Gmm With Parameters Initialization Based On Svd For Network Threat Detection
JOURNAL OF INTELLIGENT & FUZZY SYSTEMS(2021)
摘要
In the field of security, the data labels are unknown or the labels are too expensive to label, so that clustering methods are used to detect the threat behavior contained in the big data. The most widely used probabilistic clustering model is Gaussian Mixture Models(GMM), which is flexible and powerful to apply prior knowledge for modelling the uncertainty of the data. Therefore, in this paper, we use GMM to build the threat behavior detection model. Commonly, Expectation Maximization (EM) and Variational Inference (VI) are used to estimate the optimal parameters of GMM. However, both EM and VI are quite sensitive to the initial values of the parameters. Therefore, we propose to use Singular Value Decomposition (SVD) to initialize the parameters. Firstly, SVD is used to factorize the data set matrix to get the singular value matrix and singular matrices. Then we calculate the number of the components of GMM by the first two singular values in the singular value matrix and the dimension of the data. Next, other parameters of GMM, such as the mixing coefficients, the mean and the covariance, are calculated based on the number of the components. After that, the initialization values of the parameters are input into EM and VI to estimate the optimal parameters of GMM. The experiment results indicate that our proposed method performs well on the parameters initialization of GMM clustering using EM and VI for estimating parameters.
更多查看译文
关键词
Network threat detection, gaussian mixture models, expectation maximization, variational inference, singular value decomposition, parameters initialization
AI 理解论文
溯源树
样例
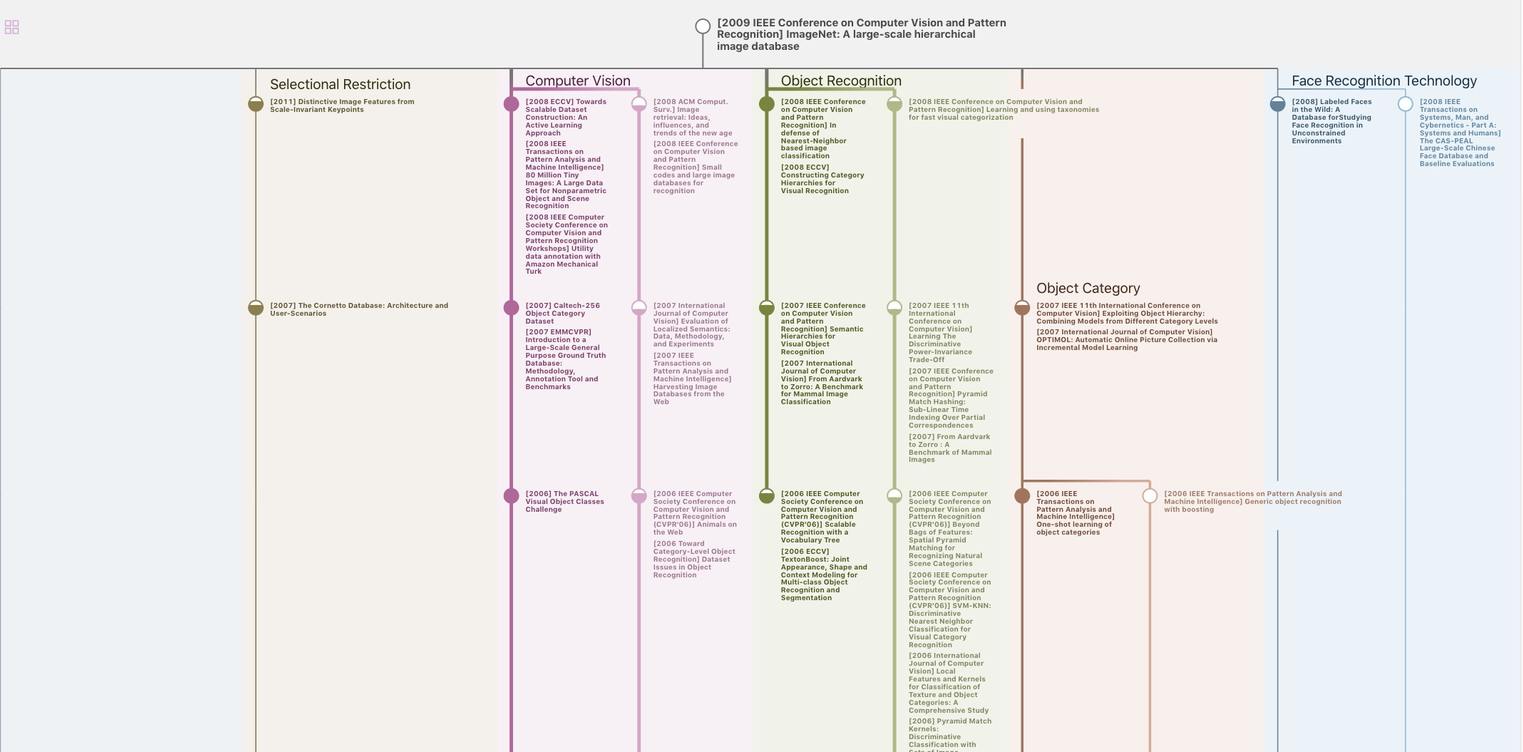
生成溯源树,研究论文发展脉络
Chat Paper
正在生成论文摘要