Neural-Learning-Based Force Sensorless Admittance Control for Robots With Input Deadzone
IEEE Transactions on Industrial Electronics(2021)
摘要
This article presents a neural network based admittance control scheme for robotic manipulators when interacting with the unknown environment in the presence of the actuator deadzone without needing force sensing. A compliant behavior of robotic manipulators in response to external torques from the unknown environment is achieved by admittance control. Inspired by broad learning system, a flatted neural network structure using radial basis function (RBF) with incremental learning algorithm is proposed to estimate the external torque, which can avoid retraining process if the system is modeled insufficiently. To deal with uncertainties in the robot system, an adaptive neural controller with dynamic learning framework is developed to ensure the tracking performance. Experiments on the Baxter robot have been implemented to test the effectiveness of the proposed method.
更多查看译文
关键词
Adaptive control,admittance control,broad learning,force/torque observer,neural networks (NNs)
AI 理解论文
溯源树
样例
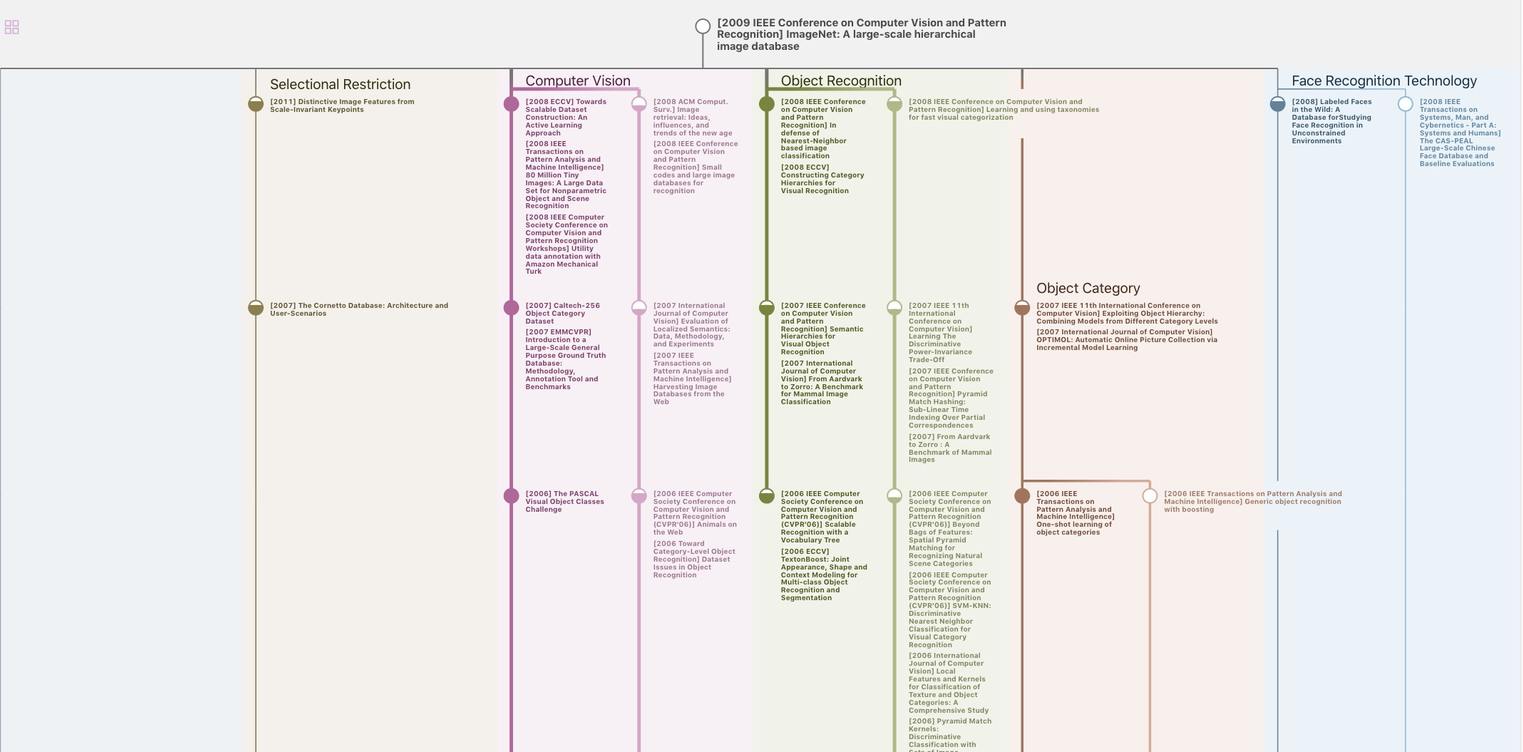
生成溯源树,研究论文发展脉络
Chat Paper
正在生成论文摘要