Utilizing Automatic Query Reformulations as Genetic Operations to Improve Feature Location in Software Models
IEEE Transactions on Software Engineering(2022)
摘要
In the combination of Model-Driven Engineering (MDE) and Search-Based Software Engineering (SBSE), genetic operations are one of the key ingredients. Our work proposes a novel adaptation of automatic query reformulations as genetic operations that leverage the latent semantics of software models (the cornerstone artefact of MDE). We analyze the impact of these reformulation operations in a real-world industrial case study of feature location in models. As baselines, we use: 1) the widespread single-point crossover plus random mutation; and 2) mask crossover plus random mutation, which is the best performer for feature location in models. We also perform a statistical analysis to provide quantitative evidence of the impact of the results and to show that this impact is significant. Our reformulation operations improve the results of the best baseline by 37.73 percent in recall and 14.08 percent in precision. These results are relevant for the task of feature location in models (one of the main activities performed during software maintenance and evolution). Furthermore, given that the only requirement to apply our approach is term availability in models, our work opens a new research direction to improve more tasks in MDE such as bug location or requirements traceability.
更多查看译文
关键词
Model-driven engineering,search-based software engineering,automatic query reformulations
AI 理解论文
溯源树
样例
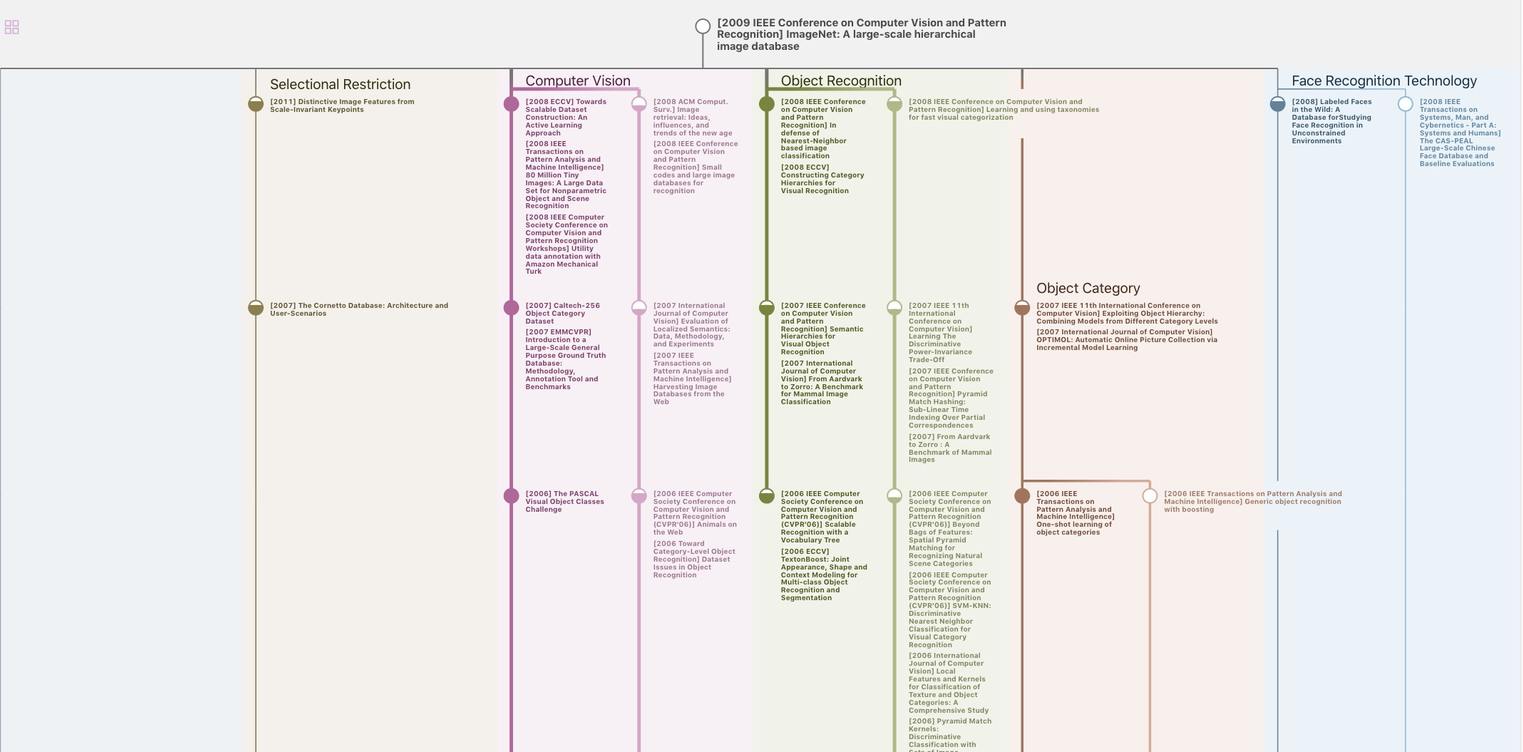
生成溯源树,研究论文发展脉络
Chat Paper
正在生成论文摘要