Generating Probabilistic Next-Day Severe Weather Forecasts From Convection-Allowing Ensembles Using Random Forests
WEATHER AND FORECASTING(2020)
摘要
Extracting explicit severe weather forecast guidance from convection-allowing ensembles (CAEs) is challenging since CAEs cannot directly simulate individual severe weather hazards. Currently, CAE-based severe weather probabilities must be inferred from one or more storm-related variables, which may require extensive calibration and/or contain limited information. Machine learning (ML) offers a way to obtain severe weather forecast probabilities from CAEs by relating CAE forecast variables to observed severe weather reports. This paper develops and verifies a random forest (RF)-based ML method for creating day 1 (1200-1200 UTC) severe weather hazard probabilities and categorical outlooks based on 0000 UTC Storm-Scale Ensemble of Opportunity (SSEO) forecast data and observed Storm Prediction Center (SPC) storm reports. RF forecast probabilities are compared against severe weather forecasts from calibrated SSEO 2-5-km updraft helicity (UH) forecasts and SPC convective outlooks issued at 0600 UTC. Continuous RF probabilities routinely have the highest Brier skill scores (BSSs), regardless of whether the forecasts are evaluated over the full domain or regional/seasonal subsets. Even when RF probabilities are truncated at the probability levels issued by the SPC, the RF forecasts often have BSSs better than or comparable to correspondingUHand SPC forecasts. Relative to theUHand SPC forecasts, the RF approach performs best for severe wind and hail prediction during the spring and summer (i.e., March-August). Overall, it is concluded that the RF method presented here provides skillful, reliable CAE-derived severe weather probabilities that may be useful to severe weather forecasters and decision-makers.
更多查看译文
AI 理解论文
溯源树
样例
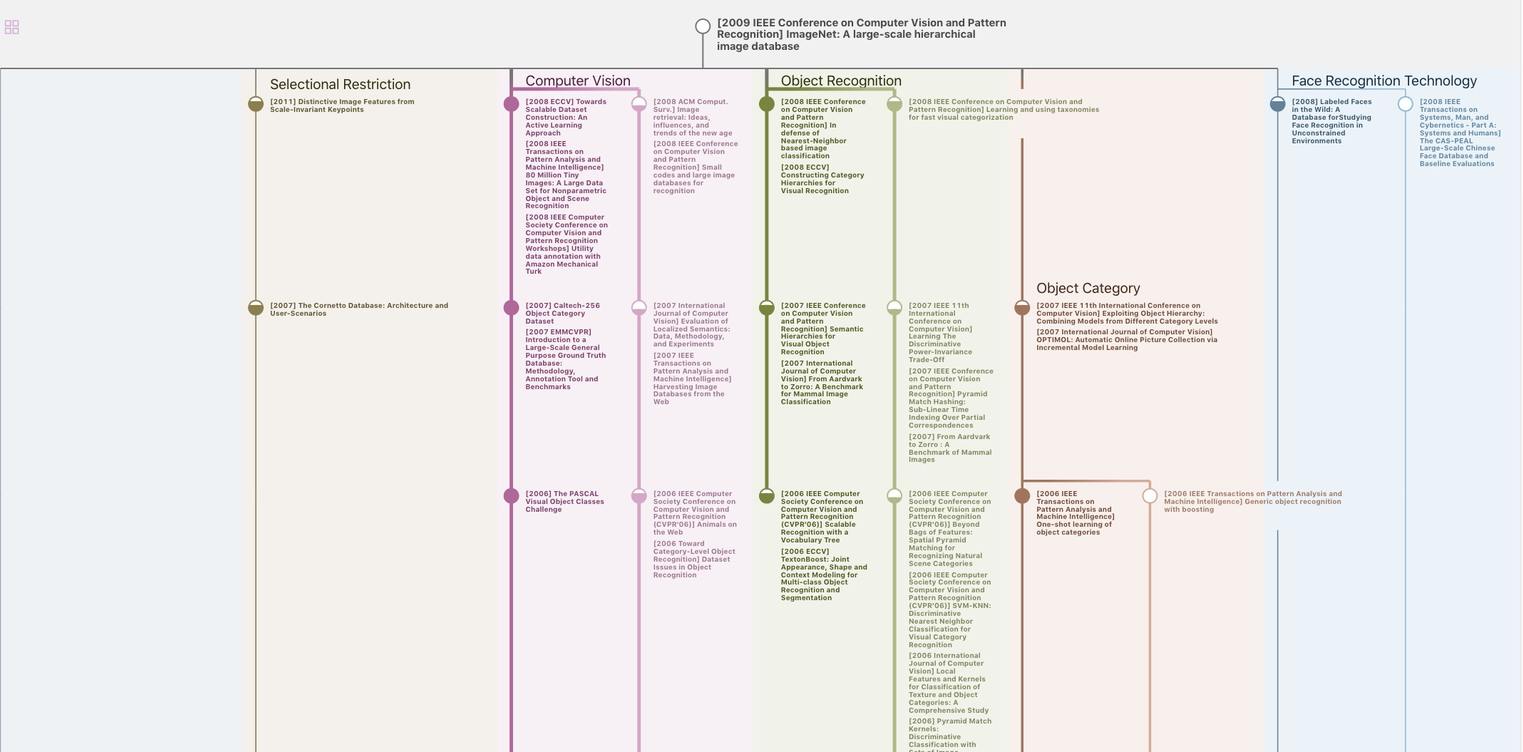
生成溯源树,研究论文发展脉络
Chat Paper
正在生成论文摘要