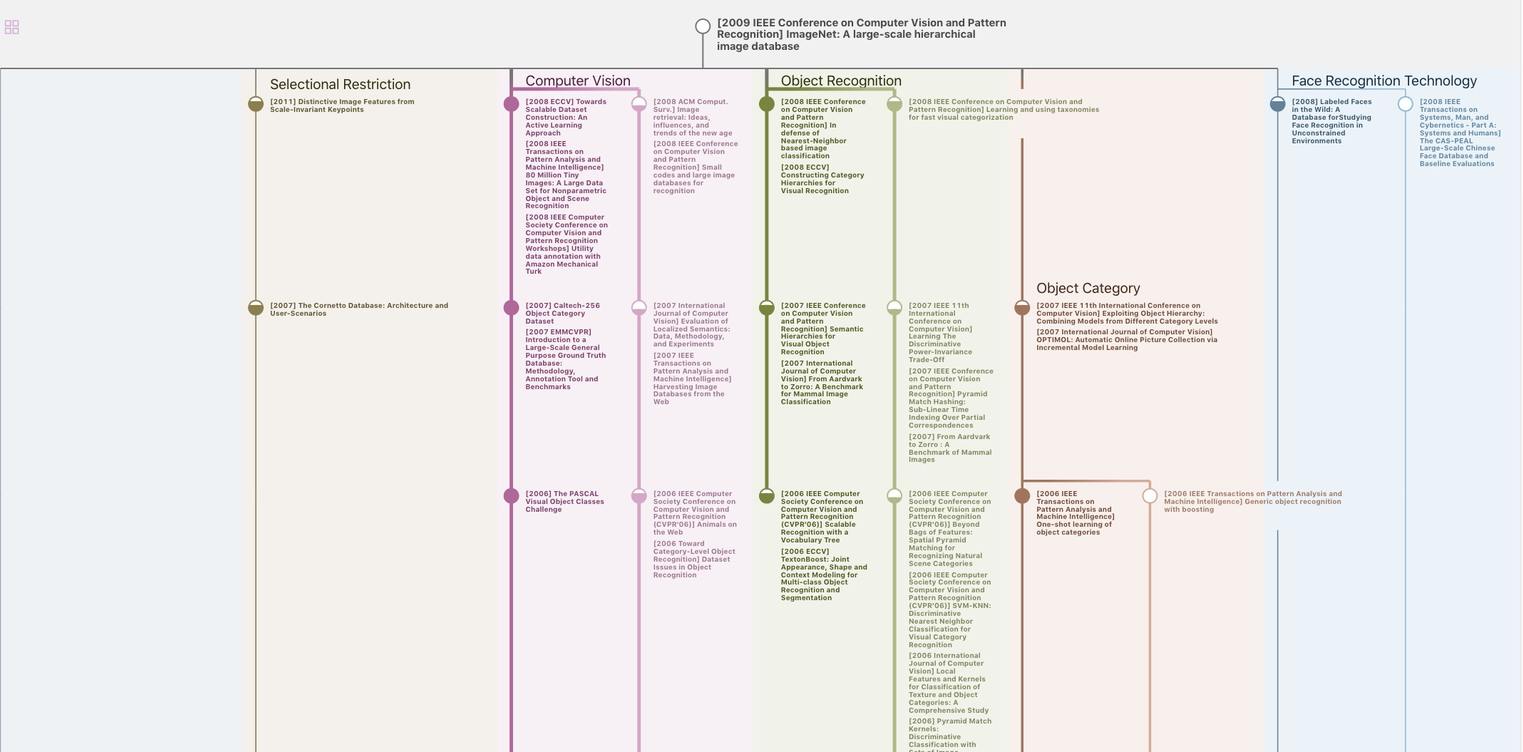
生成溯源树,研究论文发展脉络
Chat Paper
正在生成论文摘要