Sentiment analysis of tweets through Altmetrics: A machine learning approach
JOURNAL OF INFORMATION SCIENCE(2021)
摘要
The purpose of the study is to (a) contribute to annotating an Altmetrics dataset across five disciplines, (b) undertake sentiment analysis using various machine learning and natural language processing-based algorithms, (c) identify the best-performing model and (d) provide a Python library for sentiment analysis of an Altmetrics dataset. First, the researchers gave a set of guidelines to two human annotators familiar with the task of related tweet annotation of scientific literature. They duly labelled the sentiments, achieving an inter-annotator agreement (IAA) of 0.80 (Cohen's Kappa). Then, the same experiments were run on two versions of the dataset: one with tweets in English and the other with tweets in 23 languages, including English. Using 6388 tweets about 300 papers indexed in Web of Science, the effectiveness of employed machine learning and natural language processing models was measured by comparing with well-known sentiment analysis models, that is, SentiStrength and Sentiment140, as the baseline. It was proved that Support Vector Machine with uni-gram outperformed all the other classifiers and baseline methods employed, with an accuracy of over 85%, followed by Logistic Regression at 83% accuracy and Naive Bayes at 80%. The precision, recall and F1 scores for Support Vector Machine, Logistic Regression and Naive Bayes were (0.89, 0.86, 0.86), (0.86, 0.83, 0.80) and (0.85, 0.81, 0.76), respectively.
更多查看译文
关键词
Altmetrics, comparative analysis, machine learning, sentiment analysis, Twitter
AI 理解论文
溯源树
样例
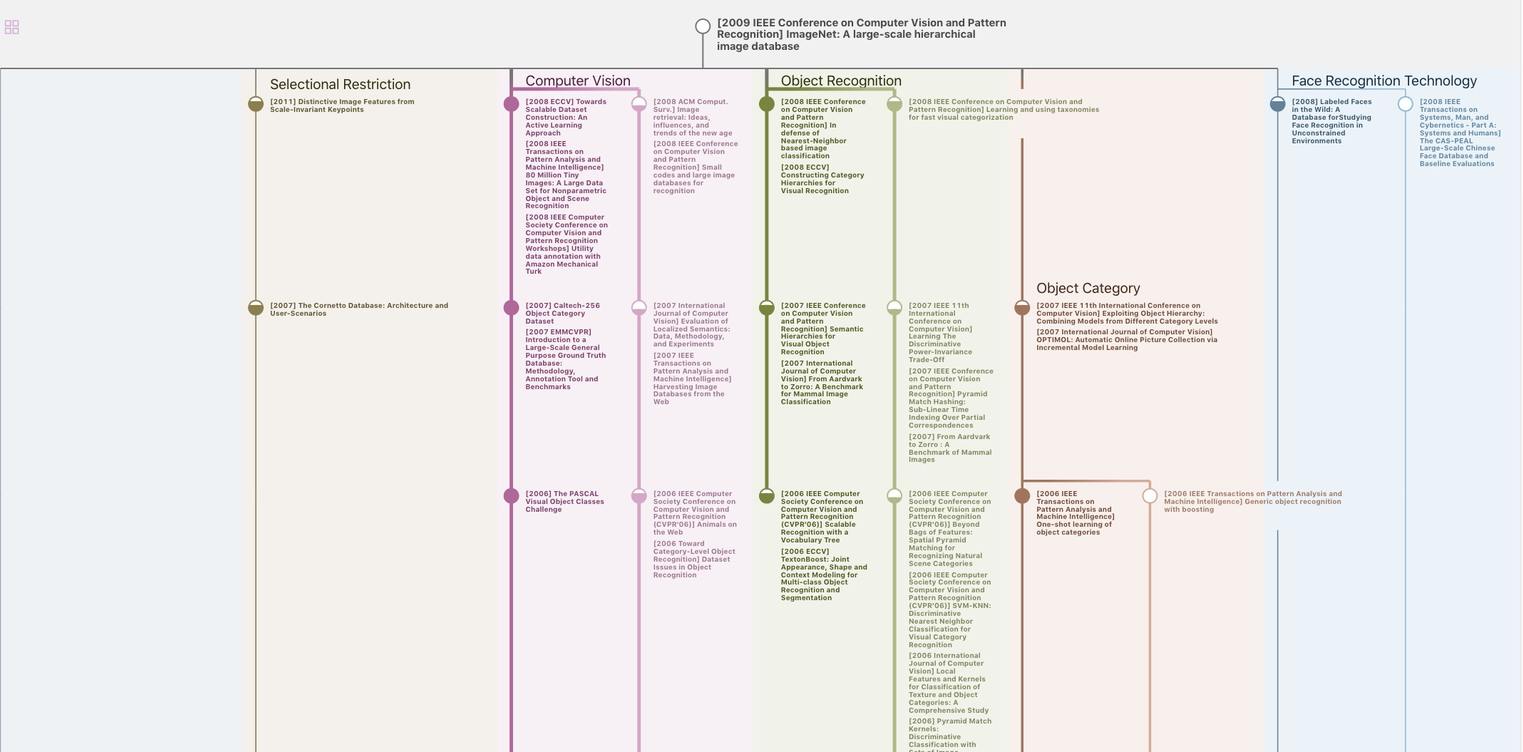
生成溯源树,研究论文发展脉络
Chat Paper
正在生成论文摘要