Learning Causal Representations for Robust Domain Adaptation
IEEE Transactions on Knowledge and Data Engineering(2023)
摘要
In this study, we investigate a challenging problem, namely, robust domain adaptation, where data from only a single well-labeled source domain are available in the training phase. To address this problem, assuming that the causal relationships between the features and the class variable are robust across domains, we propose a novel causal autoencoder (CAE), which integrates a deep autoencoder and a causal structure learning model to learn causal representations using data from a single source domain. Specifically, a deep autoencoder model is adopted to learn the low-dimensional representations, and a causal structure learning model is designed to separate the low-dimensional representations into two groups: causal representations and task-irrelevant representations. Using three real-world datasets, the experiments have validated the effectiveness of CAE, in comparison with eleven state-of-the-art methods.
更多查看译文
关键词
Domain adaptation,causal discovery,autoencoder
AI 理解论文
溯源树
样例
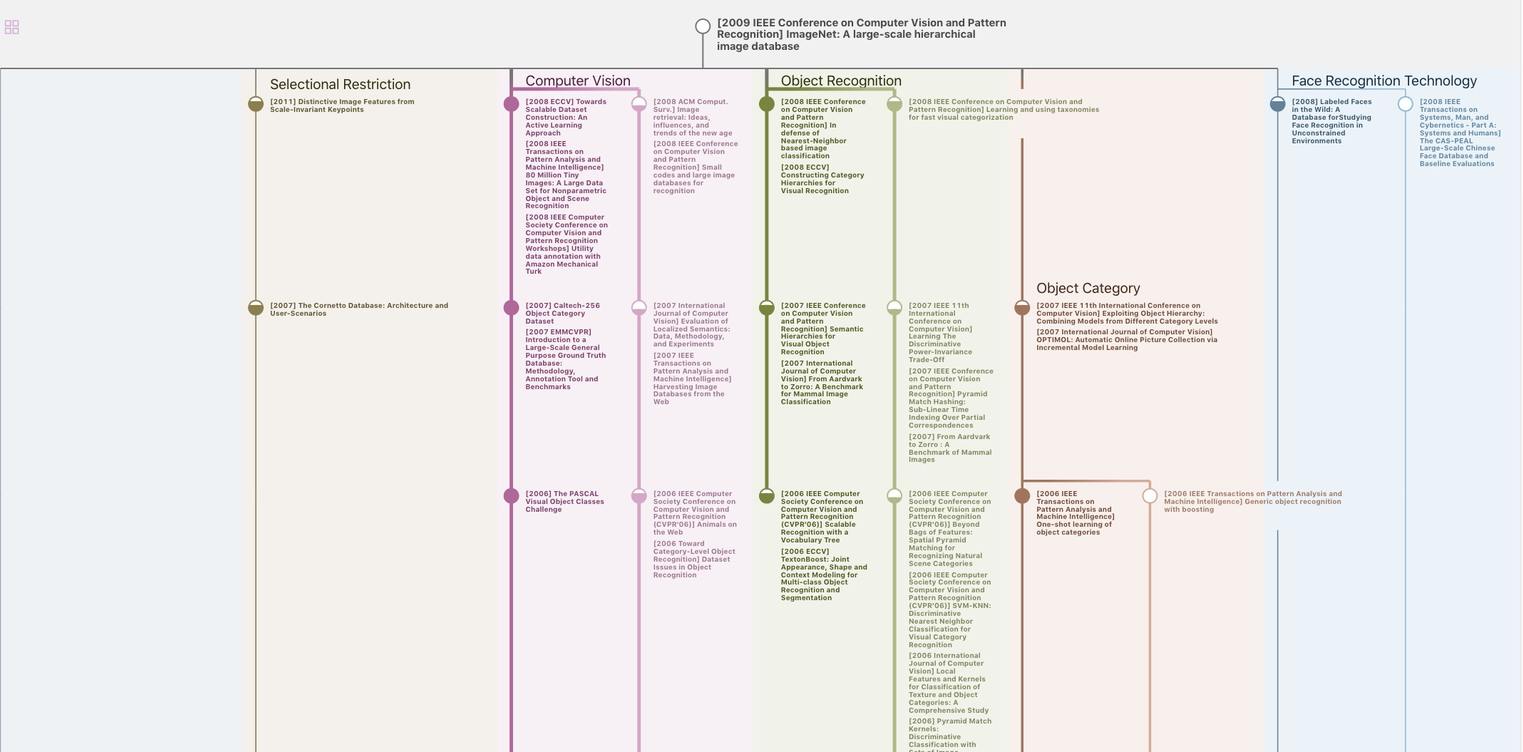
生成溯源树,研究论文发展脉络
Chat Paper
正在生成论文摘要