Mitigating Leakage in Federated Learning with Trusted Hardware
arxiv(2020)
摘要
In federated learning, multiple parties collaborate in order to train a global model over their respective datasets. Even though cryptographic primitives (e.g., homomorphic encryption) can help achieve data privacy in this setting, some partial information may still be leaked across parties if this is done non-judiciously. In this work, we study the federated learning framework of SecureBoost [Cheng et al., FL@IJCAI'19] as a specific such example, demonstrate a leakage-abuse attack based on its leakage profile, and experimentally evaluate the effectiveness of our attack. We then propose two secure versions relying on trusted execution environments. We implement and benchmark our protocols to demonstrate that they are 1.2-5.4X faster in computation and need 5-49X less communication than SecureBoost.
更多查看译文
关键词
federated learning,trusted hardware,leakage
AI 理解论文
溯源树
样例
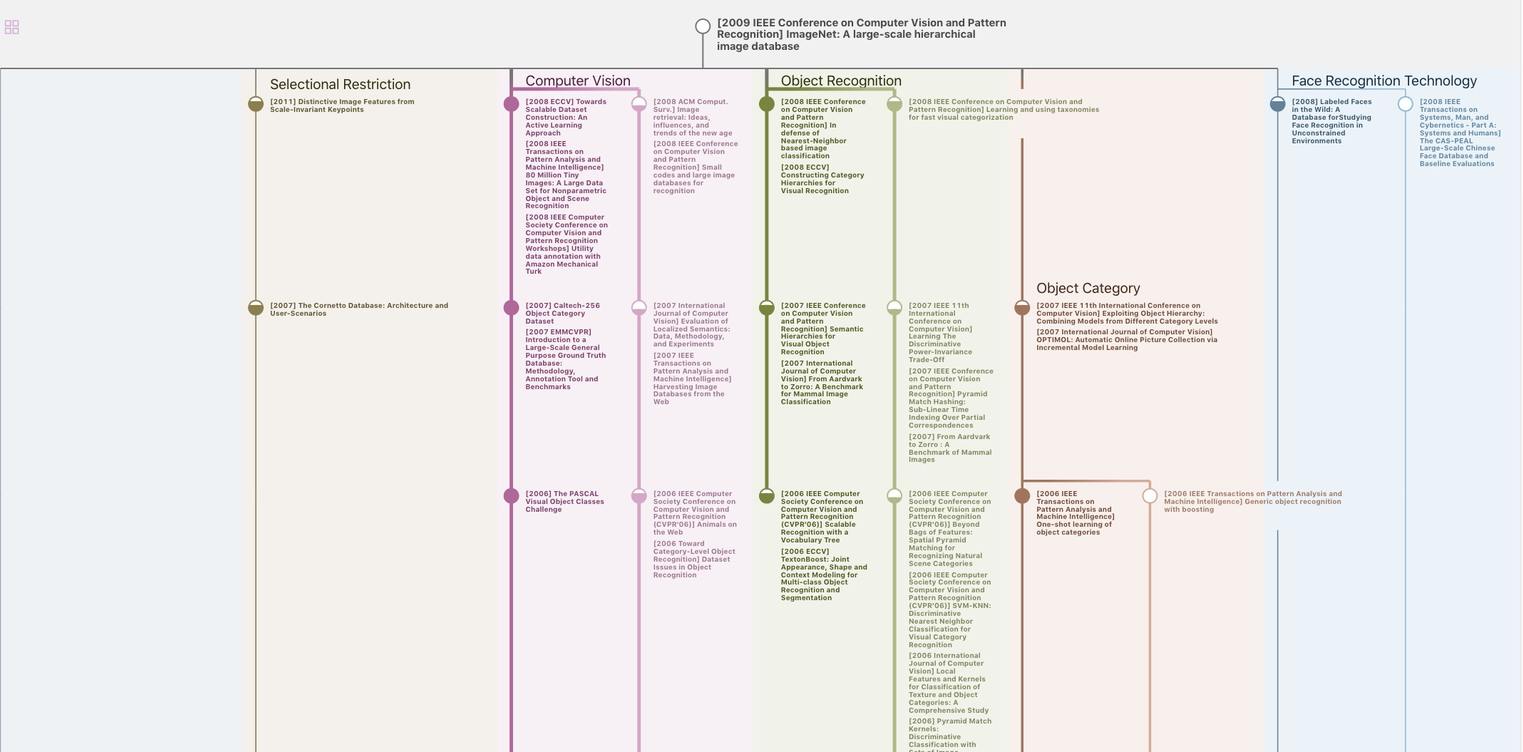
生成溯源树,研究论文发展脉络
Chat Paper
正在生成论文摘要