Modeling of MEMS gyro drift based on wavelet threshold denoising and improved Elman neural network
2019 14th IEEE International Conference on Electronic Measurement & Instruments (ICEMI)(2019)
Abstract
Low signal-noise-ratio (SNR) of micro-electro-mechanical system (MEMS) gyro has directly restricted the accuracy and practical application of MEMS inertial measurement unit (MIMU). Aiming at diminishing accumulative errors resulted from inherent drift, a MEMS gyro drift modeling method based on wavelet threshold denoising and improved Elman neural network is proposed in this paper. To observe noise characteristics, gyro signals are first processed by Allan variance analysis. The wavelet threshold denoising algorithm is employed to separate random drift and high-frequency white noise. Due to the nonstationary and time-varying characteristics of drift error, an improved Elman neural network is designed for drift modeling and compensation. Experimental results demonstrate that the proposed method achieves high-precision drift modeling and has superior performance compared with traditional time series analysis.
MoreTranslated text
Key words
MEMS gyro,wavelet threshold denoising,Elman neural network,random drift,Allan variance.
AI Read Science
Must-Reading Tree
Example
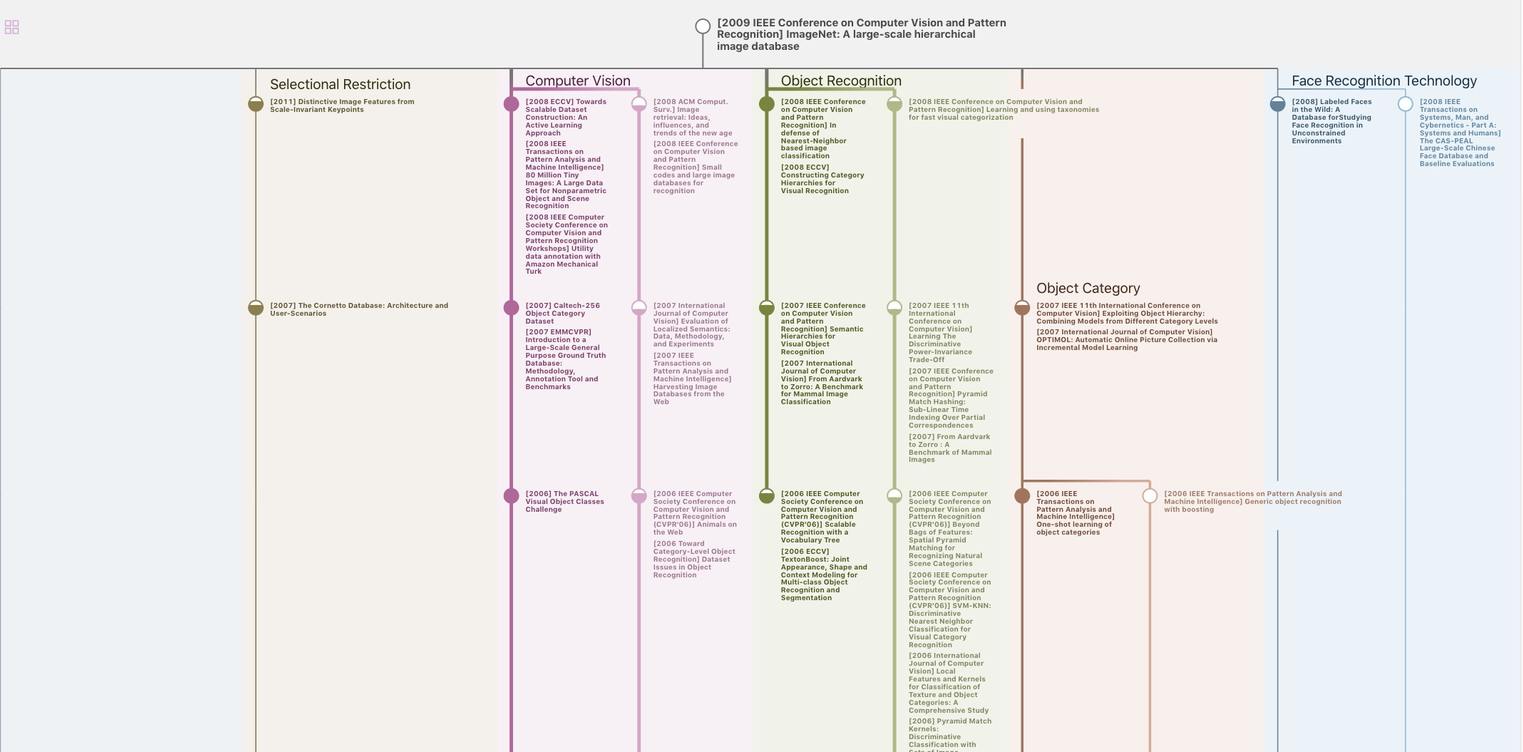
Generate MRT to find the research sequence of this paper
Chat Paper
Summary is being generated by the instructions you defined