DIAGNOSIS OF LUNG NODULES FROM 2D COMPUTER TOMOGRAPHY SCANS
BIOMEDICAL ENGINEERING-APPLICATIONS BASIS COMMUNICATIONS(2020)
摘要
Cancers typically are both highly dangerous and common. Among these, lung cancer has one of the lowest survival rates compared to other cancers. CT scans can reveal dense masses of different shapes and sizes; in the lungs, these are called lung nodules. This study applied a computer-aided diagnosis (CAD) system to detect candidate nodules - and diagnose it either solitary or juxtapleural - with equivalent diameters, ranging from 7.78 mm to 22.48 mm in a 2D CT slice. Pre-processing and segmentation is a very important step to segment and enhance the CT image. A segmentation and enhancement algorithm is achieved using bilateral filtering, Thresholding the gray-level transformation function, Bounding box and maximum intensity projection. Border artifacts are removed by clearing the lung border, erosion, dilation and superimposing. Feature extraction is done by extracting 20 gray-level co-occurrence matrix features from four directions: 0 degrees, 45 degrees, 90 degrees and 135 degrees and one distance of separation (d = 1 pixel). In the classification step, two classifiers are proposed to classify two types of nodules based on their locations: as juxtapleural or solitary nodules. The two classifiers are a deep learning convolutional neural network (CNN) and the K-nearest neighbor (KNN) algorithm. Random oversampling and 10-fold cross-validation are used to improve the results. In our CAD system, the highest accuracy and sensitivity rates achieved by the CNN were 96% and 95%, respectively, for solitary nodule detection. The highest accuracy and sensitivity rates achieved by the KNN model were 93.8% and 96.7%, respectively, and K was set to 1 to detect juxtapleural nodules.
更多查看译文
关键词
CT scans,Maximum intensity projection,Convolutional neural network,Deep learning,K-nearest neighbor
AI 理解论文
溯源树
样例
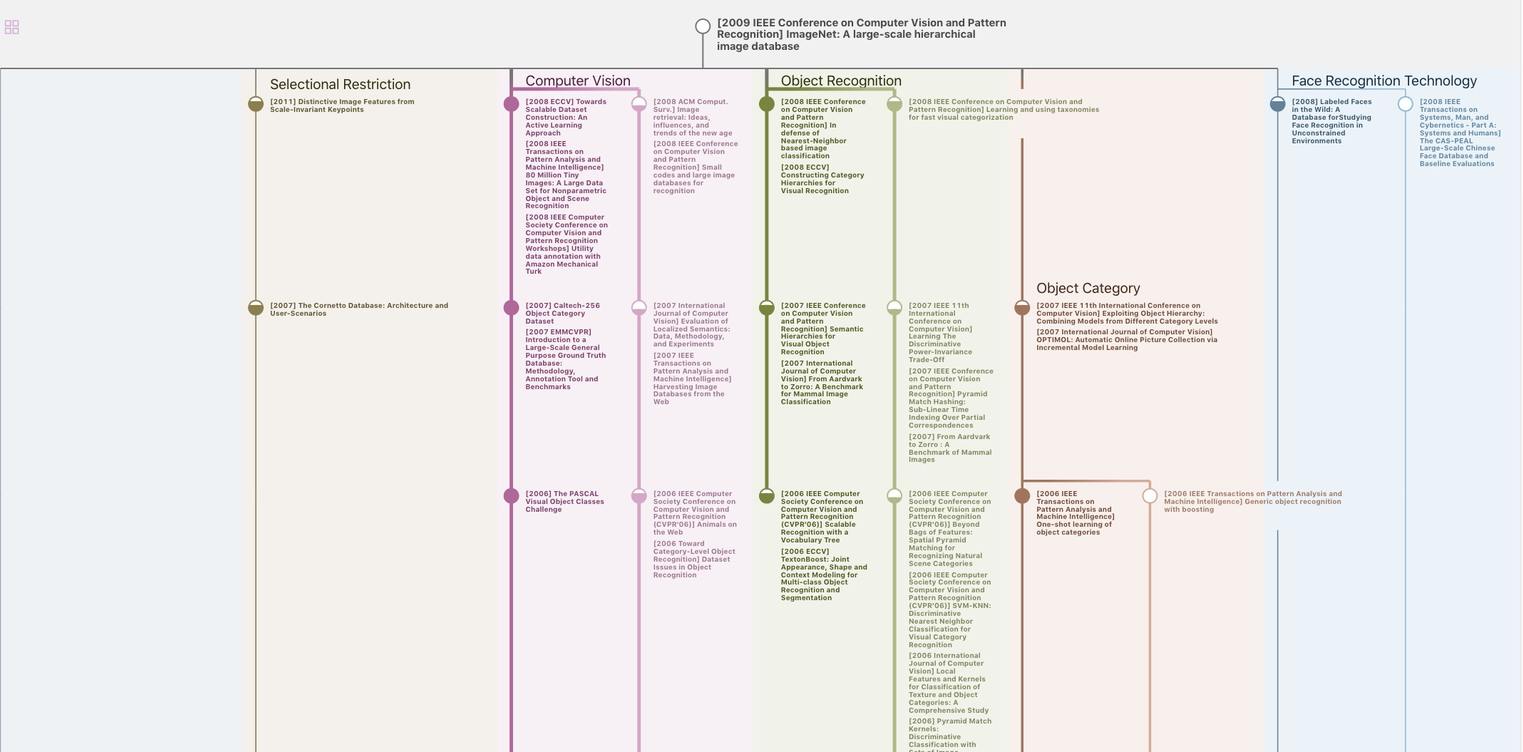
生成溯源树,研究论文发展脉络
Chat Paper
正在生成论文摘要