O8C.1 A probabilistic bias-analysis method for evaluating disease misclassification in a historical cohort mortality study of trichlorophenol workers
Occupational and Environmental Medicine(2019)
Abstract
Occupational epidemiologists have long considered death certificate inaccuracies a critical issue when conducting historical cohort mortality (HCM) studies. However, the vast majority of these types of studies do not include a quantitative assessment of the impact of disease misclassification on study results. We developed a probabilistic bias-analysis method to evaluate the effect of disease misclassification using ischemic heart disease (IHD) mortality data from a cohort study of New Zealand trichlorophenol workers exposed to 2,3,7,8-tetrachlorodibenzo-p-dioxin (TCDD). The sensitivity and specificity of IHD death certificate diagnoses in high income countries, as described in 14 peer-reviewed validation studies, were used to construct bias parameter distributions for nine simulation scenarios. We defined the parameter distributions for a non-differential disease misclassification scenario first, using a beta distribution for the sensitivity parameters and a maximum extreme distribution for the specificity parameters. To evaluate the potential effects of differential misclassification, we also varied the distribution peaks for the highest and lowest exposure categories. As before, a beta distribution was used for all the sensitivity parameters. However, both maximum and minimum extreme distributions were used for specificity parameters in these scenarios. For each scenario, the specified sensitivity and specificity distributions were sampled using Monte Carlo techniques. The inverse matrix of the sampled classification proportions was then multiplied by the vector of observed cell counts to generate a vector of cell counts adjusted for disease misclassification, which were used to calculate an adjusted odds ratio. When misclassification was differential, the geometric mean adjusted odds ratio ranged from 1.9 to 4.9 with study error resulting in bias both away from and toward the null. Under the assumption of non-differential misclassification, the geometric mean adjusted odds ratio was slightly smaller than the unadjusted estimate. Probabilistic bias analysis can be a useful tool for evaluating study error in historical cohort mortality studies.
MoreTranslated text
Key words
Cohort Study
AI Read Science
Must-Reading Tree
Example
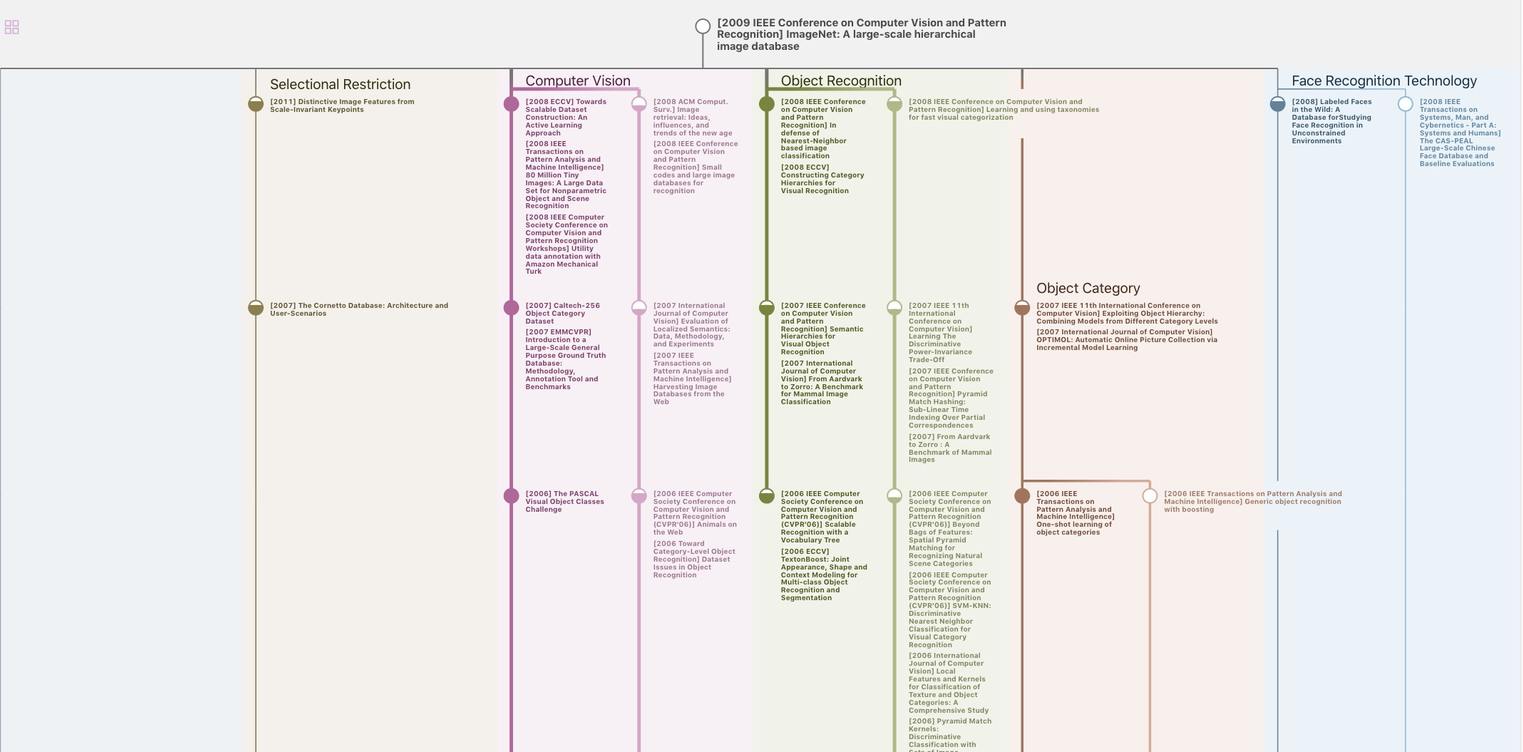
Generate MRT to find the research sequence of this paper
Chat Paper
Summary is being generated by the instructions you defined