Prolsfeo-Ldl: Prototype Selection And Label- Specific Feature Evolutionary Optimization For Label Distribution Learning
APPLIED SCIENCES-BASEL(2020)
摘要
Label Distribution Learning (LDL) is a general learning framework that assigns an instance to a distribution over a set of labels rather than to a single label or multiple labels. Current LDL methods have proven their effectiveness in many real-life machine learning applications. In LDL problems, instance-based algorithms and particularly the adapted version of the k-nearest neighbors method for LDL (AA-kNN) has proven to be very competitive, achieving acceptable results and allowing an explainable model. However, it suffers from several handicaps: it needs large storage requirements, it is not efficient predicting and presents a low tolerance to noise. The purpose of this paper is to mitigate these effects by adding a data reduction stage. The technique devised, called Prototype selection and Label-Specific Feature Evolutionary Optimization for LDL (ProLSFEO-LDL), is a novel method to simultaneously address the prototype selection and the label-specific feature selection pre-processing techniques. Both techniques pose a complex optimization problem with a huge search space. Therefore, we have proposed a search method based on evolutionary algorithms that allows us to obtain a solution to both problems in a reasonable time. The effectiveness of the proposed ProLSFEO-LDL method is verified on several real-world LDL datasets, showing significant improvements in comparison with using raw datasets.
更多查看译文
关键词
label distribution learning, evolutionary optimization, protoype selection, label-specific feature, machine learning
AI 理解论文
溯源树
样例
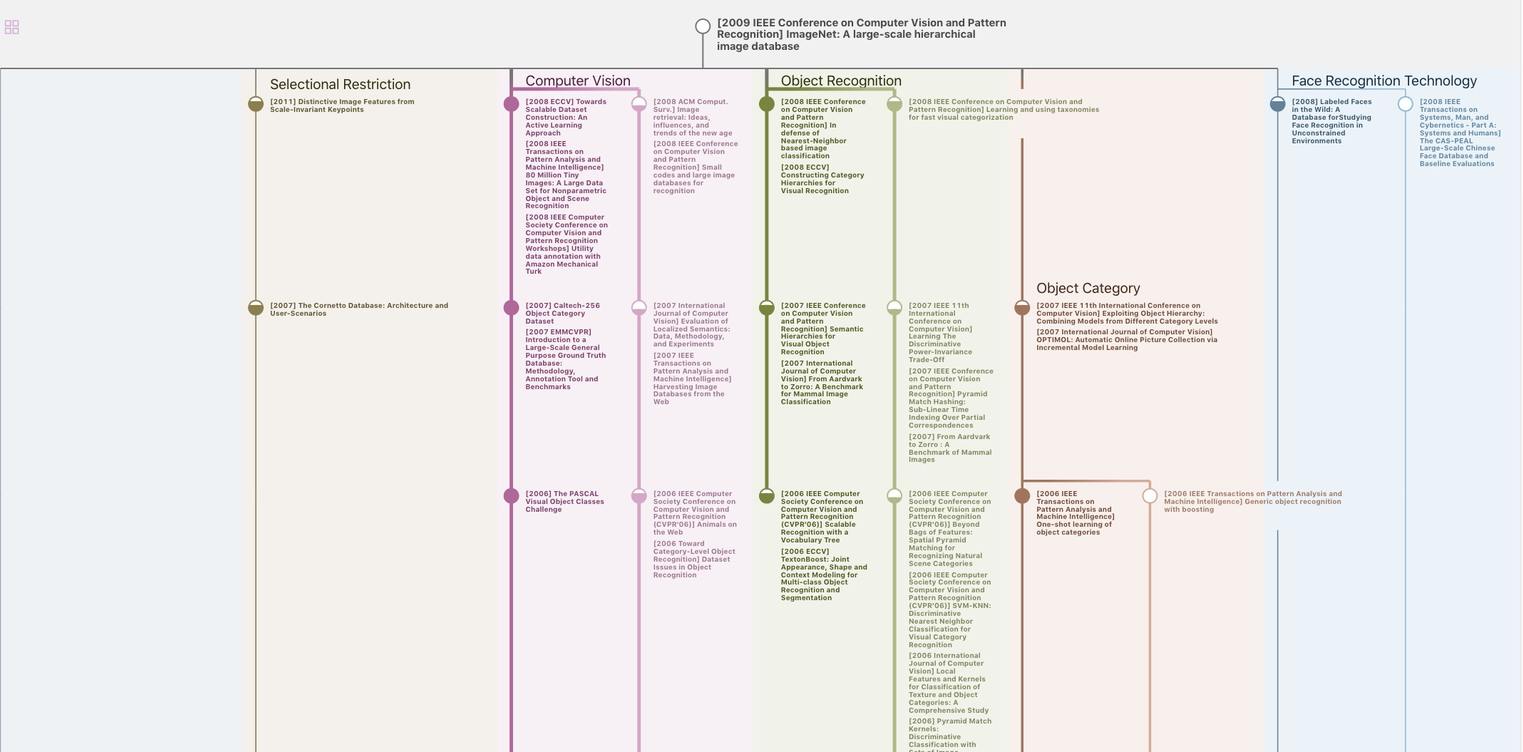
生成溯源树,研究论文发展脉络
Chat Paper
正在生成论文摘要