An Enhanced Weighted Greedy Analysis Pursuit Algorithm with Application to EEG Signal Reconstruction
International Journal of Imaging Systems and Technology(2020)
关键词
compressed sensing,cosparse analysis model,EEG signal reconstruction,Greedy Analysis Pursuit,sparsity
AI 理解论文
溯源树
样例
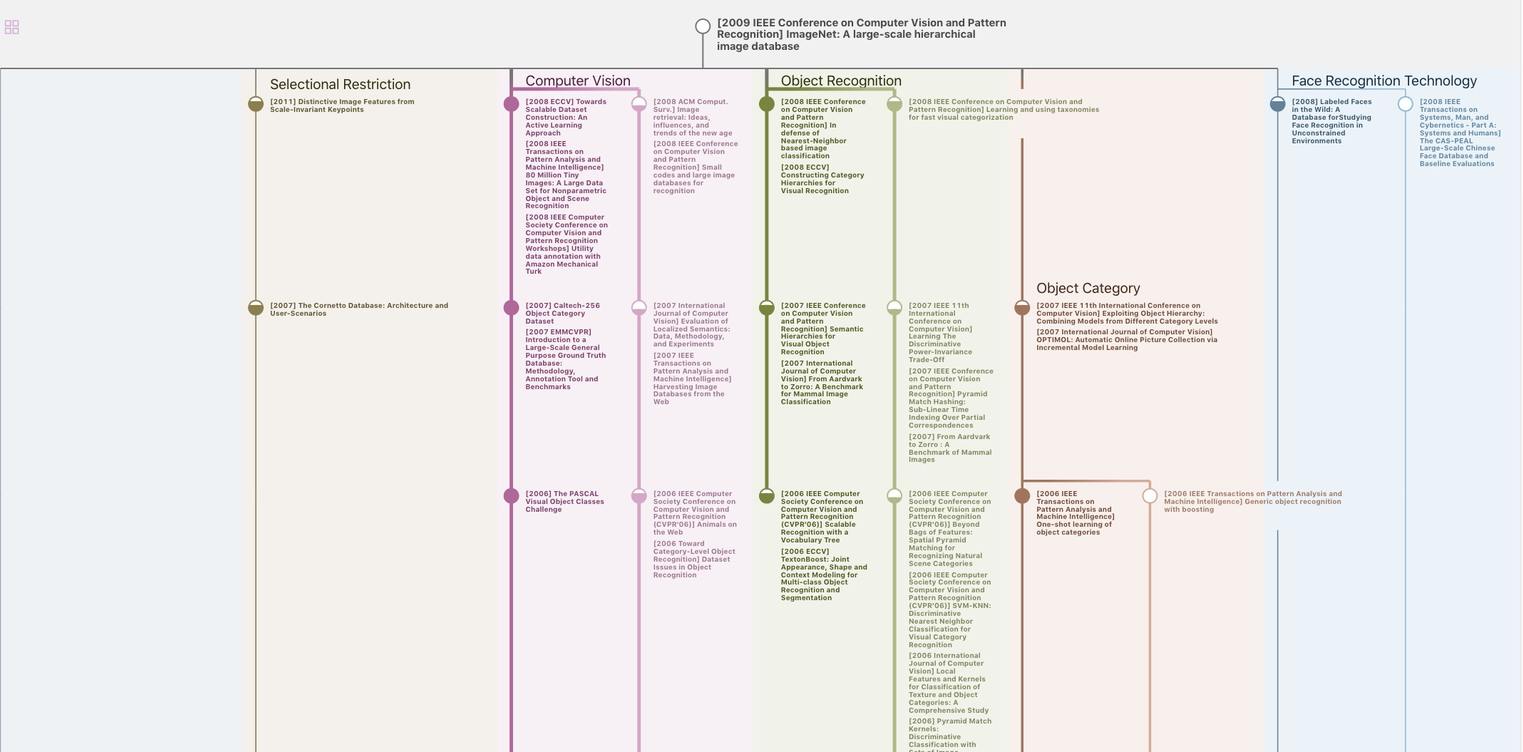
生成溯源树,研究论文发展脉络
Chat Paper
正在生成论文摘要