Bias Decomposition In The Value-At-Risk Calculation By A Garch(1,1)
INTERNATIONAL JOURNAL OF COMPUTATIONAL ECONOMICS AND ECONOMETRICS(2020)
Abstract
The recent researches show that value-at-risk (VaR) estimations are biased and is calculated conservatively. Bao and Ullah (2004) proved the bias of an ARCH(1) model for VaR can be decomposed in to two parts: bias due to the returns' misspecification distributional assumption for GARCH(1,1), i.e., (Bias1) and bias due to estimation error, i.e., (Bias(2)). Using quasi maximum likelihood estimation method this paper intends to find an analytical framework for the two sources of bias. We generate returns from Normal and t-student distributions, then estimate the GARCH(1,1) under Normal and t-student assumptions. Our findings reveal that Bias(1)equals to zero for the Normal likelihood function, but Bias(2)not equal 0. Also, Bias(1)and Bias(2)are not zero for the t-student likelihood function as analytically were expected, however, all the biases become modest, when the number of observations and degree of freedom gets large.
MoreTranslated text
Key words
VaR,value-at-risk,GARCH(1,1),second-order bias
AI Read Science
Must-Reading Tree
Example
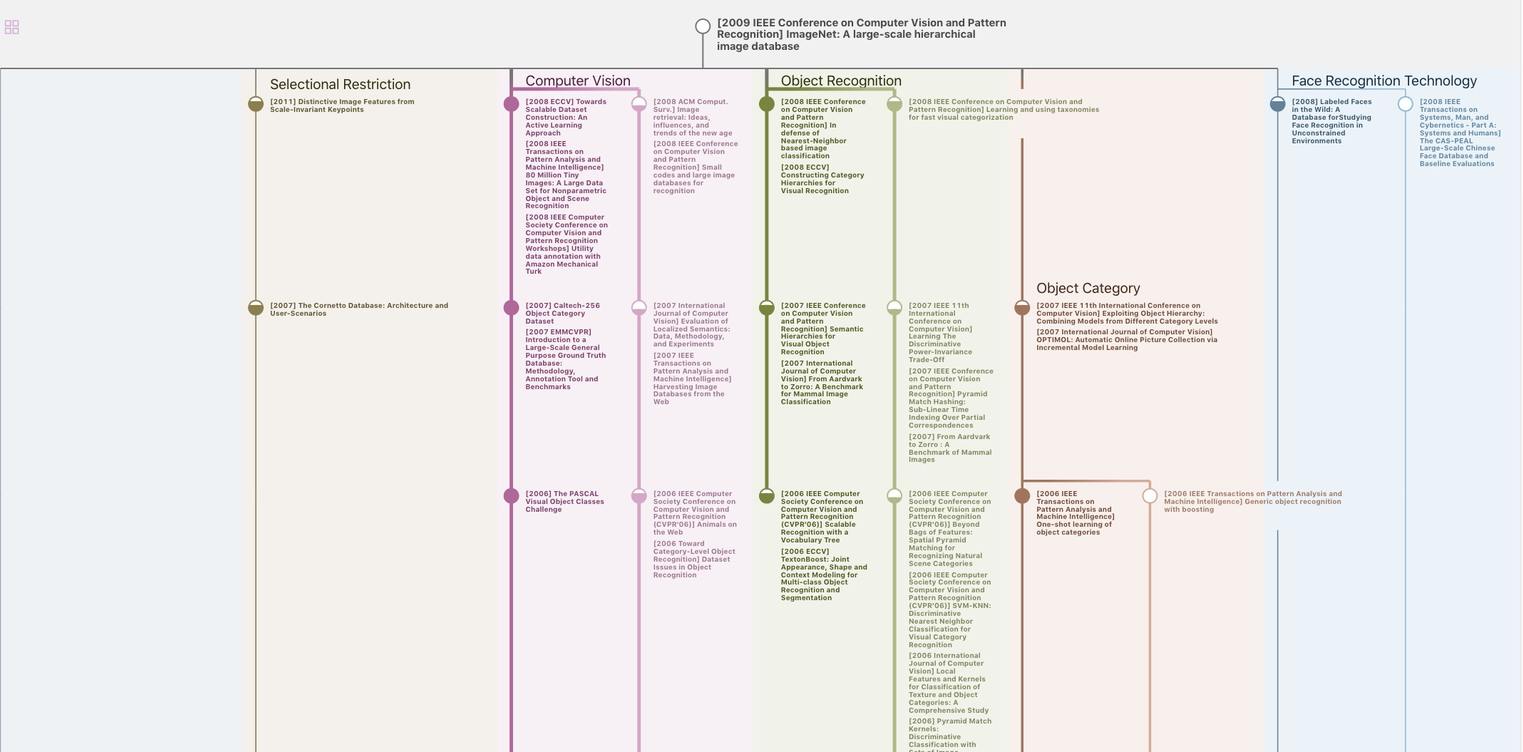
Generate MRT to find the research sequence of this paper
Chat Paper
Summary is being generated by the instructions you defined