Machine Learning for Detecting Potato Populations Using SSR Markers
IRANIAN JOURNAL OF SCIENCE AND TECHNOLOGY TRANSACTION A-SCIENCE(2020)
Abstract
A study was performed to determine how bioinformatics algorithms could be employed to classify and estimate 50 potato commercial and wild populations using microsatellite (SSR) markers. In this study, 40 SSR primers were used for estimating the genetic structure of all 50 potato populations. The data were generated in two different experiments including 32 wild and 18 commercial accessions. The results indicate that 31 SSR primers were polymorphic, and also these primers with 334 alleles were analyzed. Analyses through data cleaning, attribute weighting, and machine learning classified the populations into different categories. The machine learning methods used here for classifying the potato accessions discovered that the SS110 was the best SSR primer for the potato population analysis, and also, it can be emphasized that the machine learning analyses are the best tool for the classification of potato populations based on populations specific genetic architecture.
MoreTranslated text
Key words
Accession, Algorithm, Commercial, Molecular marker, Supervised
AI Read Science
Must-Reading Tree
Example
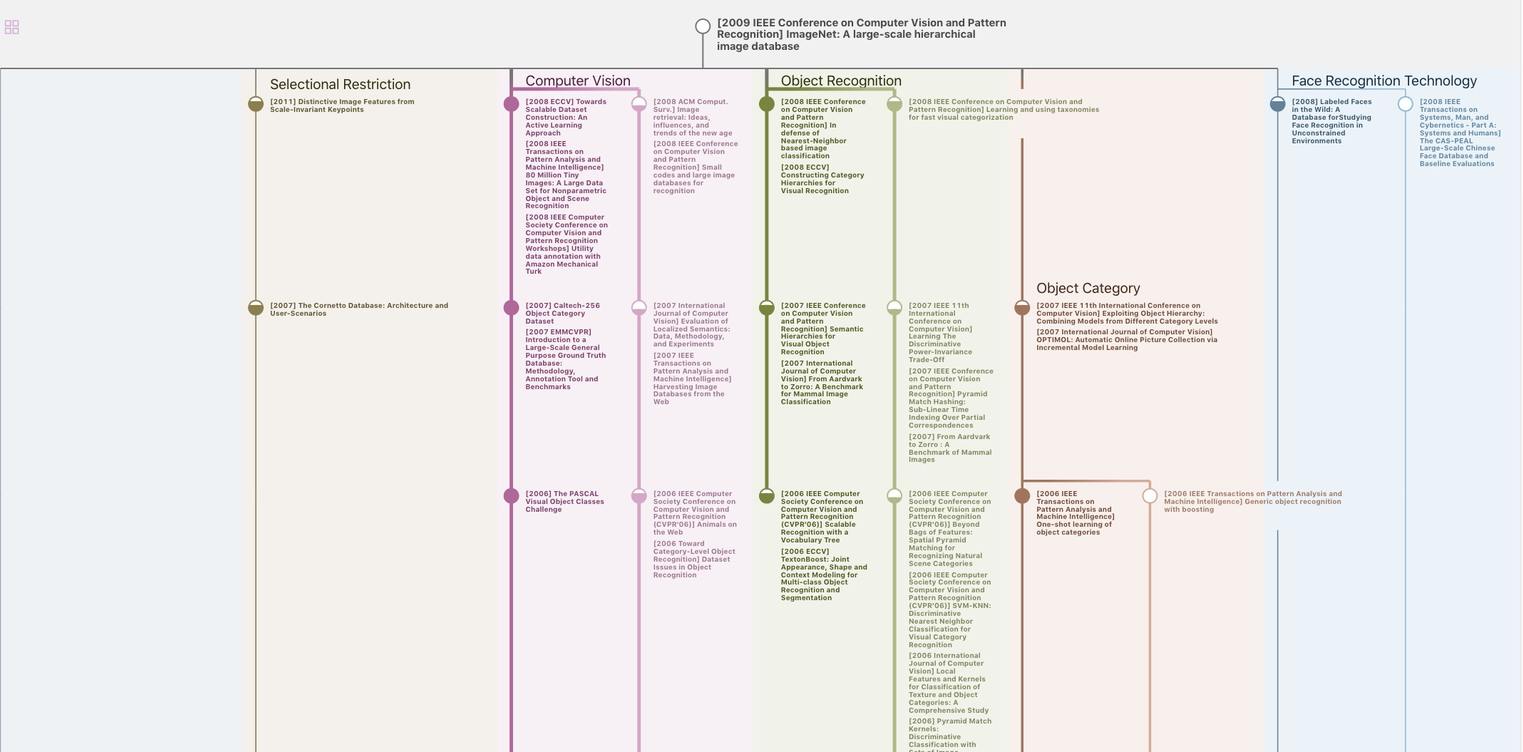
Generate MRT to find the research sequence of this paper
Chat Paper
Summary is being generated by the instructions you defined