A Multi-Input Neural Network With Dense Flow And Spatio-Temporal Features For Anomaly Detection
2019 FIFTEENTH INTERNATIONAL CONFERENCE ON INFORMATION PROCESSING (ICINPRO): INTERNET OF THINGS(2019)
摘要
IoT today is expanding at a rapid rate. The number of connected devices is expected to hit 70 billion by 2025 and will increase further at an exponential rate. Additionally, the surge in IoT surveillance devices makes manual human monitoring impossible and presents a pressing need for automated monitoring. Thus, we present a paper that aims to detect anomalies from surveillance videos and identify the point and duration of anomaly occurrence in the video as well. We propose a novel multi-input neural network incorporating spatio-temporal features and dense flow features to train our dataset. We show the ability of our model to capture anomalous content better than baseline approaches. The low false alarm rate shows the resistance of our model to normal videos, despite containing a lot of motion. The introduction of our new standardized dataset also opens scope for further research in this field.
更多查看译文
关键词
Anomaly detection, Artificial Intelligence, Deep learning, IoT, Optical Flow, Surveillance
AI 理解论文
溯源树
样例
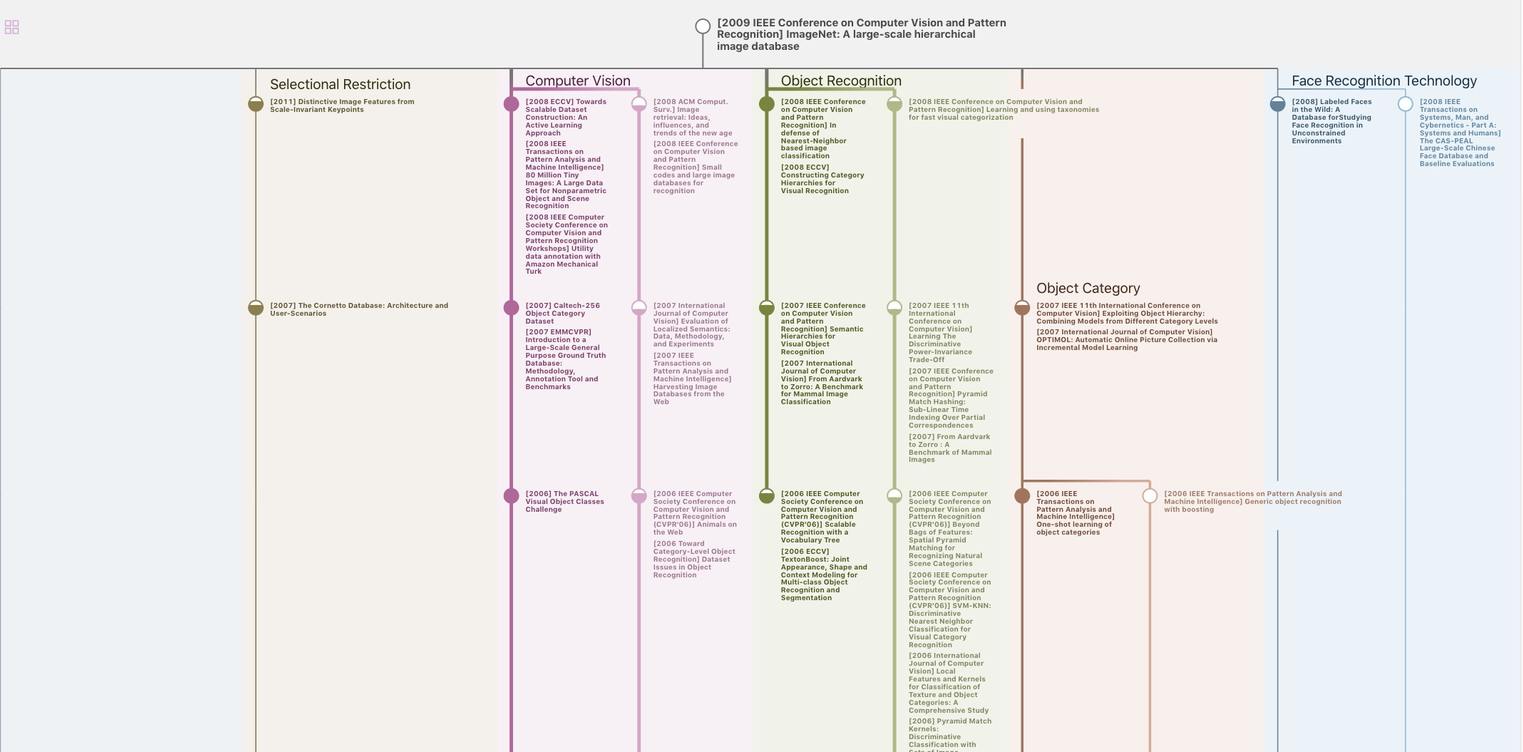
生成溯源树,研究论文发展脉络
Chat Paper
正在生成论文摘要