Visual-Guided Robotic Object Grasping Using Dual Neural Network Controllers.
IEEE Trans. Ind. Informatics(2021)
Abstract
It has been a challenging task for a robotic arm to accurately reach and grasp objects, which has drawn much research attention. This article proposes a robotic hand–eye coordination system by simulating the human behavior pattern to achieve a fast and robust reaching ability. This is achieved by two neural-network-based controllers, including a rough reaching movement controller implemented by a pretrained radial basis function for rough reaching movements, and a correction movement controller built from a specifically designed brain emotional nesting network (BENN) for smooth correction movements. In particular, the proposed BENN is designed with high nonlinear mapping ability, with its adaptive laws derived from the Lyapunov stability theorem; from this, the robust tracking performance and accordingly the stability of the proposed control system are guaranteed by the utilization of the $H^{\infty }$ control approach. The proposed BENN is validated and evaluated by a chaos synchronization simulation, and the overall control system by object grasping tasks through a physical robotic arm in a real-world environment. The experimental results demonstrate the superiority of the proposed control system in reference to those with single neural networks.
MoreTranslated text
Key words
Neural-network-based controller,robotic hand–eye coordination,robotic reaching movement
AI Read Science
Must-Reading Tree
Example
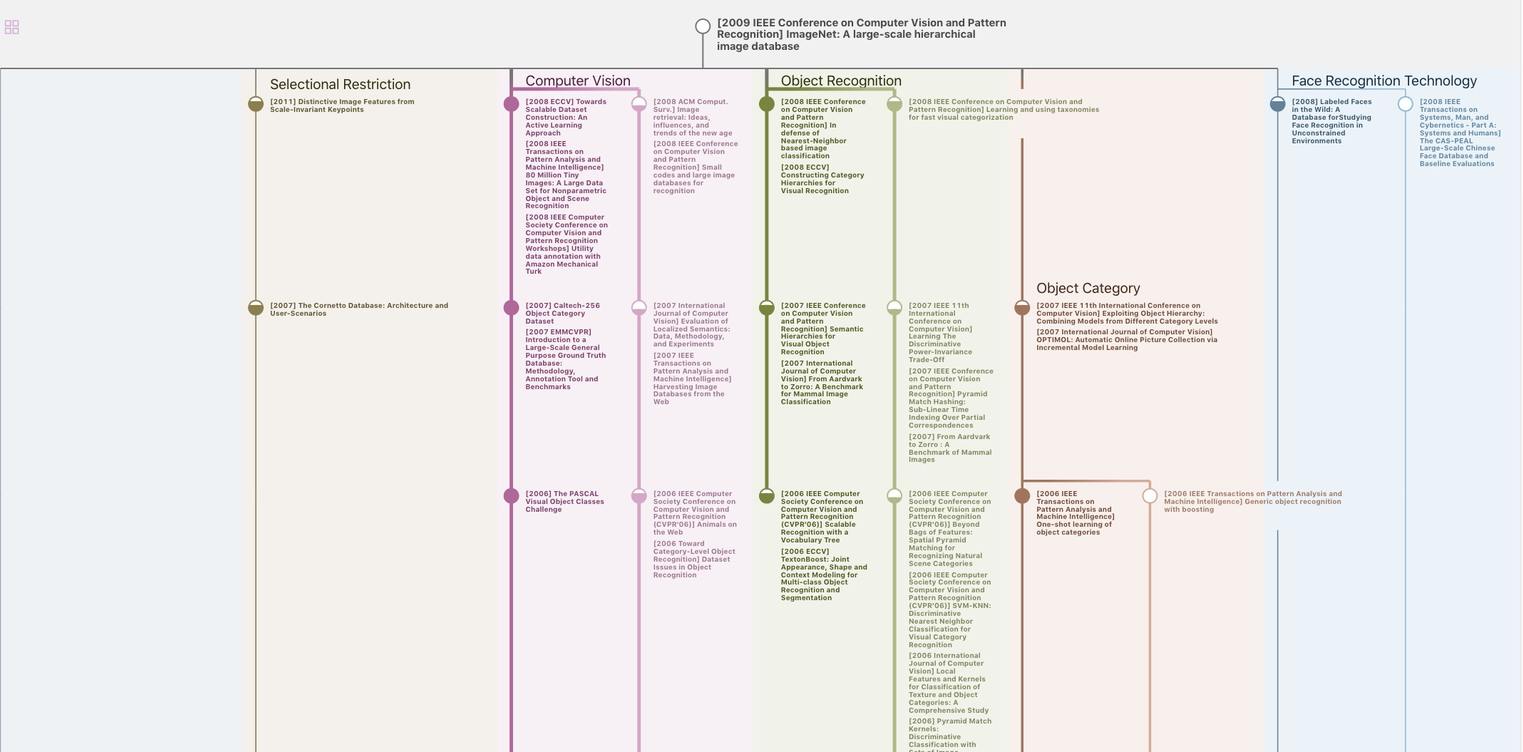
Generate MRT to find the research sequence of this paper
Chat Paper
Summary is being generated by the instructions you defined