Clustering Analysis as a Tool to Define Endotypes in Type 1 Diabetes (T1D)
Diabetes(2020)
Abstract
Due to heterogeneity in pediatric T1D, uniform treatment strategies are unlikely to benefit all patients equally. T1D endotypes, or subgroups with distinct characteristics amenable to particular interventions, are a potential solution, but defining endotypes remains a challenge. We used electronic medical records (EMR) to define clusters of distinct presentations of pediatric T1D in a diverse population with a high prevalence of obesity. We analyzed data collected through an EMR Population Health Diabetes Registry of 604 children newly diagnosed with T1D at a large academic hospital in southwestern USA. Using PROC Cluster (SAS 9.4), we applied the Centroid Clustering method to find the optimal number of clusters that minimized the sum of squared distance, then used SAS PROC Tree to identify subjects in each cluster. Variables used were age, DKA at diagnosis, weight, sex, race/ethnicity, c-peptide, hemoglobin A1c (A1c), thyroglobulin antibody (TgAb), and thyroid peroxidase antibody (TPO). Due to multiple testing, p-values Disclosure A.F. Siller: None. X. Gu: None. M. Tosur: None. M. Astudillo: None. A. Balasubramanyam: None. M. Bondy: None. M.J. Redondo: None. Funding Baylor College of Medicine
MoreTranslated text
Key words
diabetes,endotypes,clustering
AI Read Science
Must-Reading Tree
Example
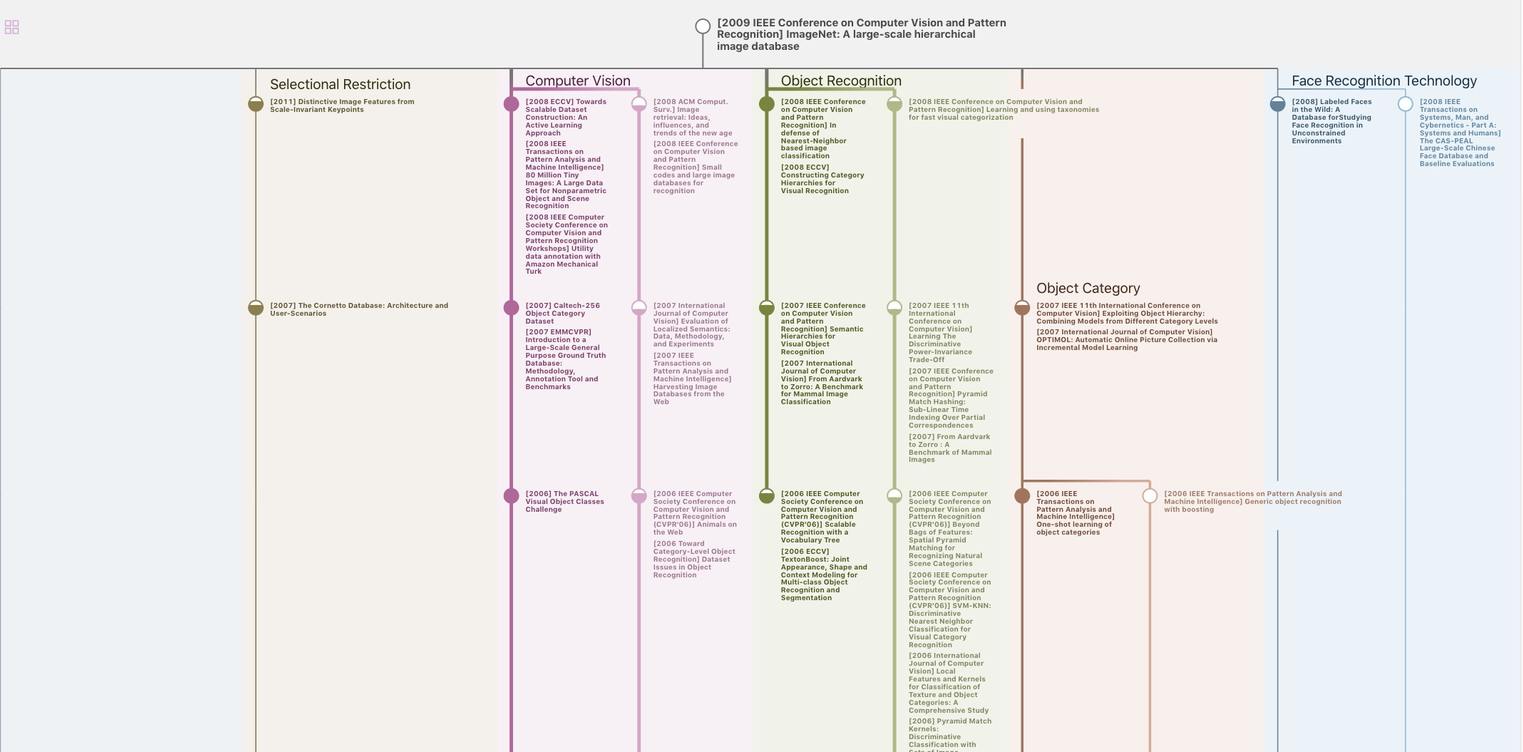
Generate MRT to find the research sequence of this paper
Chat Paper
Summary is being generated by the instructions you defined