Multi-component transfer metric learning for handling unrelated source domain samples
Knowledge-Based Systems(2020)
摘要
Transfer learning (TL) is a machine learning paradigm designed for the problem where the training and test data are from different domains. Existing TL approaches mostly assume that training data from the source domain are collected from multiple views or devices. However, in practical applications, a sample in a target domain often only corresponds to a specific view or device. Without the ability to mitigate the influence of the many unrelated samples, the performance of existing TL approaches may deteriorate for such learning tasks. This problem will be exacerbated if the intrinsic relationships among the source domain samples are unclear. Currently, there is no mechanism for determining the intrinsic characteristics of samples in order to treat them differently during TL. The source domain samples that are not related to the test data not only incur computational overhead, but may result in negative transfer. We propose the multi-component transfer metric learning (MCTML) method to address this challenging research problem. Unlike previous metric-based transfer learning which are only capable of using one metric to transform all the samples, MCTML automatically extracts distinct components from the source domain and learns one metric for each component. For each component, MCTML learns the importance of that component in terms of its predictive power based on the Mahalanobis distance metrics. The optimized combination of components are then used to predict the test data collaboratively. Extensive experiments on public datasets demonstrates its effectiveness in knowledge transfer under this challenging condition.
更多查看译文
关键词
Transfer learning,Metric learning,Component,Mahalanobis distance,Weight matrix
AI 理解论文
溯源树
样例
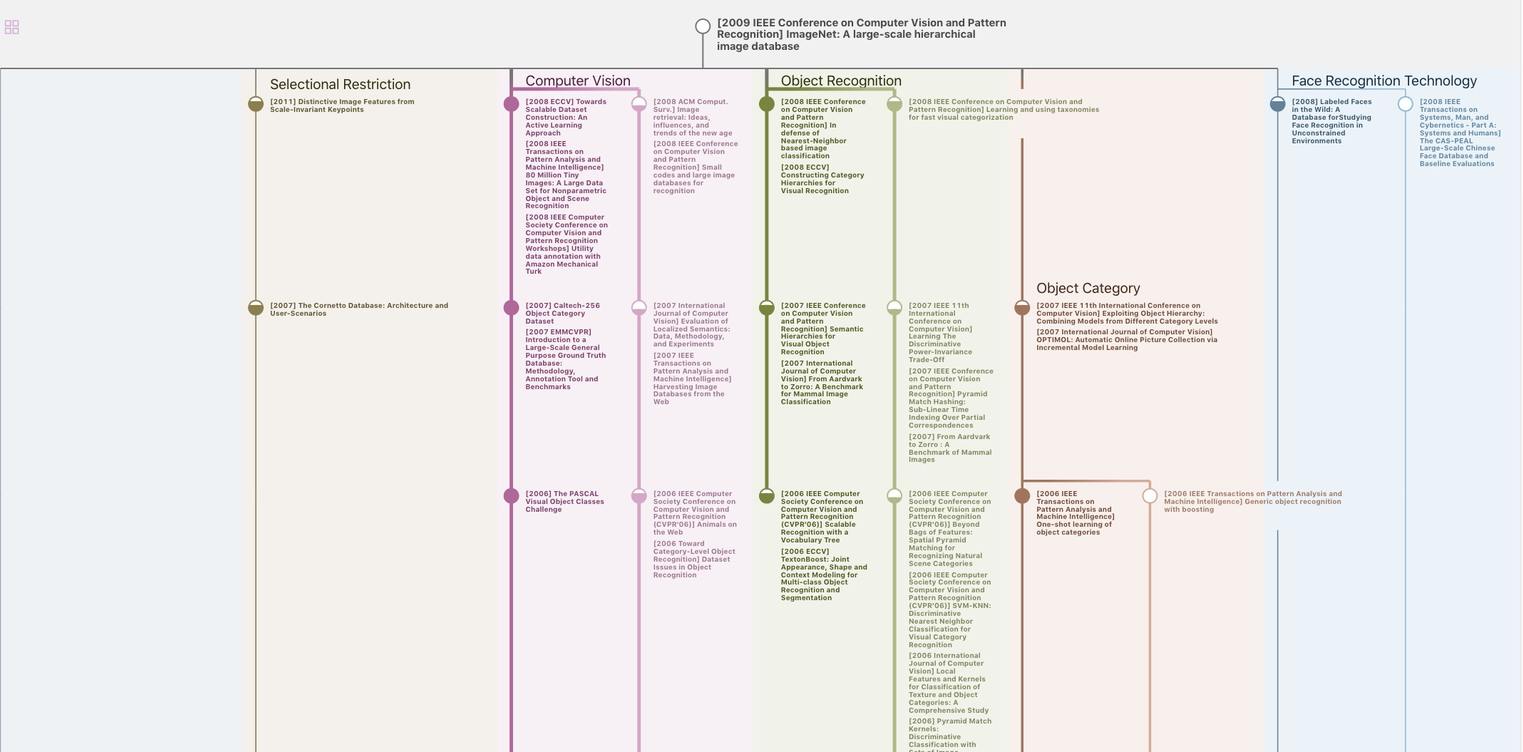
生成溯源树,研究论文发展脉络
Chat Paper
正在生成论文摘要