TMMF: Temporal Multi-Modal Fusion for Single-Stage Continuous Gesture Recognition.
IEEE TRANSACTIONS ON IMAGE PROCESSING(2021)
摘要
Gesture recognition is a much studied research area which has myriad real-world applications including robotics and human-machine interaction. Current gesture recognition methods have focused on recognising isolated gestures, and existing continuous gesture recognition methods are limited to two-stage approaches where independent models are required for detection and classification, with the performance of the latter being constrained by detection performance. In contrast, we introduce a single-stage continuous gesture recognition framework, called Temporal Multi-Modal Fusion (TMMF), that can detect and classify multiple gestures in a video via a single model. This approach learns the natural transitions between gestures and non-gestures without the need for a pre-processing segmentation step to detect individual gestures. To achieve this, we introduce a multi-modal fusion mechanism to support the integration of important information that flows from multi-modal inputs, and is scalable to any number of modes. Additionally, we propose Unimodal Feature Mapping (UFM) and Multi-modal Feature Mapping (MFM) models to map uni-modal features and the fused multi-modal features respectively. To further enhance performance, we propose a mid-point based loss function that encourages smooth alignment between the ground truth and the prediction, helping the model to learn natural gesture transitions. We demonstrate the utility of our proposed framework, which can handle variable-length input videos, and outperforms the state-of-the-art on three challenging datasets: EgoGesture, IPN hand and ChaLearn LAP Continuous Gesture Dataset (ConGD). Furthermore, ablation experiments show the importance of different components of the proposed framework.
更多查看译文
关键词
Gesture recognition, Feature extraction, Solid modeling, Streaming media, Semantics, Visualization, Three-dimensional displays, Gesture recognition, spatio-temporal representation learning, temporal convolution networks
AI 理解论文
溯源树
样例
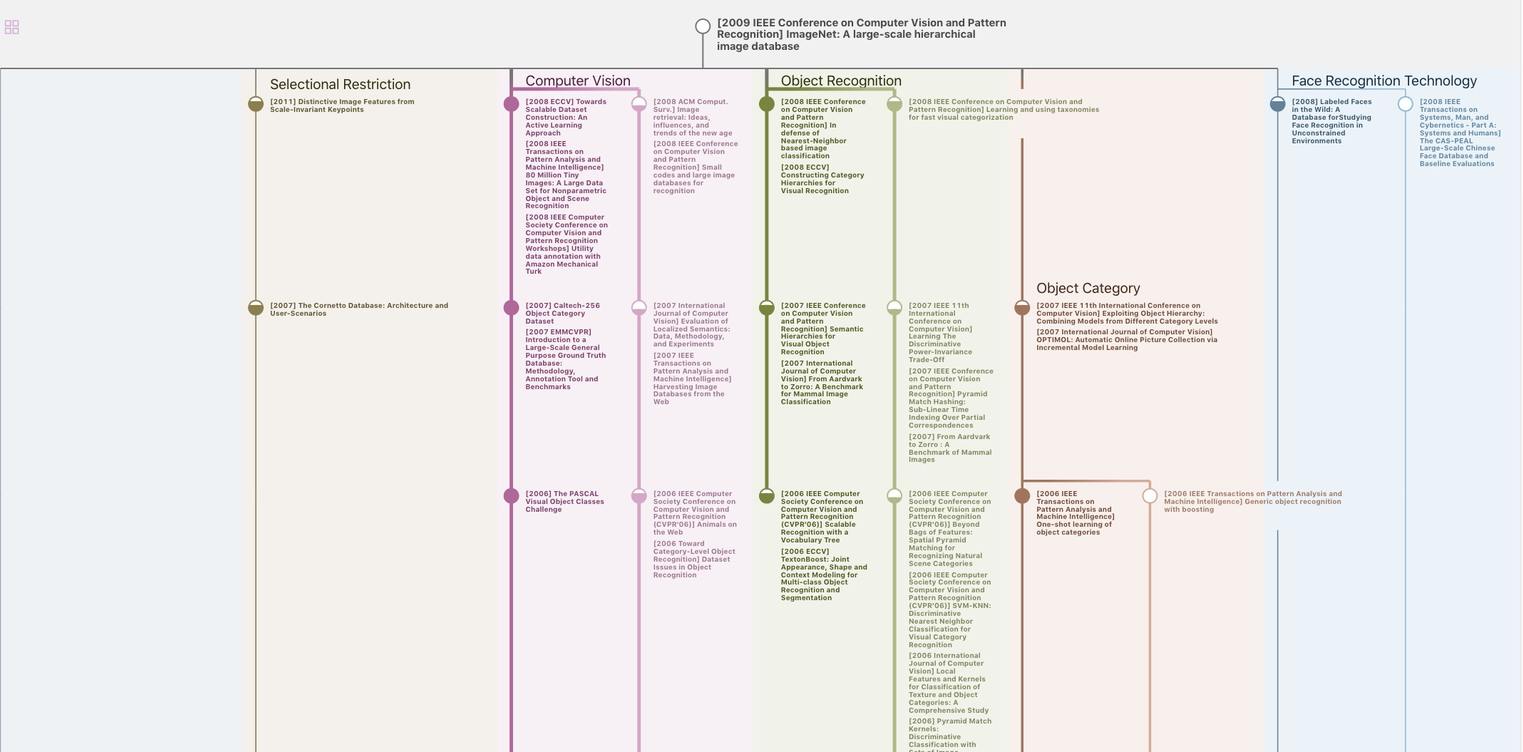
生成溯源树,研究论文发展脉络
Chat Paper
正在生成论文摘要