Large Scale Network Embedding: A Separable Approach
IEEE Transactions on Knowledge and Data Engineering(2022)
摘要
Many successful methods have been proposed for learning low-dimensional representations on large-scale networks, while almost all existing methods are designed in inseparable processes, learning embeddings for entire networks even when only a small proportion of nodes are of interest. This leads to great inconvenience, especially on large-scale or dynamic networks, where these methods become almost impossible to implement. In this paper, we formalize the problem of separated matrix factorization, based on which we elaborate a novel objective function that preserves both local and global information. We compare our SMF framework with approximate SVD algorithms and demonstrate SMF can capture more information when factorizing a given matrix. We further propose SepNE, a simple and flexible network embedding algorithm which independently learns representations for different subsets of nodes in separated processes. By implementing separability, our algorithm reduces the redundant efforts to embed irrelevant nodes, yielding scalability to large networks. To further incorporate complex information into SepNE, we discuss several methods that can be used to leverage high-order proximities in large networks. We demonstrate the effectiveness of SepNE on several real-world networks with different scales and subjects. With comparable accuracy, our approach significantly outperforms state-of-the-art baselines in running times on large networks.
更多查看译文
关键词
Network embedding,matrix factorization,separability
AI 理解论文
溯源树
样例
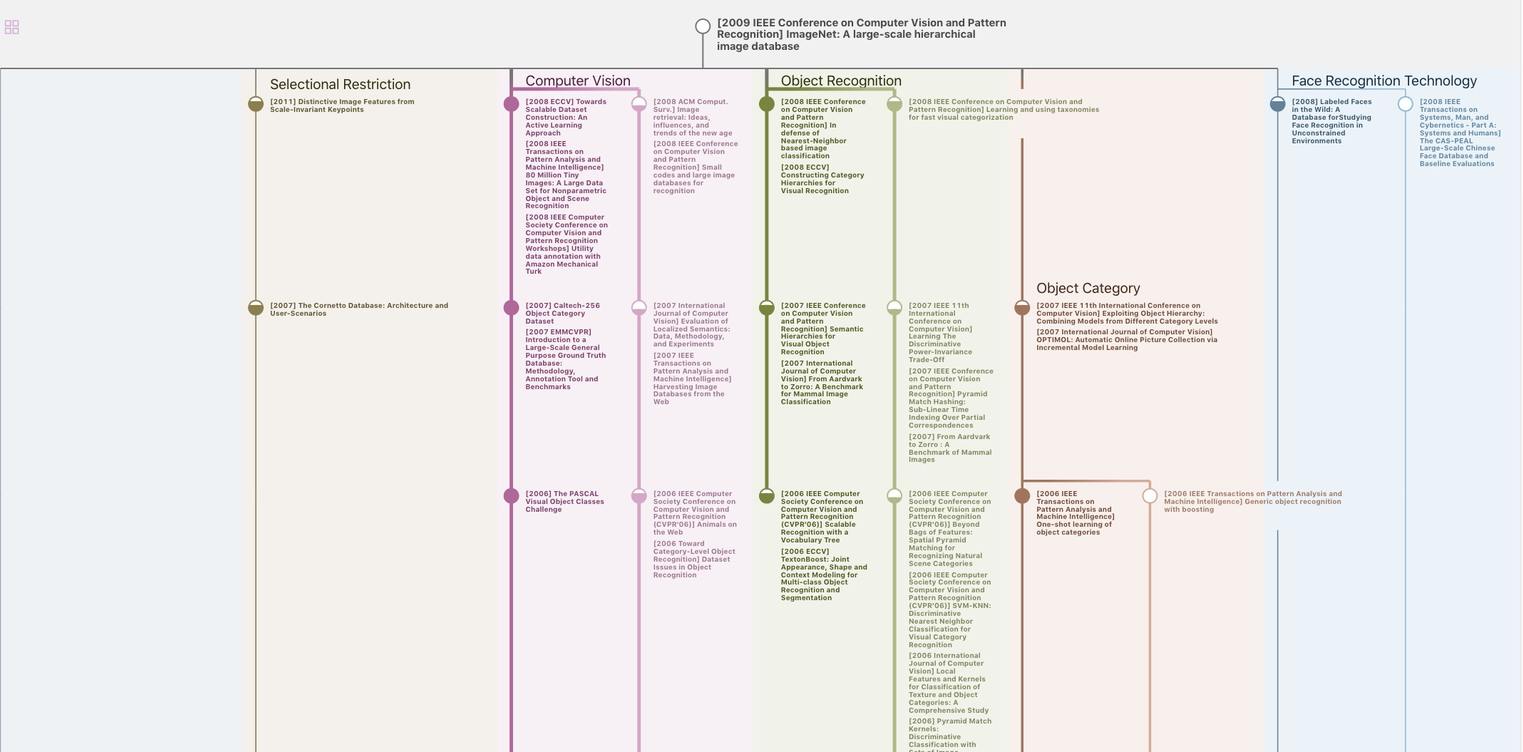
生成溯源树,研究论文发展脉络
Chat Paper
正在生成论文摘要