Using Machine Learning To Predict Immunotherapy Response In Advanced Melanoma.
JOURNAL OF CLINICAL ONCOLOGY(2020)
摘要
10010 Background: Several predictors of response to checkpoint inhibitors show potential, but use pathological assays and/or molecular characterization, which limits their clinical utility outside of the academic setting. We aimed to develop a streamlined approach by leveraging information immediately available through standard care. Here, we present a computational method that integrates deep learning on histology specimens with clinicodemographic variables to predict treatment outcomes in advanced melanoma. Methods: We used hematoxylin and eosin (H&E) sections from 72 patients ( n= 153 slides) from New York University (NYU) to build a Segmentation Classifier that distinguishes tumor, lymphocyte, and connective tissue. Using pre-treatment H&E slides from 121 NYU patients ( n =302 slides), we trained a Response Classifier to predict response by selectively analyzing tumor regions. We then developed a logistic regression classifier that combines neural network output with clinicodemographic variables. The classifiers were tested on an independent cohort of 32 patients from Vanderbilt University ( n =42 slides). Area under the curve (AUC) was calculated as a measure of prediction accuracy. Results: The Segmentation Classifier distinguished tumor, lymphocyte, and connective tissue with AUCs 0.886-0.984. For the Response Classifier, optimal learning conditions were identified through training on NYU patients and testing on Vanderbilt patients (AUCs 0.685 and 0.728, respectively). The fully trained Response Classifier performed with AUC 0.711 on Vanderbilt patients. The logistic regression model performed with enhanced prediction accuracy with AUC 0.803 on NYU patients and AUC 0.793 on Vanderbilt patients. Conclusions: Histology slides and patients’ clinicodemographic characteristics are readily available through routine standard of care and have the potential to predict immunotherapy response. Our approach is time-efficient, reproducible, and requires minimal resource allocation, thus overcoming multiple common barriers to generalizability for contemporary biomarkers.
更多查看译文
AI 理解论文
溯源树
样例
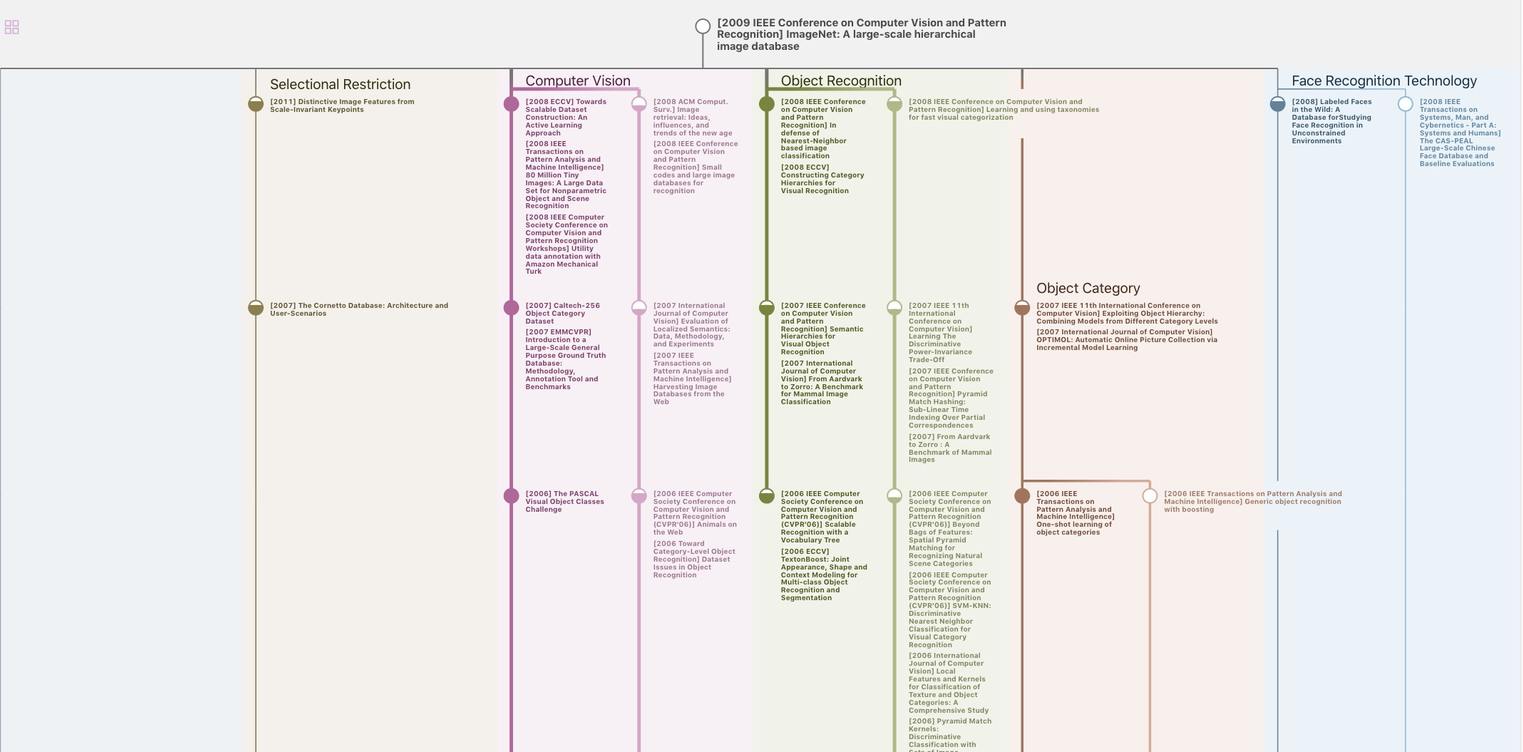
生成溯源树,研究论文发展脉络
Chat Paper
正在生成论文摘要