Towards context-aware collaborative filtering by learning context-aware latent representations
Knowledge-Based Systems(2020)
摘要
Contexts have been proven to be an important source of information that can significantly improve the performance of collaborative filtering (CF), e.g., for recommendation. Most context-aware approaches that are basing on latent factor models assume that contexts share the same latent space with users and items. However such a strategy does not always make sense, e.g., the influence of contextual information may be overestimated. In this paper, we propose a generic framework to learn context-aware latent representations for context-aware collaborative filtering without imposing contexts into latent space of users and items. Contextual contents are combined via a function to produce the contextual influence factor, which is then combined with each latent factor to derive latent representations. We instantiate the generic framework using biased Matrix Factorization for rating prediction task and Bayesian Personalized Ranking (BPR) for item recommendation tasks. Stochastic Gradient Descent (SGD) based optimization procedures are developed to fit the two context-aware models by jointly learning the weight of each context and latent factors of users and items. Experiments conducted on three real-world datasets demonstrate that our context-aware CF model significantly outperforms not only the base models but also the representative context-aware models.
更多查看译文
关键词
Context-aware collaborative filtering,Latent factor models,Top-N recommendation,Matrix factorization
AI 理解论文
溯源树
样例
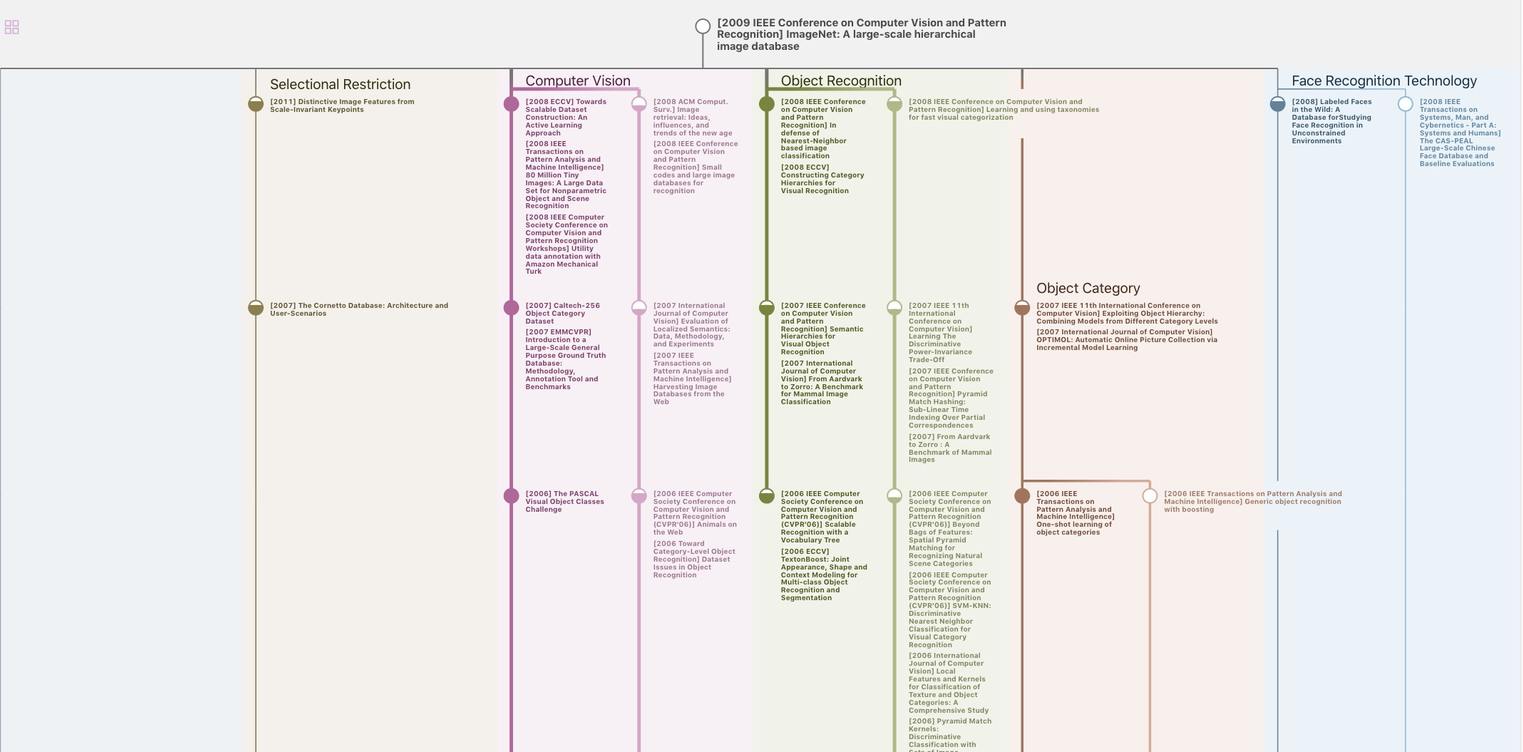
生成溯源树,研究论文发展脉络
Chat Paper
正在生成论文摘要