Random Sampling Neural Network For Quantum Many-Body Problems
PHYSICAL REVIEW B(2021)
摘要
The eigenvalue problem of quantum many-body systems is a fundamental and challenging subject in condensed-matter physics because the dimension of the Hilbert space (and hence the required computational memory and time) grows exponentially as the system size increases. A few numerical methods have been developed for some specific systems but may not be applicable in others. Here we propose a general numerical method, random sampling neural networks (RSNNs), to utilize the pattern recognition technique for the random sampling matrix elements of an interacting many-body system via a self-supervised learning approach. Several exactly solvable one-dimensional models, including the Ising model with a transverse field, the Fermi-Hubbard model, and the spin-1/2 XXZ model, are used to test the applicability of RSNN. Pretty high accuracy (>96%) of energy spectra, magnetization, critical exponents, etc. can be obtained within the strongly correlated regime or near the quantum phase-transition point, even the corresponding RSNN models are trained in the weakly interacting regime. After including data augmentation and transfer learning methods, RSNN can be further applied to systems of larger sizes with much less training data if only pretrained in a smaller system. Our results demonstrate the possibility to combine the existing numerical methods and the RSNN to explore quantum many-body problems in a much wider parameter space.
更多查看译文
AI 理解论文
溯源树
样例
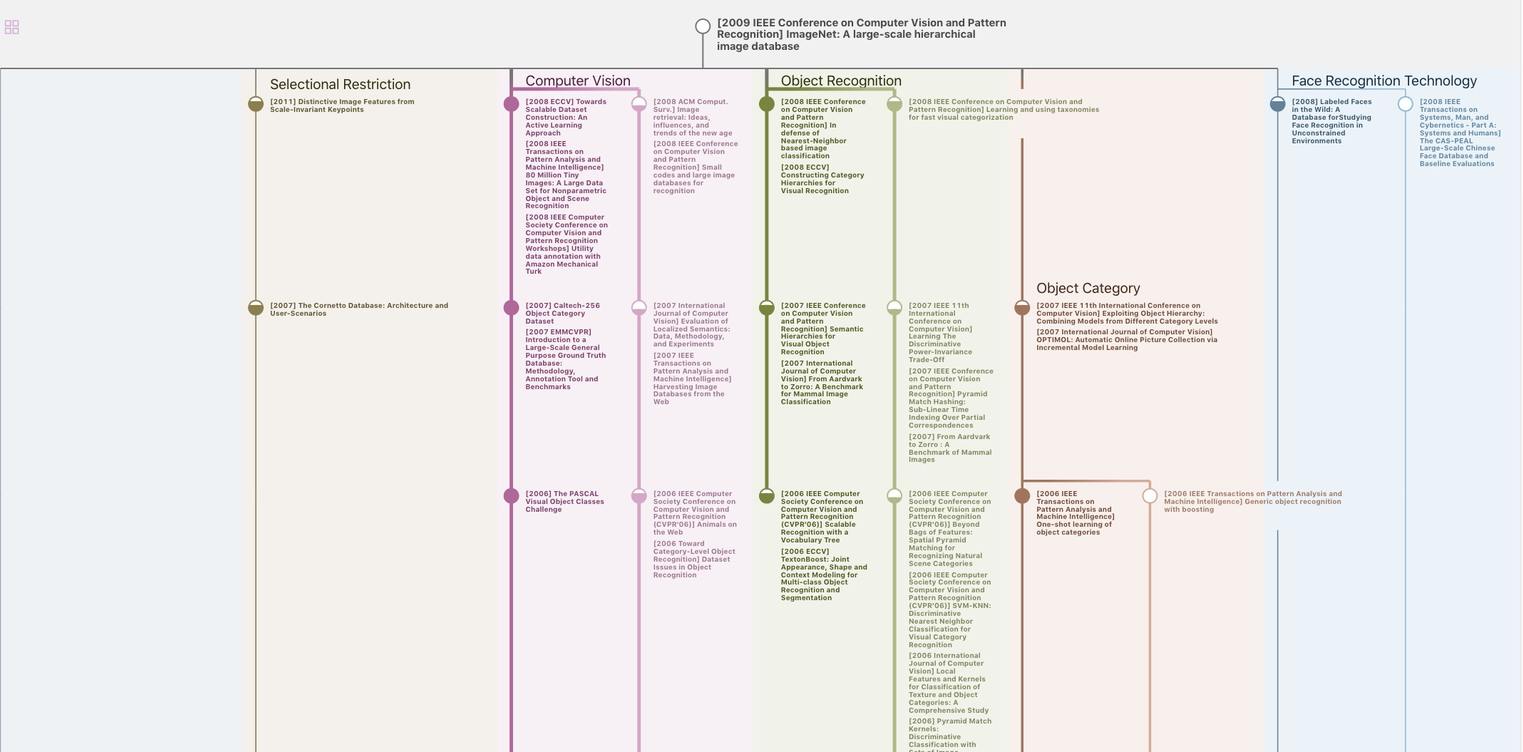
生成溯源树,研究论文发展脉络
Chat Paper
正在生成论文摘要