A Semi-supervised Trace Lasso Norm Regularized Image Classification Method
TWELFTH INTERNATIONAL CONFERENCE ON DIGITAL IMAGE PROCESSING (ICDIP 2020)(2020)
摘要
Since the cost of labeling data is getting higher and higher, it is essential to develop some algorithms for practical applications especially when only a small number of labeled images are available. We deal with this by designing a novel model and show that with a small amount of unlabeled samples added to the training set the classification result can be effectively promoted. In our method the query image is collaboratively represented by the whole training samples, whether they are labeled or unlabeled. There are two regularization terms. Rather than getting the sample sparsity, the first regularization term, a variant Trace Lasso norm, forces the group sparsity, which means the query image is presented by a small number of groups, say one or two groups, and in each group the training samples are fully used. This sparsity between groups and density within a group is preferred to the aim of face classification. The similarity matrix is used in the second term to obtain the pseudo-labels for the unlabeled training samples, thus the whole samples are classified into different groups, each one corresponding to a class. Augmented Lagrange Multiplier scheme and Alternating Direction Method of Multiplier are given to solve the problem numerically. Some experiments are conducted to show the performance of the proposed work.
更多查看译文
关键词
Image classification,trace lasso,semi-supervised
AI 理解论文
溯源树
样例
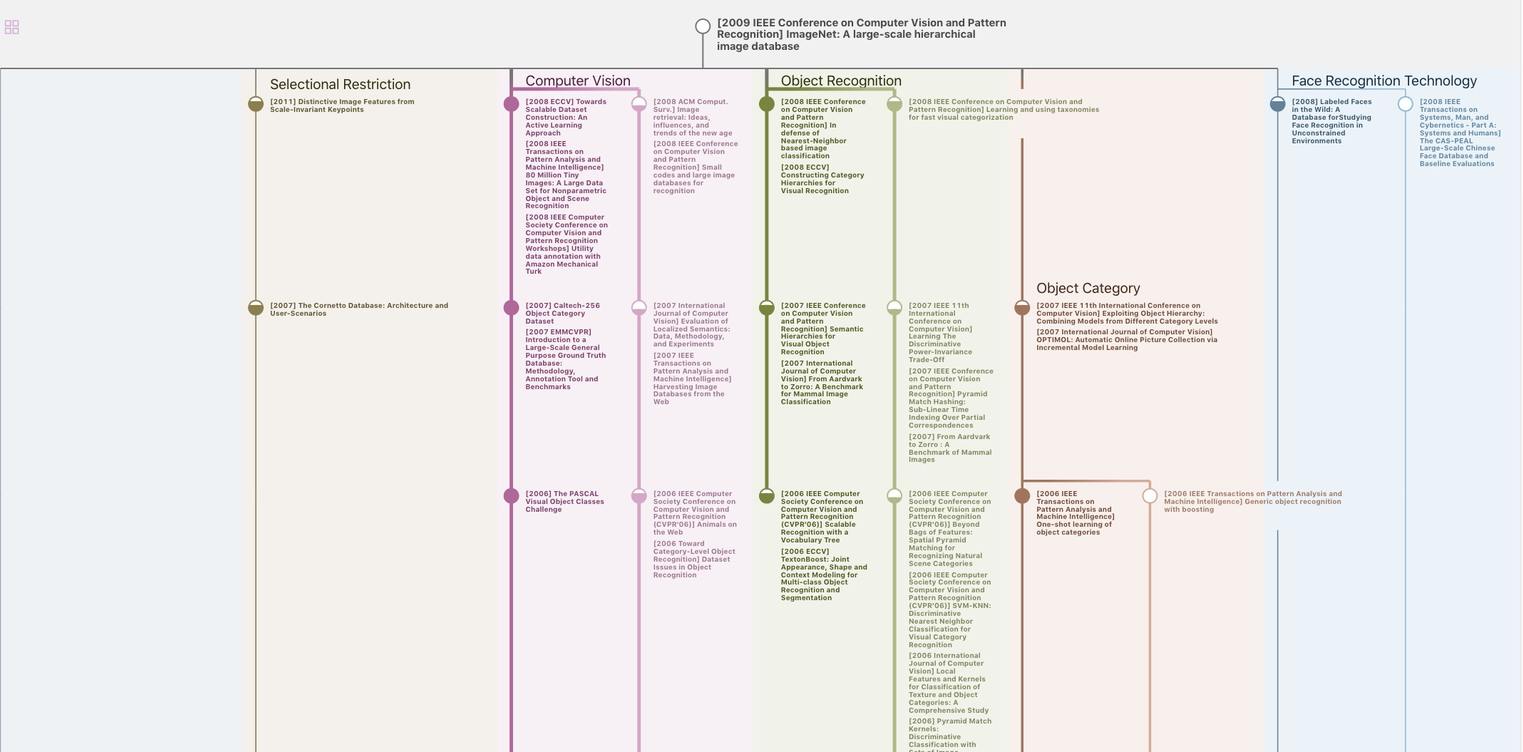
生成溯源树,研究论文发展脉络
Chat Paper
正在生成论文摘要