Zero-Shot Terrain Generalization for Visual Locomotion Policies
CoRR(2020)
Abstract
Legged robots have unparalleled mobility on unstructured terrains. However, it remains an open challenge to design locomotion controllers that can operate in a large variety of environments. In this paper, we address this challenge of automatically learning locomotion controllers that can generalize to a diverse collection of terrains often encountered in the real world. We frame this challenge as a multi-task reinforcement learning problem and define each task as a type of terrain that the robot needs to traverse. We propose an end-to-end learning approach that makes direct use of the raw exteroceptive inputs gathered from a simulated 3D LiDAR sensor, thus circumventing the need for ground-truth heightmaps or preprocessing of perception information. As a result, the learned controller demonstrates excellent zero-shot generalization capabilities and can navigate 13 different environments, including stairs, rugged land, cluttered offices, and indoor spaces with humans.
MoreTranslated text
Key words
terrain,visual,generalization,zero-shot
AI Read Science
Must-Reading Tree
Example
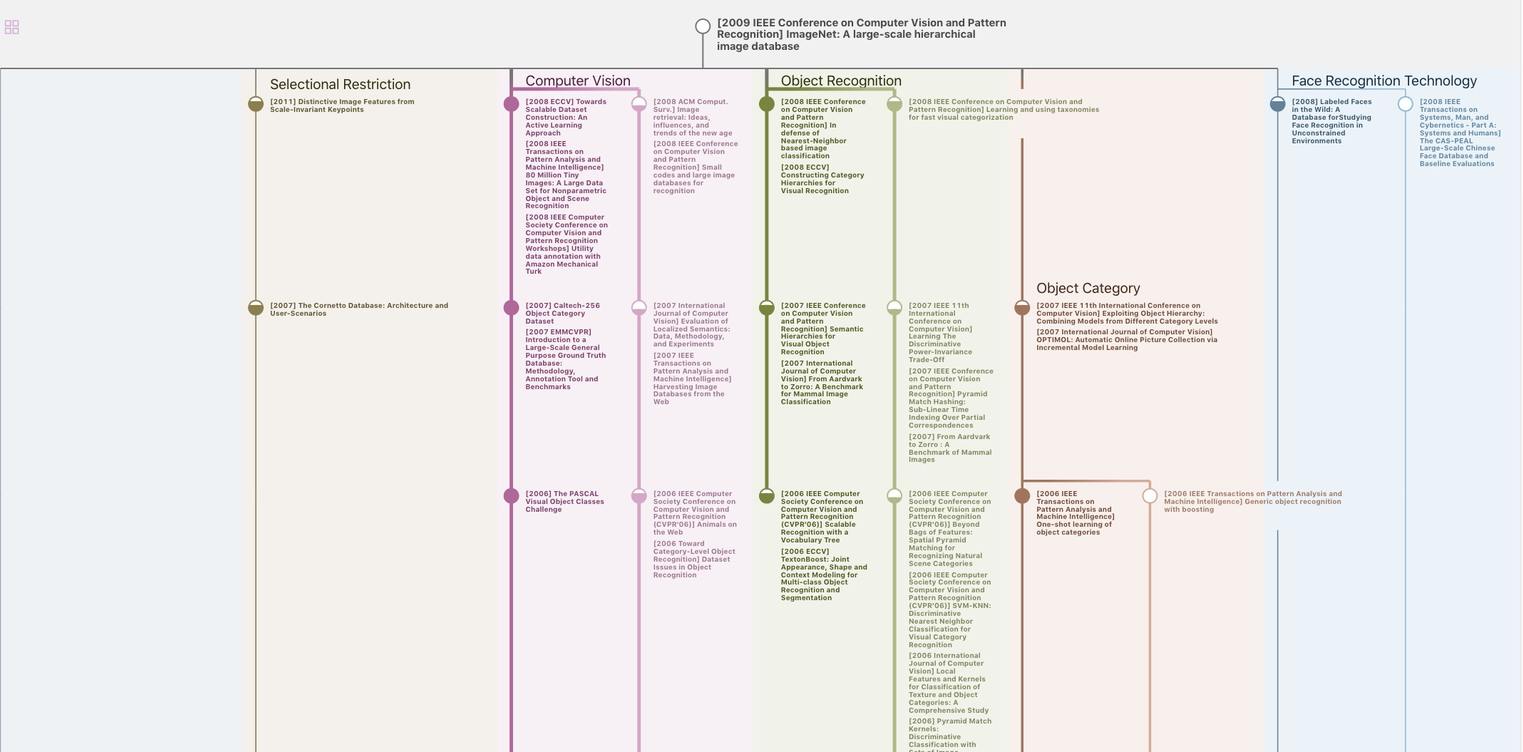
Generate MRT to find the research sequence of this paper
Chat Paper
Summary is being generated by the instructions you defined