A Learning Frequency-Aware Feature Siamese Network for Real-Time Visual Tracking
ELECTRONICS(2020)
摘要
Visual object tracking by Siamese networks has achieved favorable performance in accuracy and speed. However, the features used in Siamese networks have spatially redundant information, which increases computation and limits the discriminative ability of Siamese networks. Addressing this issue, we present a novel frequency-aware feature (FAF) method for robust visual object tracking in complex scenes. Unlike previous works, which select features from different channels or layers, the proposed method factorizes the feature map into multi-frequency and reduces the low-frequency information that is spatially redundant. By reducing the low-frequency map's resolution, the computation is saved and the receptive field of the layer is also increased to obtain more discriminative information. To further improve the performance of the FAF, we design an innovative data-independent augmentation for object tracking to improve the discriminative ability of tracker, which enhanced linear representation among training samples by convex combinations of the images and tags. Finally, a joint judgment strategy is proposed to adjust the bounding box result that combines intersection-over-union (IoU) and classification scores to improve tracking accuracy. Extensive experiments on 5 challenging benchmarks demonstrate that our FAF method performs favorably against SOTA tracking methods while running around 45 frames per second.
更多查看译文
关键词
deep learning,computer version,object tracking
AI 理解论文
溯源树
样例
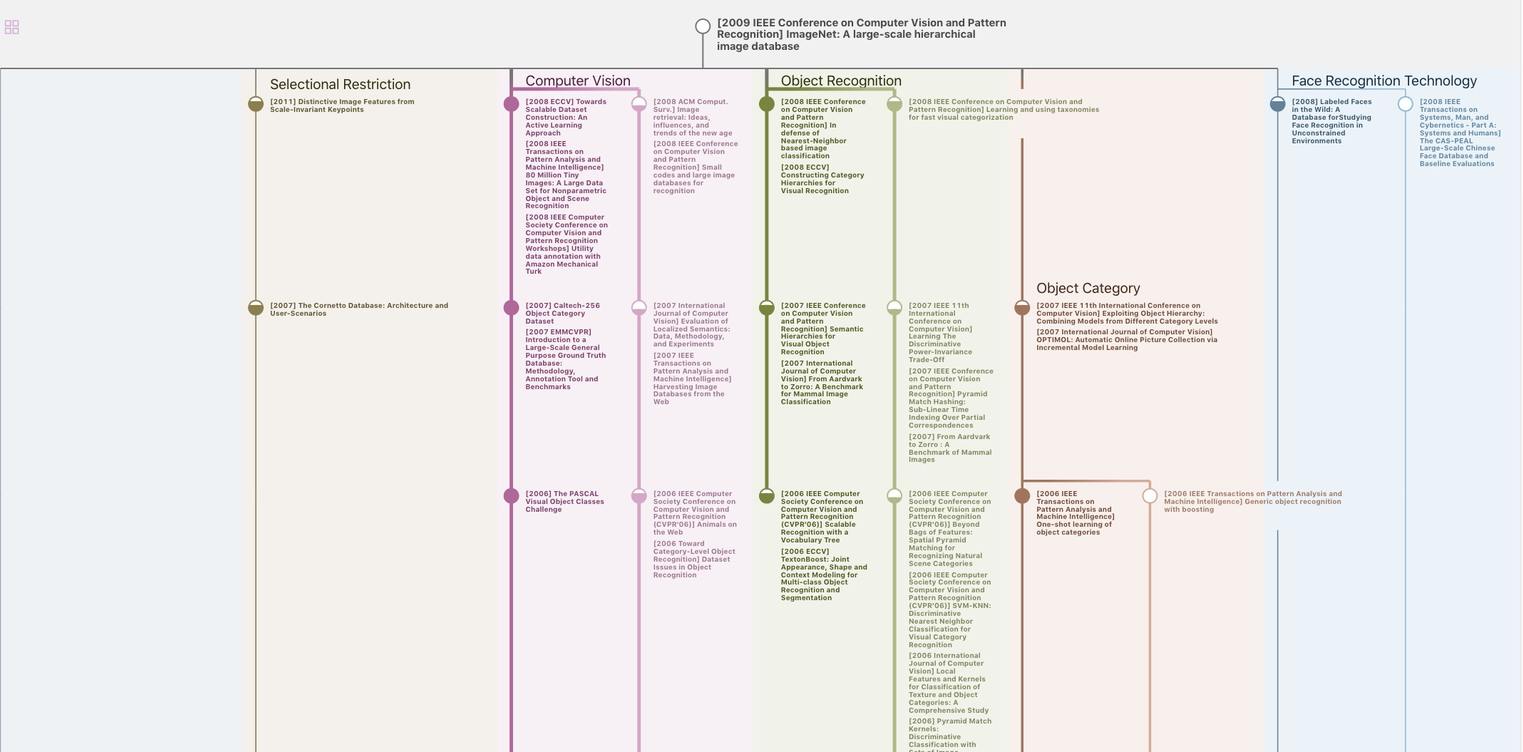
生成溯源树,研究论文发展脉络
Chat Paper
正在生成论文摘要