Random survival forest for competing credit risks
JOURNAL OF THE OPERATIONAL RESEARCH SOCIETY(2022)
摘要
Random survival forest for Competing Risks (CR Rsf) is a tree-based estimation and prediction method. The applications of this recently proposed method have not yet been considered in the extant credit risk literature. The appealing features of CR Rsf compared to the existing competing risks methods are that it is nonparametric and has the ability to handle high-dimensional data. This paper applies CR Rsf to the financial dataset which involves two competing credit risks: default and early repayment. This application yields two novel findings. First, CR Rsf dominates, in terms of prediction accuracy, the state of art model in survival analysis-Cox proportional hazard model for competing risks. Second, ignoring the competing risk event of early repayment results in an upwardly-biased estimate of the cumulative probability of default. The first finding suggests that CR Rsf may be a useful alternative to the existing competing risks models. The second has ramifications for the extant literature devoted to the estimation of the probability of default in cases where a competing risk exists, but is not explicitly taken into account.
更多查看译文
关键词
Machine learning, brier score, competing credit risks, cox hazard model, cumulative incidence function of default
AI 理解论文
溯源树
样例
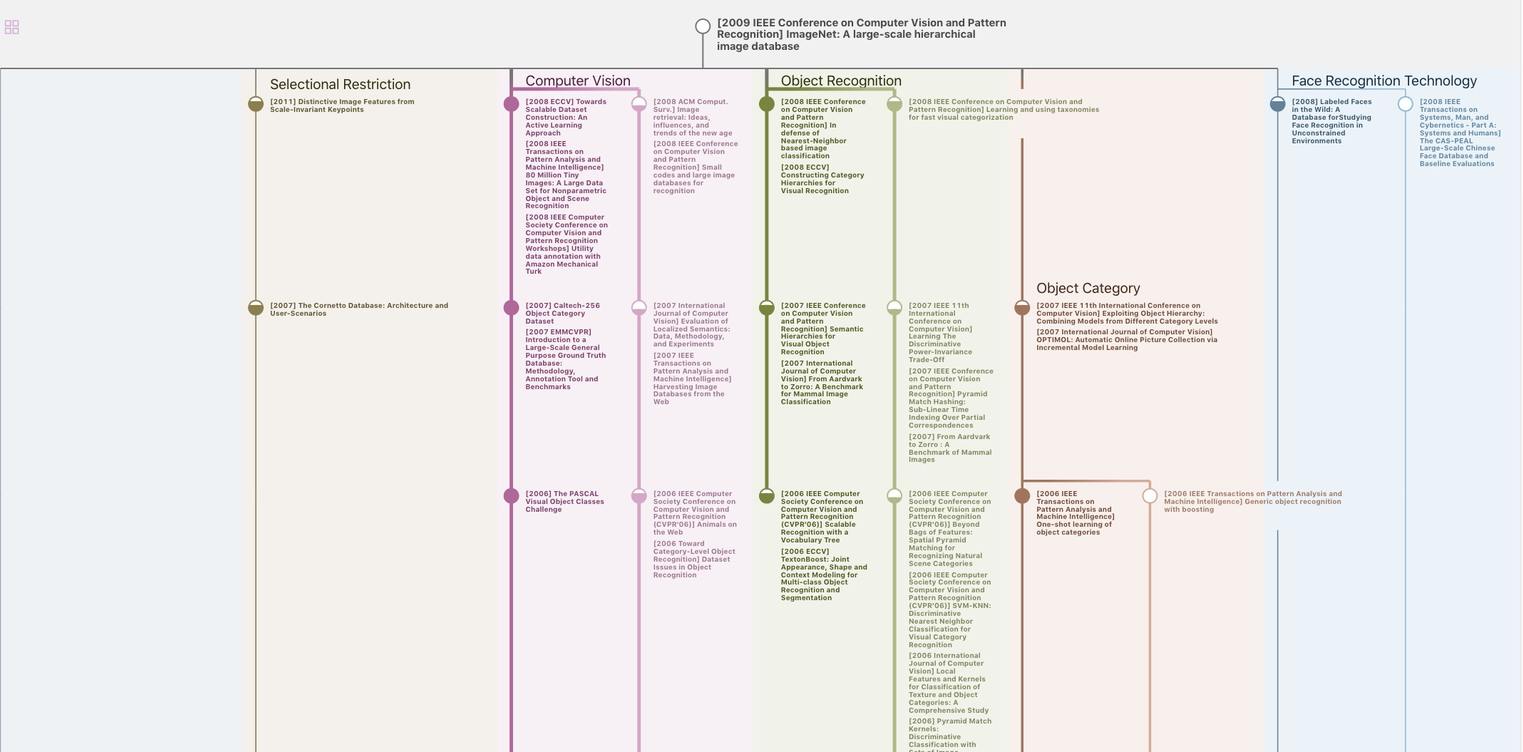
生成溯源树,研究论文发展脉络
Chat Paper
正在生成论文摘要