Combining Deep Neural Networks for Protein Secondary Structure Prediction
IEEE ACCESS(2020)
摘要
By combining convolutional neural networks (CNN) and long short term memory networks (LSTM) into the learning structure, this paper presents a supervised learning method called combining deep neural networks (CDNN) for protein secondary structure prediction. First, we use multiple convolutional neural networks with different number of layers and different size of filters to extract the protein secondary structure features. Second, we use bidirectional LSTM to extract features continually based on the raw features and the features which extracted by CNNs. Third, a fully connected dense layer is used to map the extracted features by LSTM to the different protein secondary structure classes. CDNN architecture is trained by RMSProp optimizer based on the cross entropy error between protein secondary structure labels and dense layer & x2019;s outputs. CDNN not only inherits the abstraction ability of CNN and sequence data process ability of LSTM, but also demonstrates the attractive classification ability for handling protein secondary structure data. The empirical validation on two protein secondary structure prediction datasets demonstrates the effectiveness of CDNN method.
更多查看译文
关键词
Deep learning,supervised learning,prediction,protein secondary structure
AI 理解论文
溯源树
样例
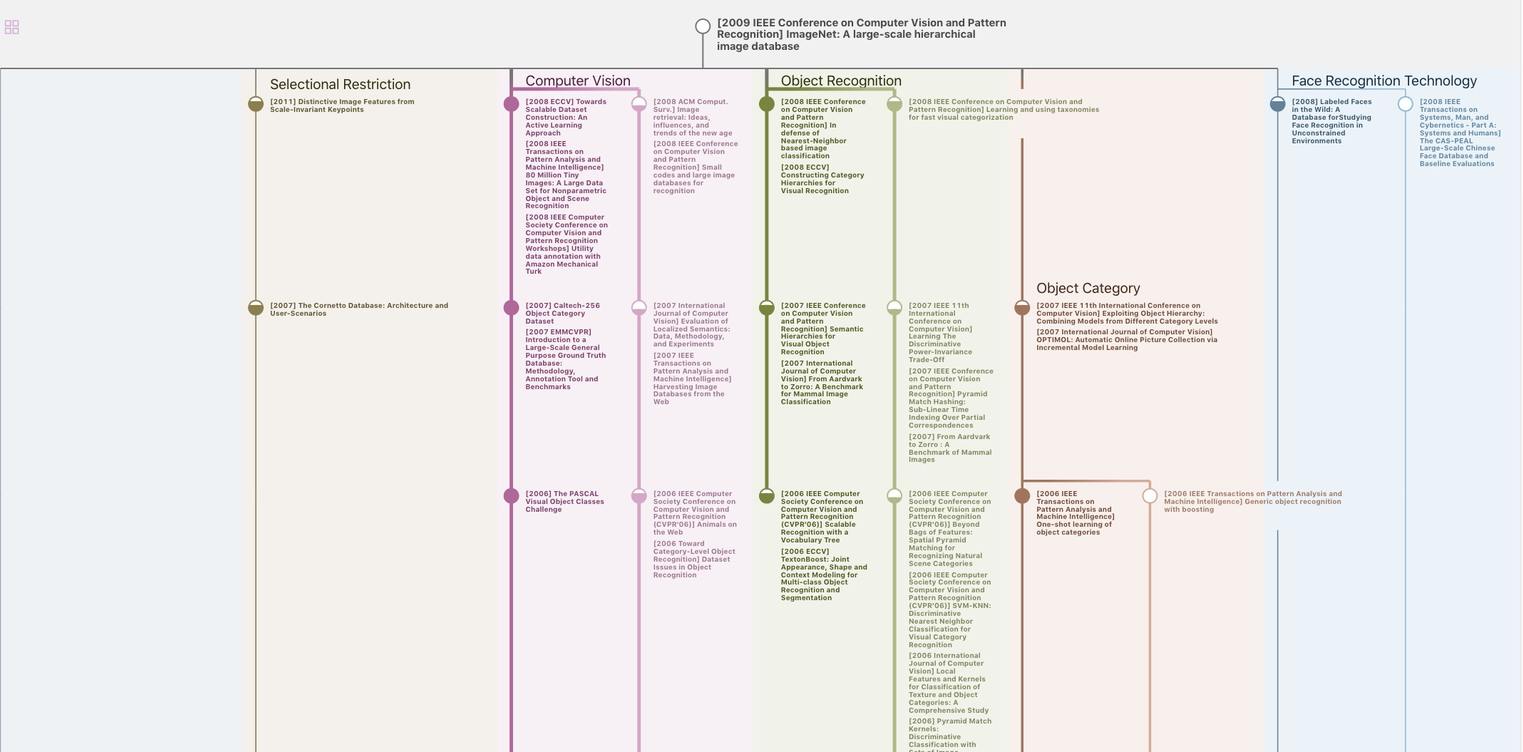
生成溯源树,研究论文发展脉络
Chat Paper
正在生成论文摘要