Argument-based assessment of predictive uncertainty of data-driven environmental models
Environmental Modelling & Software(2020)
摘要
Increasing volumes of data allow environmental scientists to use machine learning to construct data-driven models of phenomena. These models can provide decision-relevant predictions, but confident decision-making requires that the involved uncertainties are understood. We argue that existing frameworks for characterizing uncertainties are not appropriate for data-driven models because of their focus on distinct locations of uncertainty. We propose a framework for uncertainty assessment that uses argument analysis to assess the justification of the assumption that the model is fit for the predictive purpose at hand. Its flexibility makes the framework applicable to data-driven models. The framework is illustrated using a case study from environmental science. We show that data-driven models can be subject to substantial second-order uncertainty, i.e., uncertainty in the assessment of the predictive uncertainty, because they are often applied to ill-understood problems. We close by discussing the implications of the predictive uncertainties of data-driven models for decision-making.
更多查看译文
关键词
Uncertainty,Data-driven models,Argument analysis,Predictions,Decision-making
AI 理解论文
溯源树
样例
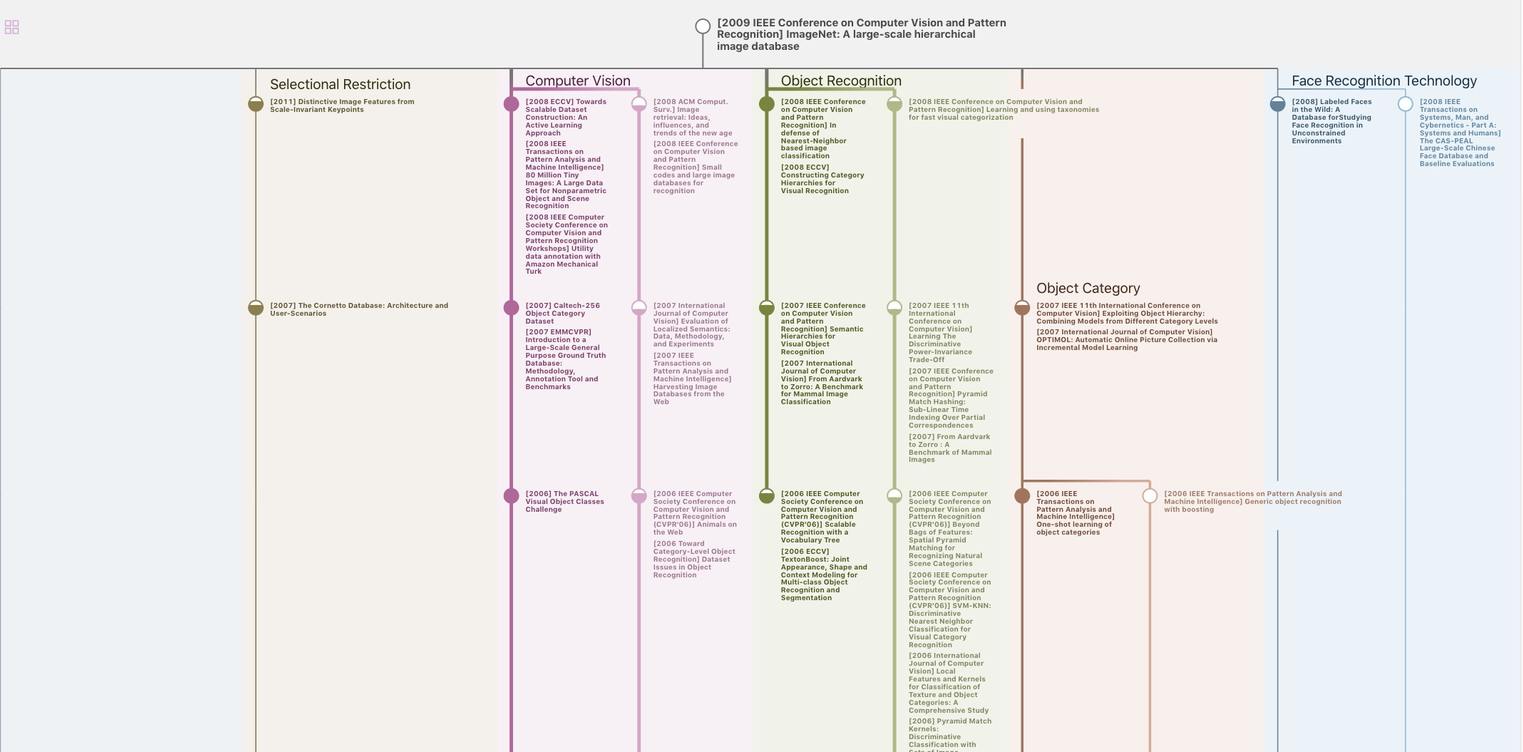
生成溯源树,研究论文发展脉络
Chat Paper
正在生成论文摘要