A Category-Based Calibration Approach With Fault Tolerance for Air Monitoring Sensors
IEEE Sensors Journal(2020)
摘要
Air pollution monitoring has attracted much attention in recent years because of the deterioration of the environment. Standard stations, installed by governments with a high cost, can provide reliable air quality information; whereas a large number of portable air monitoring sensors with low cost are widely used and output less precise results. In this paper, we propose a category-based calibration approach (CCA) using machine learning algorithms for such portable sensors. Compared with traditional methods that often learn a single regression model, CCA includes multiple regression models according to pollutant concentration categories, and builds a more accurate mapping from sensor readings to reference. Furthermore, CCA introduces two fault-tolerance modules: classification tolerance and sample tolerance. The former mitigates the impact of misclassification for concentration category, and the latter improves the robustness of individual regression model. Our approach is evaluated on carbon monoxide (CO) and ozone (O-3) from two cities of China. The experiment results show that CCA has a better performance than traditional calibration models in both accuracy and robustness.
更多查看译文
关键词
Air quality,air monitoring sensors,machine learning,category-based calibration,fault-tolerance strategy
AI 理解论文
溯源树
样例
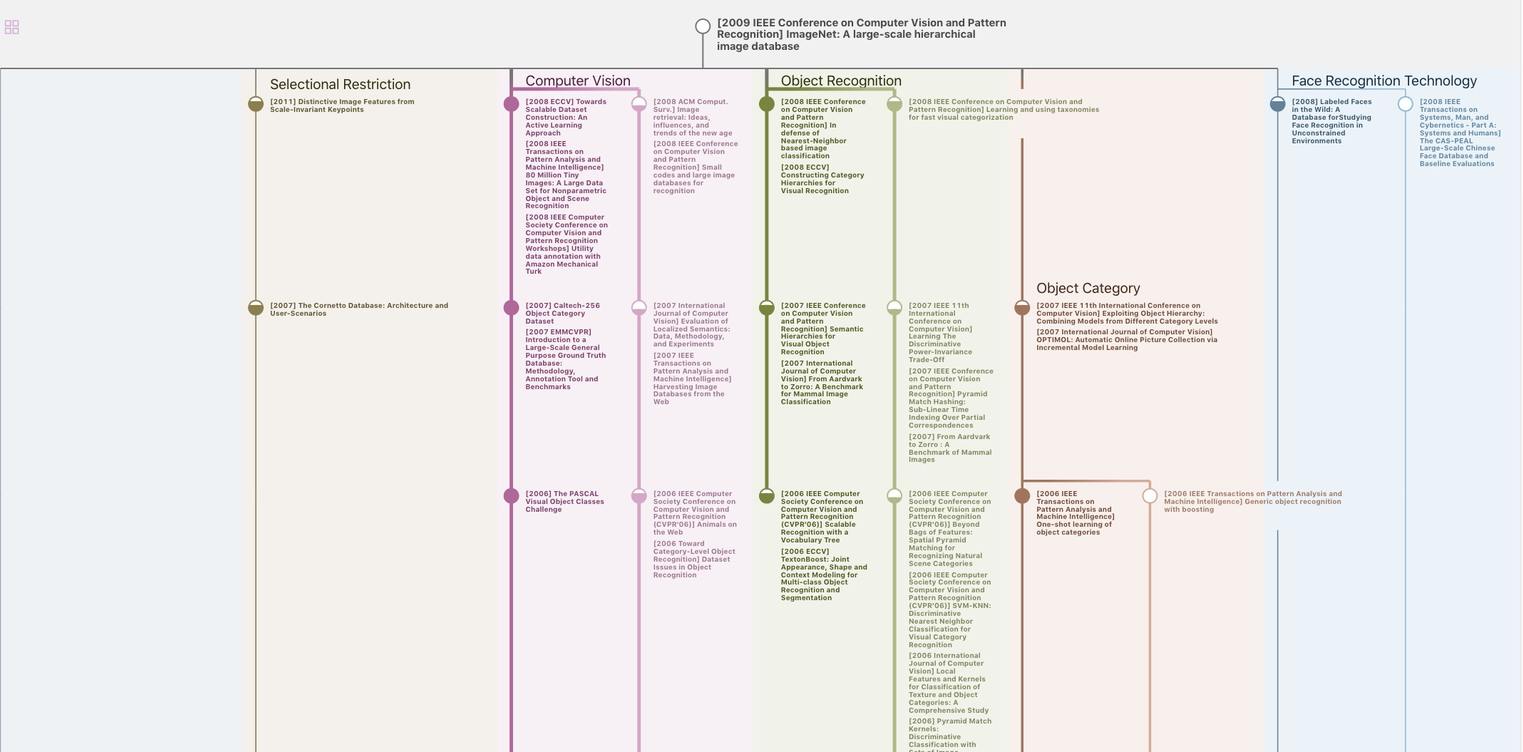
生成溯源树,研究论文发展脉络
Chat Paper
正在生成论文摘要