A Novel Privacy-Preserved Recommender System Framework based on Federated Learning
ICSIM(2021)
摘要
Recommender System (RS) is currently an effective way to solve information overload. To meet users' next click behavior, RS needs to collect users' personal information and behavior to achieve a comprehensive and profound user preference perception. However, these centrally collected data are privacy-sensitive, and any leakage may cause severe problems to both users and service providers. This paper proposed a novel privacy-preserved recommender system framework (PPRSF), through the application of federated learning paradigm, to enable the recommendation algorithm to be trained and carry out inference without centrally collecting users' private data. The PPRSF not only able to reduces the privacy leakage risk, satisfies legal and regulatory requirements but also allows various recommendation algorithms to be applied.
更多查看译文
关键词
federated learning,recommender system framework,privacy-preserved
AI 理解论文
溯源树
样例
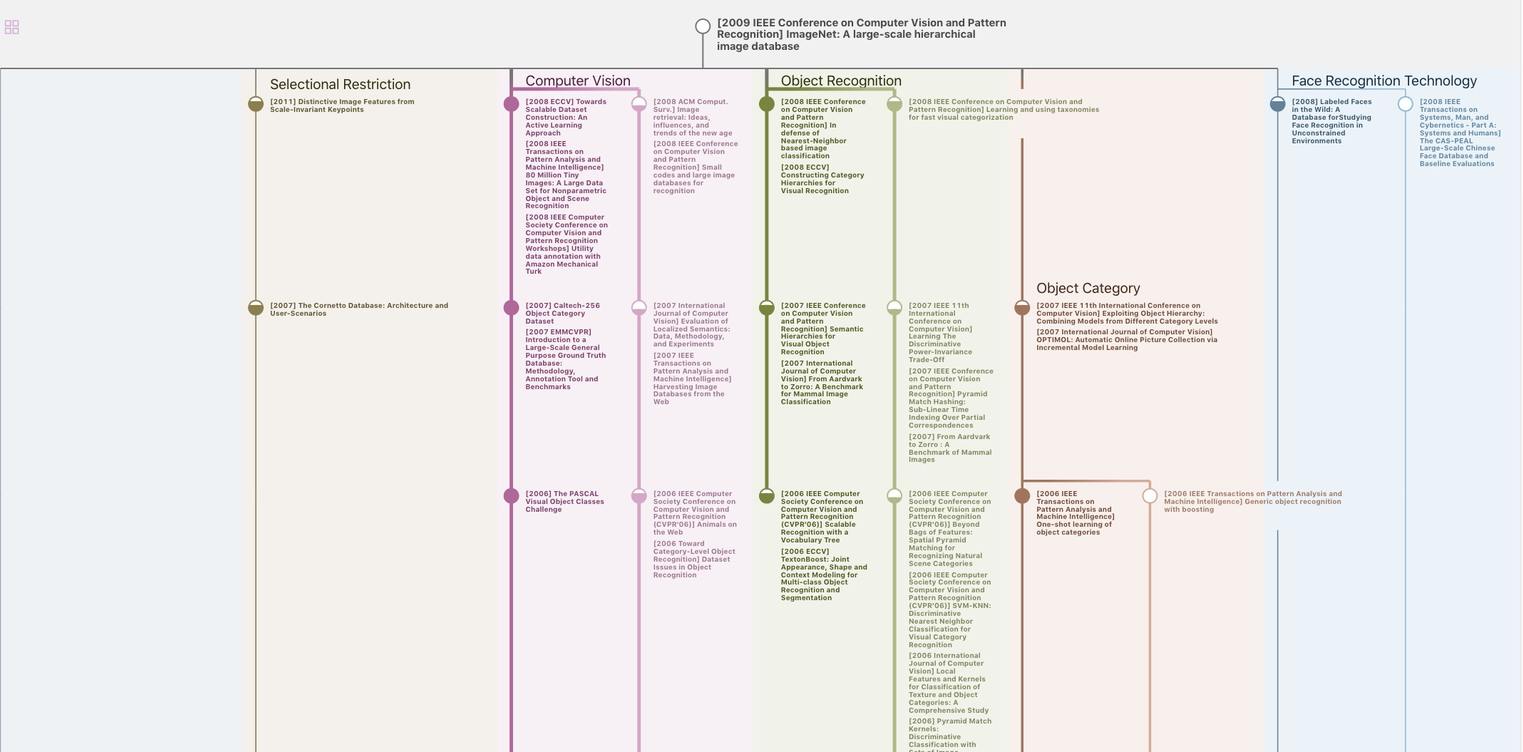
生成溯源树,研究论文发展脉络
Chat Paper
正在生成论文摘要