A Context-Aware Location Recommendation System for Tourists Using Hierarchical LSTM Model
Sustainability(2020)
摘要
The significance of contextual data has been recognized by analysts and specialists in numerous disciplines such as customization, data recovery, ubiquitous and versatile processing, information mining, and management. While a generous research has just been performed in the zone of recommender frameworks, by far most of the existing approaches center on prescribing the most relevant items to customers. It usually neglects extra-contextual information, for example time, area, climate or the popularity of different locations. Therefore, we proposed a deep long-short term memory (LSTM) based context-enriched hierarchical model. This proposed model had two levels of hierarchy and each level comprised of a deep LSTM network. In each level, the task of the LSTM was different. At the first level, LSTM learned from user travel history and predicted the next location probabilities. A contextual learning unit was active between these two levels. This unit extracted maximum possible contexts related to a location, the user and its environment such as weather, climate and risks. This unit also estimated other effective parameters such as the popularity of a location. To avoid feature congestion, XGBoost was used to rank feature importance. The features with no importance were discarded. At the second level, another LSTM framework was used to learn these contextual features embedded with location probabilities and resulted into top ranked places. The performance of the proposed approach was elevated with an accuracy of 97.2%, followed by gated recurrent unit (GRU) (96.4%) and then Bidirectional LSTM (94.2%). We also performed experiments to find the optimal size of travel history for effective recommendations.
更多查看译文
关键词
context aware,recommendation system,LSTM,feature importance,XGBoost,tourism
AI 理解论文
溯源树
样例
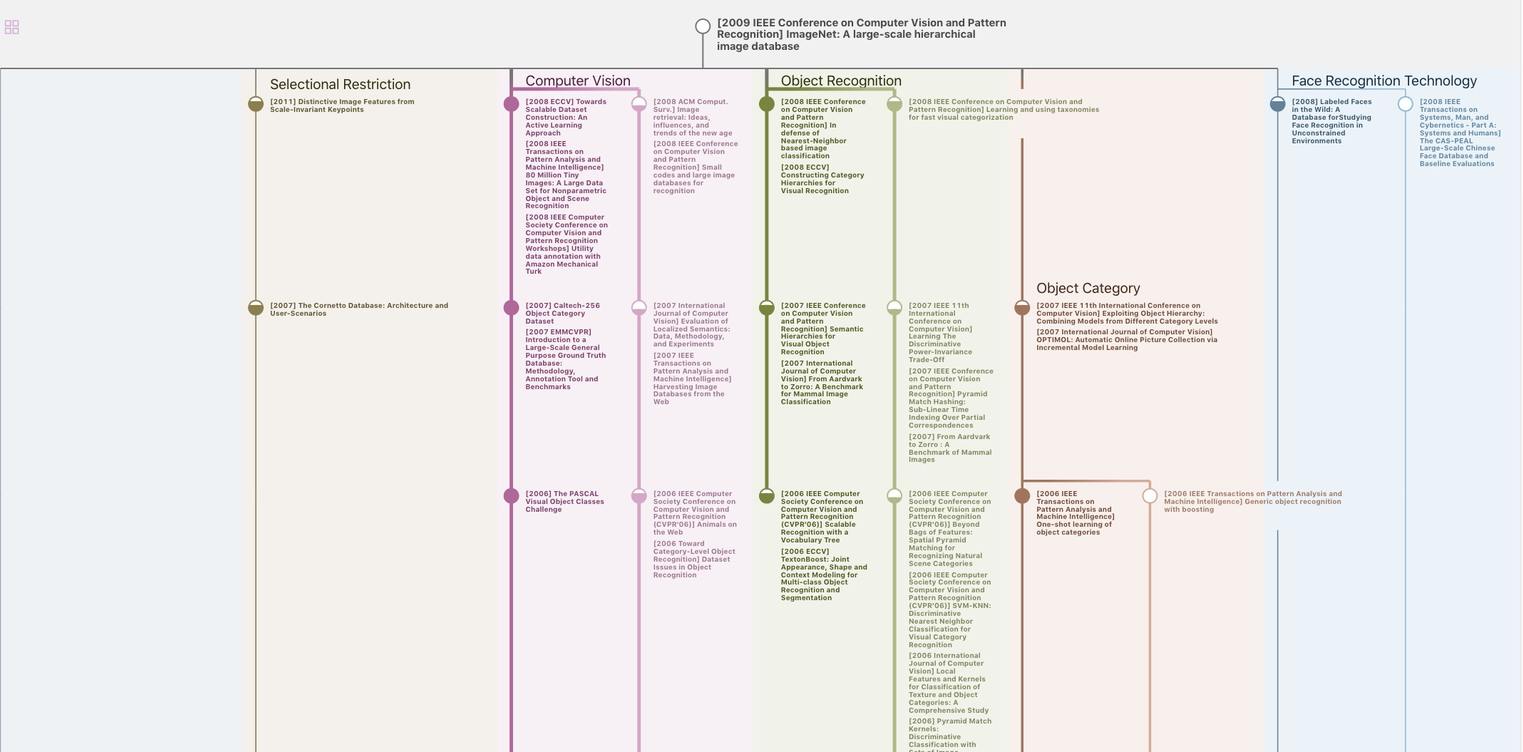
生成溯源树,研究论文发展脉络
Chat Paper
正在生成论文摘要