UAV navigation in high dynamic environments: A deep reinforcement learning approach
Chinese Journal of Aeronautics(2021)
摘要
Unmanned Aerial Vehicle (UAV) navigation is aimed at guiding a UAV to the desired destinations along a collision-free and efficient path without human interventions, and it plays a crucial role in autonomous missions in harsh environments. The recently emerging Deep Reinforcement Learning (DRL) methods have shown promise for addressing the UAV navigation problem, but most of these methods cannot converge due to the massive amounts of interactive data when a UAV is navigating in high dynamic environments, where there are numerous obstacles moving fast. In this work, we propose an improved DRL-based method to tackle these fundamental limitations. To be specific, we develop a distributed DRL framework to decompose the UAV navigation task into two simpler sub-tasks, each of which is solved through the designed Long Short-Term Memory (LSTM) based DRL network by using only part of the interactive data. Furthermore, a clipped DRL loss function is proposed to closely stack the two sub-solutions into one integral for the UAV navigation problem. Extensive simulation results are provided to corroborate the superiority of the proposed method in terms of the convergence and effectiveness compared with those of the state-of-the-art DRL methods.
更多查看译文
关键词
Autonomous vehicles,Deep learning,Motion planning,Navigation,Reinforcement learning,Unmanned Aerial Vehicle (UAV)
AI 理解论文
溯源树
样例
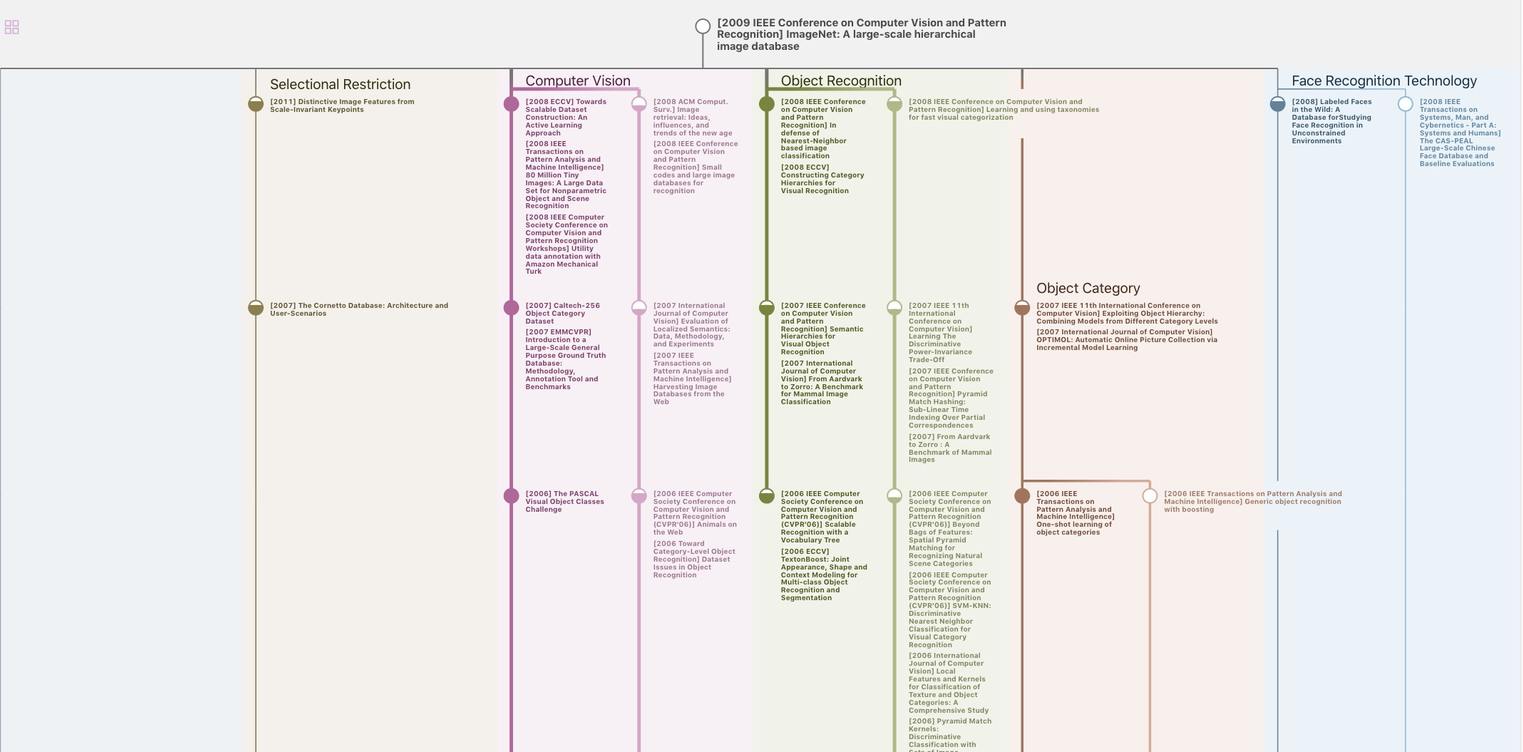
生成溯源树,研究论文发展脉络
Chat Paper
正在生成论文摘要