Mv-Gnn: Multi-View Graph Neural Network For Compression Artifacts Reduction
IEEE TRANSACTIONS ON IMAGE PROCESSING(2020)
Abstract
Inevitable compression artifacts in multi-view video (MVV) can clearly degrade the quality of experience in many interaction-oriented 3D visual applications. Under the framework of asymmetric coding, low-quality images can be enhanced with high-quality images from the neighboring viewpoints considering the similarity among different views. However, compression artifacts and warping error cause different cross-view quality gaps for various sequences, and thus the contribution of cross-view priors can hardly be located and considered in previous works. In this paper, we propose a multi-view graph neural network (MV-GNN) to reduce compression artifacts in multi-view compressed images. We dedicate to design a fusion mechanism which can exploit contributions from neighboring viewpoints and meanwhile suppress the misleading information. In our method, a GNN-based fusion mechanism is designed to fuse the cross-view information under the aggregation and update mechanism of GNN. Experiments show that 1.672 dB and 0.0242 average gains on PSNR and SSIM metrics can be obtained, respectively. For the subjective evaluations, blocking effect in the compressed images are clearly suppressed and the damaged object boundary are better recovered. The experimental results demonstrate that our MV-GNN outperforms the state-of-the-art methods.
MoreTranslated text
Key words
Image coding, Neural networks, Three-dimensional displays, Transform coding, Feature extraction, Image reconstruction, Visualization, Compression artifacts reduction, graph neural network, multi-view video compression
AI Read Science
Must-Reading Tree
Example
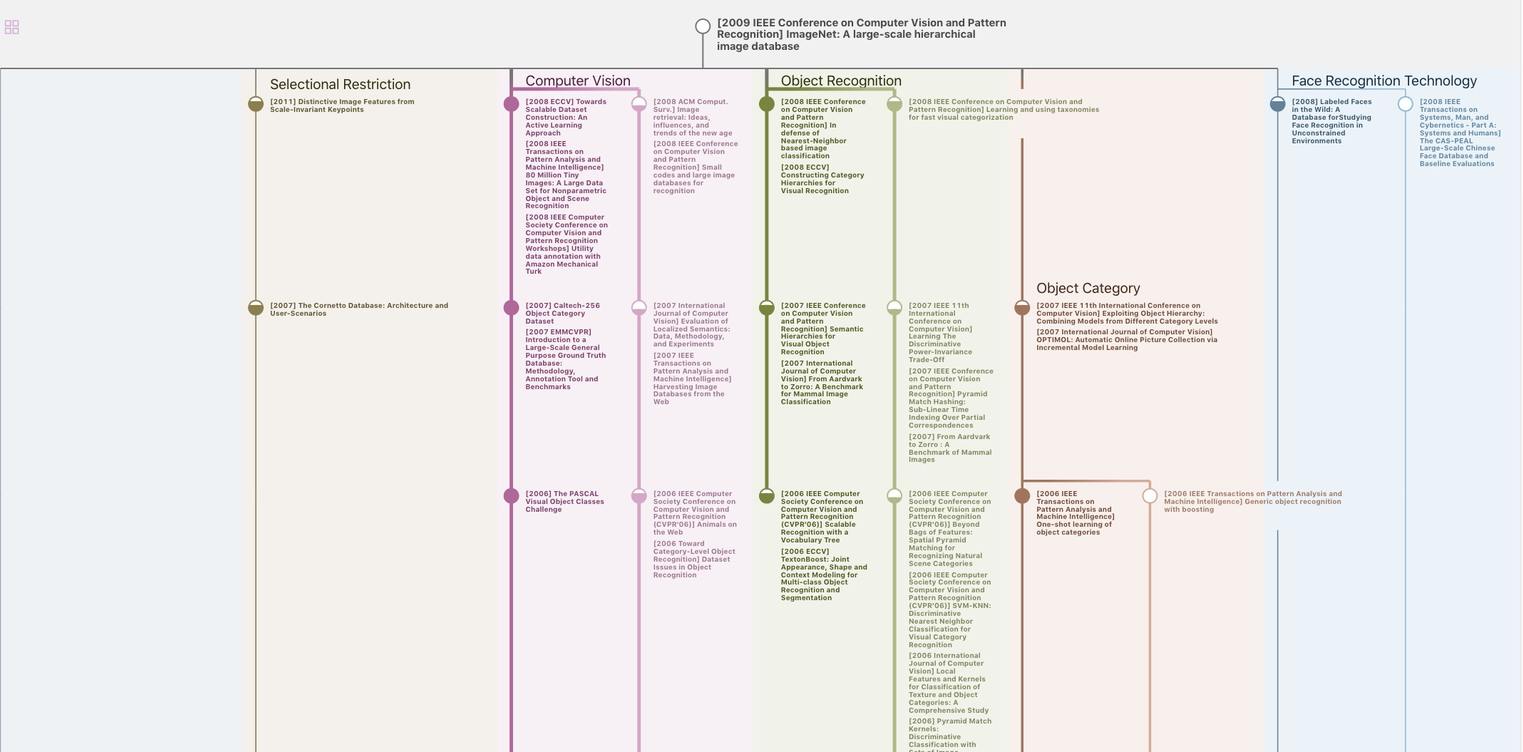
Generate MRT to find the research sequence of this paper
Chat Paper
Summary is being generated by the instructions you defined