Inferring Plasma Flows at Granular and Supergranular Scales With a New Architecture for the DeepVel Neural Network
FRONTIERS IN ASTRONOMY AND SPACE SCIENCES(2020)
摘要
The wealth of observational data available has been instrumental in investigating physical features relevant to solar granulation, supergranulation and Active Regions. Meanwhile, numerical models have attempted to bridge the gap between the physics of the solar interior and such observations. However, there are relevant physical quantities that can be modeled but that cannot be directly measured and must be inferred. For example, direct measurements of plasma motions at the photosphere are limited to the line-of-sight component. Methods have consequently been developed to infer the transverse plasma motions from continuum images in the case of the Quiet Sun and magnetograms in the case of Active Regions. Correlation-based tracking methods calculate the optical flows by correlating series of images locally while other methods like "Coherent Structure Tracking" or "Balltracking" exploit the coherency of photospheric granules to track them and use the group motions of the granules as a proxy of the average plasma flows advecting them. Recently, neural network computing has been used in conjunction with numerical models of the Sun to be able to recover the full velocity vector in photospheric plasma from continuum images. We experiment with a new architecture for the DeepVel neural network which takes inspiration from the U-Net architecture. Simulation data of the Quiet Sun and Active Regions are then used to evaluate the response at granular and supergranular scales of the aforementioned method.
更多查看译文
关键词
active region,granulation,photosphere,neural networks,simulations,sunspots,supergranulation,velocity fields
AI 理解论文
溯源树
样例
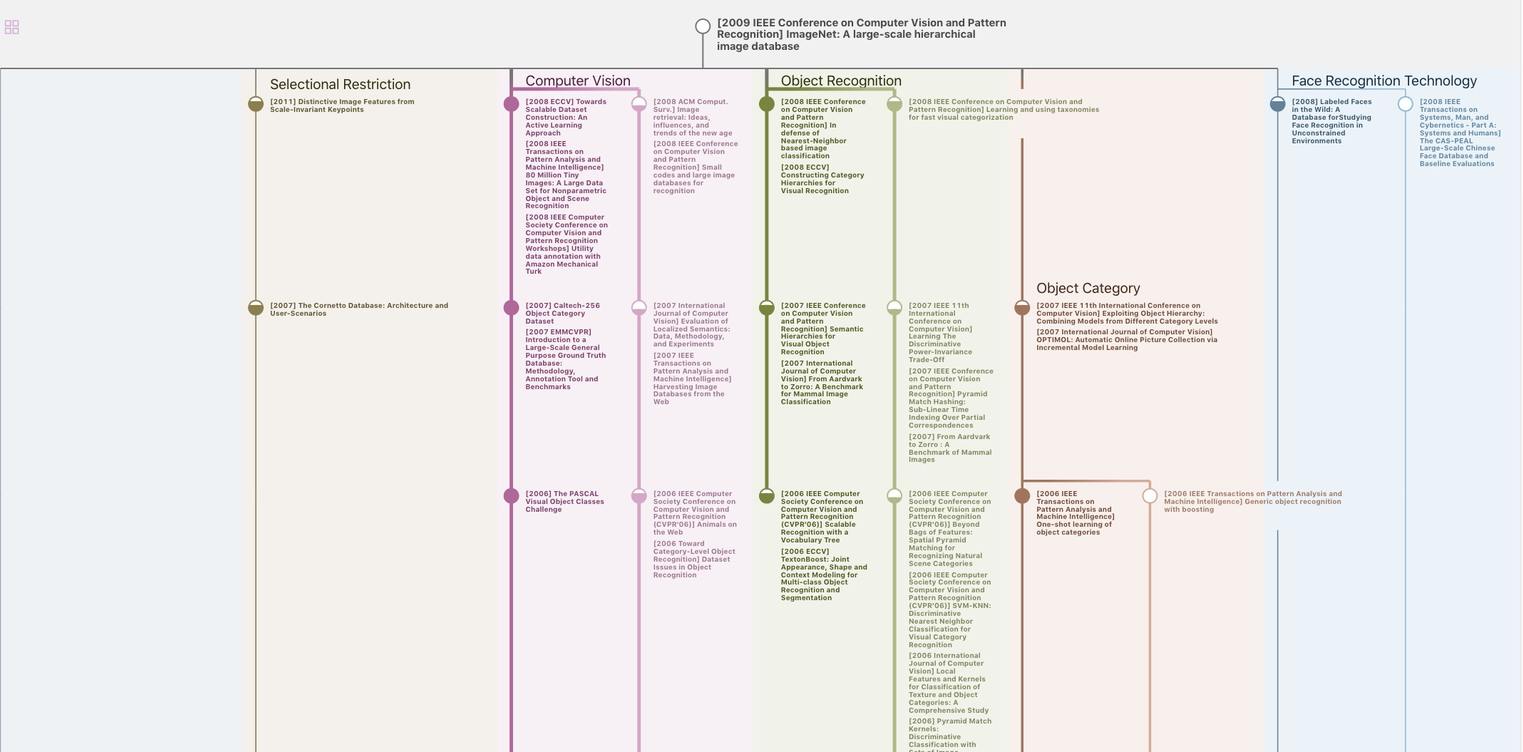
生成溯源树,研究论文发展脉络
Chat Paper
正在生成论文摘要