An Ensemble-Based Approach By Fine-Tuning The Deep Transfer Learning Models To Classify Pneumonia From Chest X-Ray Images
ICAART: PROCEEDINGS OF THE 13TH INTERNATIONAL CONFERENCE ON AGENTS AND ARTIFICIAL INTELLIGENCE - VOL 2(2021)
Abstract
Pneumonia is caused by viruses, bacteria, or fungi that infect the lungs, which, if not diagnosed and treated in time, can be fatal and lead to respiratory failure. More than 250,000 individuals in the United States, mainly adults, are diagnosed with pneumonia each year, and 50,000 die from the disease. Chest Radiography (X-ray) is widely used by radiologists to detect pneumonia. It is not uncommon to overlook pneumonia detection for a well-trained radiologist, which triggers the need for improvement in the accuracy of the diagnosis. Therefore, we propose using transfer learning, which can reduce the neural network's training time and minimize the generalization error to improve the accuracy of the diagnosis. We trained, fine-tuned the state-of-the-art deep learning models such as InceptionResNet, MobileNetV2, Xception, DenseNet201, and ResNet152V2 to classify pneumonia accurately. Later, we created a weighted average ensemble of these models and achieved a test accuracy of 98.46%, precision of 98.38%, recall of 99.53%, and f1 score of 98.96%. These performance metrics of accuracy, precision, and f1 score are at their highest levels ever reported in the literature, which can be considered a benchmark for the accurate pneumonia classification.
MoreTranslated text
Key words
Pneumonia Classification, Deep Learning, Transfer Learning, Chest X-Ray, Medical Imaging, Computer Vision
AI Read Science
Must-Reading Tree
Example
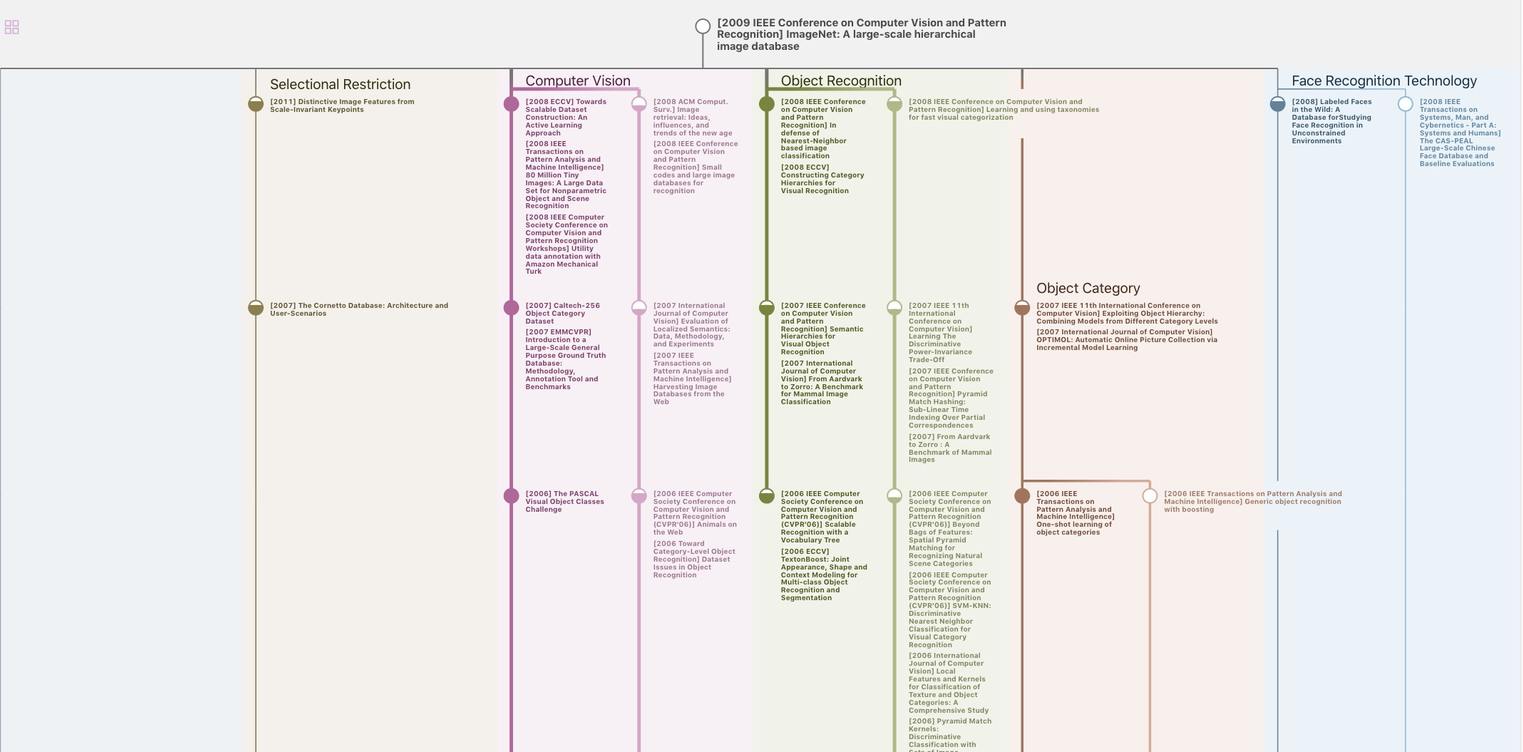
Generate MRT to find the research sequence of this paper
Chat Paper
Summary is being generated by the instructions you defined