Hierarchical graph attention networks for semi-supervised node classification
APPLIED INTELLIGENCE(2020)
摘要
Recently, there has been a promising tendency to generalize convolutional neural networks (CNNs) to graph domain. However, most of the methods cannot obtain adequate global information due to their shallow structures. In this paper, we address this challenge by proposing a hierarchical graph attention network (HGAT) for semi-supervised node classification. This network employs a hierarchical mechanism for the learning of node features. Thus, more information can be effectively obtained of the node features by iteratively using coarsening and refining operations on different hierarchical levels. Moreover, HGAT combines with the attention mechanism in the input and prediction layer. It can assign different weights to different nodes in a neighborhood, which helps to improve accuracy. Experiment results demonstrate that state-of-the-art performance was achieved by our method, not only on Cora, Citeseer, and Pubmed citation datasets, but also on the simplified NELL knowledge graph dataset. The sensitive analysis further verifies that HGAT can capture global structure information by increasing the receptive field, as well as the effective transfer of node features.
更多查看译文
关键词
Graph convolutional networks,Hierarchical representation,Semi-supervisded
AI 理解论文
溯源树
样例
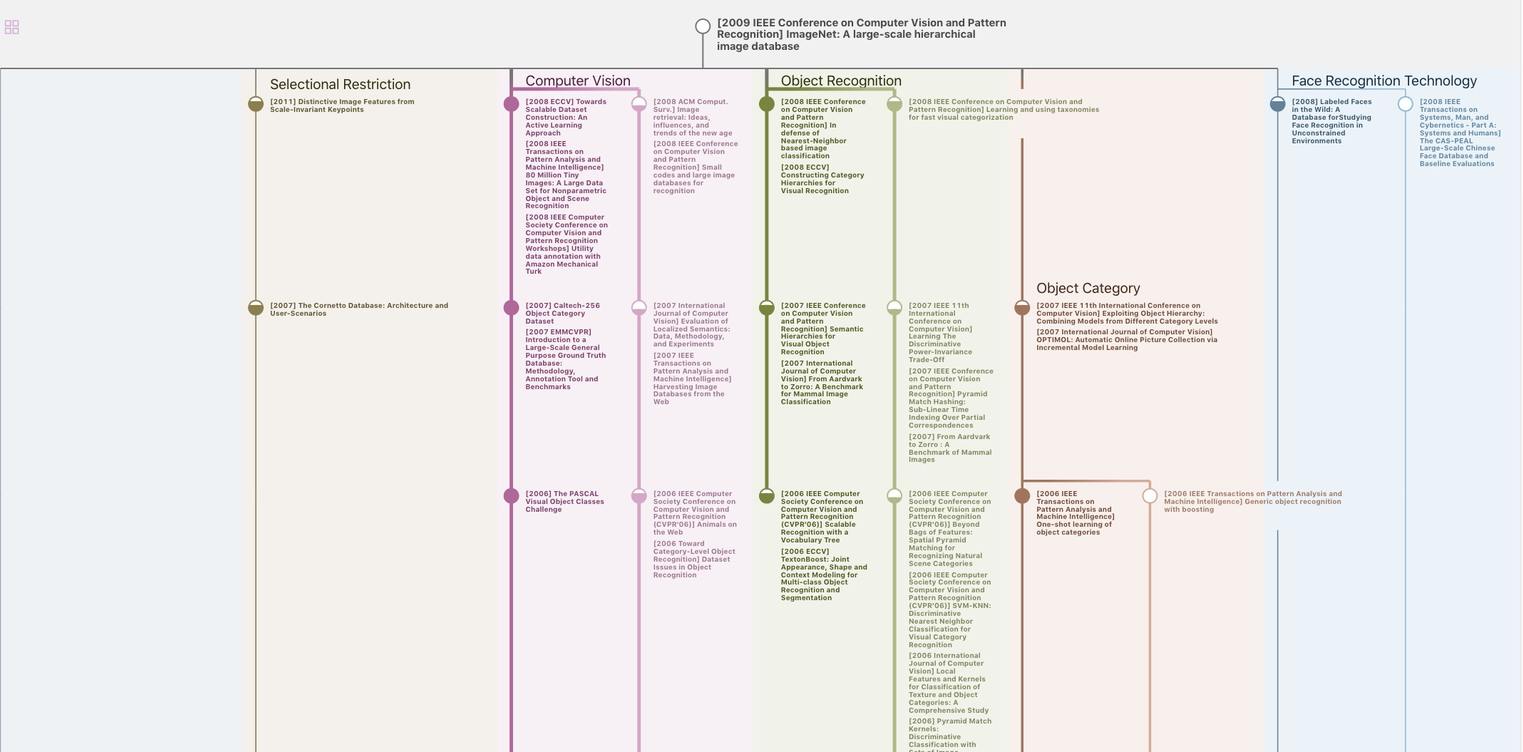
生成溯源树,研究论文发展脉络
Chat Paper
正在生成论文摘要