Shrinkage Estimators for Uplift Regression
european conference on principles of data mining and knowledge discovery(2019)
摘要
Uplift modeling is an approach to machine learning which allows for predicting the net effect of an action (with respect to not taking the action). To achieve this, the training population is divided into two parts: the treatment group, which is subjected to the action, and the control group, on which the action is not taken. Our task is to construct a model which will predict the difference between outcomes in the treatment and control groups conditional on individual objects’ features. When the group assignment is random, the model admits a causal interpretation. When we assume linear responses in both groups, the simplest way of estimating the net effect of the action on an individual is to build two separate linear ordinary least squares (OLS) regressions on the treatment and control groups and compute the difference between their predictions. In classical linear models improvements in accuracy can be achieved through the use of so called shrinkage estimators such as the well known James-Stein estimator, which has a provably lower mean squared error than the OLS estimator. In this paper we investigate the use of shrinkage estimators in the uplift modeling problem. Unfortunately direct generalization of the James-Stein estimator does not lead to improved predictions, nor does shrinking treatment and control models separately. Therefore, we propose a new uplift shrinkage method where estimators in the treatment and control groups are shrunk jointly so as to minimize the error in the predicted net effect of the action. We prove that the proposed estimator does indeed improve on the double regression estimator.
更多查看译文
AI 理解论文
溯源树
样例
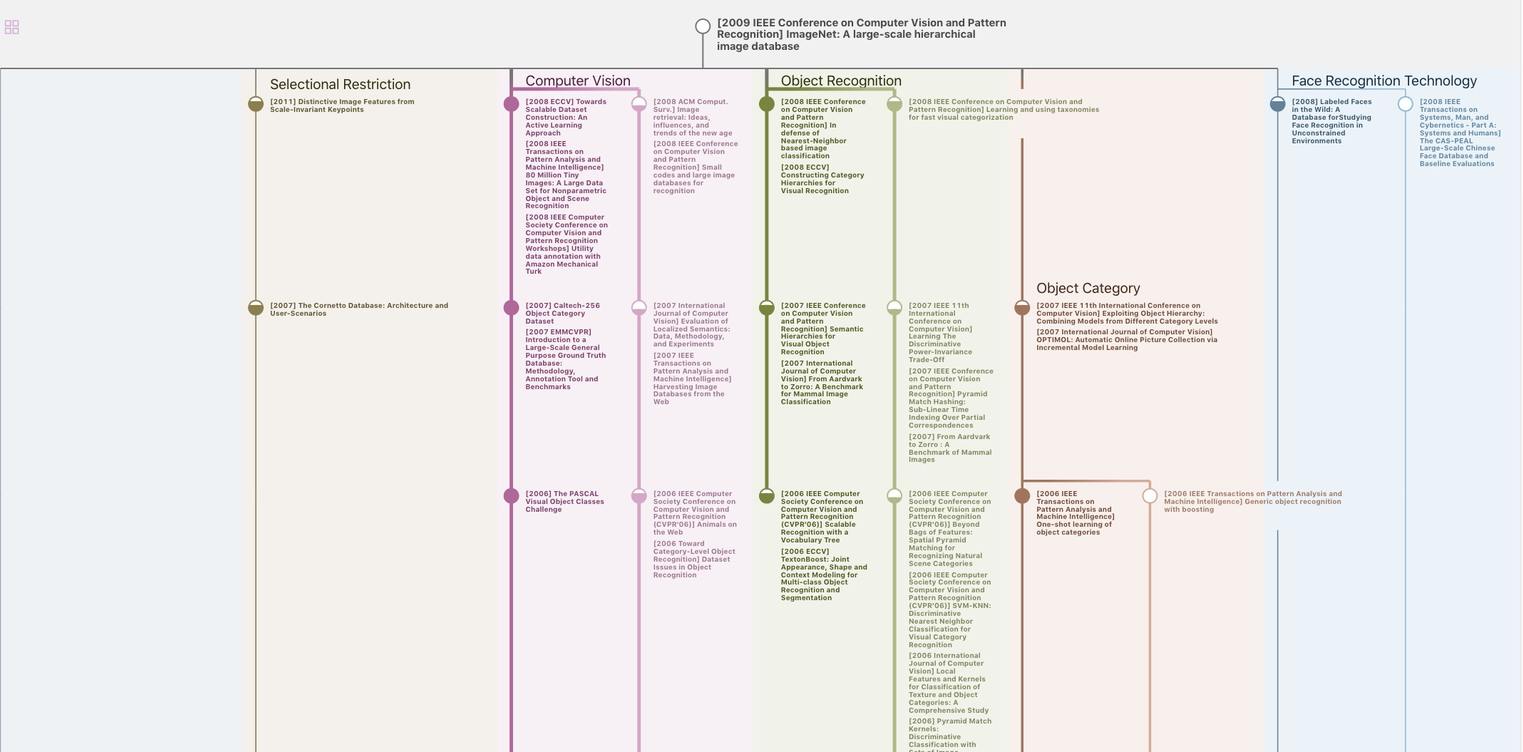
生成溯源树,研究论文发展脉络
Chat Paper
正在生成论文摘要