Juniper: A Jnd-Based Perceptual Video Coding Framework to Jointly Utilize Saliency and JND
2020 IEEE International Conference on Multimedia & Expo Workshops (ICMEW)(2020)
摘要
The characteristics of human perception are not fully exploited by the conventional quantization control methods. Just Noticeable Distortion (JND)-methods facilitate this by finding perceivable distortion levels. However, these methods fail to use human attention to further reduce bitrate. To address this issue, this paper proposes a Just Noticeable distortion-based Perceptual (JUNIPER) framework for video coding, which combines saliency and JND concepts. Firstly, a JND predictor is trained using Support Vector Machines (SVM) and various quality metrics. Secondly, an efficient saliency model is used to measure the visual importance of each Coding Tree Unit (CTU) in HEVC. Finally, a quantization control algorithm is proposed that jointly considers the visual importance, and the perceivable quality levels, to assign a quantization parameter (QP) to each CTU. Experimental results demonstrate that JUNIPER saves 29.57% bitrate on average, with similar visual quality, compared with baseline HEVC coding.
更多查看译文
关键词
Perceptual video coding (PVC),just noticeable distortion (JND),SVM,saliency map,perceptual quantization control method
AI 理解论文
溯源树
样例
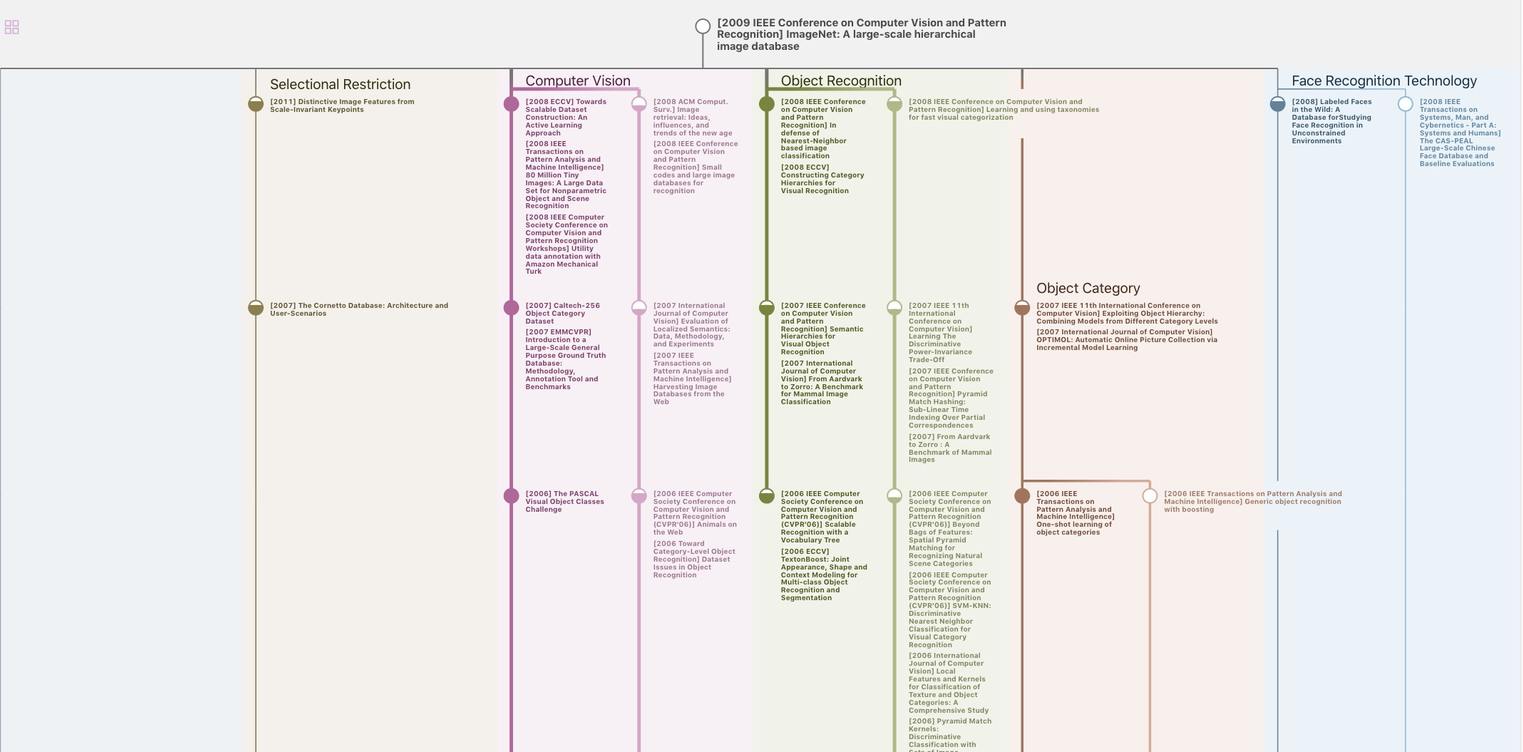
生成溯源树,研究论文发展脉络
Chat Paper
正在生成论文摘要