Robust Batch Policy Learning in Markov Decision Processes
arxiv(2021)
摘要
We consider a varying horizon Markov decision process (MDP), where each policy is evaluated by a set containing average rewards over different horizon lengths with different reference distributions. Given a pre-collected dataset of multiple trajectories generated by some behavior policy, our goal is to learn a robust policy in a pre-specified policy class that can approximately maximize the smallest value of this set. Leveraging semi-parametric statistics, we develop an efficient policy learning method for estimating the defined robust optimal policy that can efficiently break the curse of horizon. A rate-optimal regret bound up to a logarithmic factor is established in terms of the number of trajectories and the number of decision points. Our regret guarantee subsumes the long-term average reward MDP setting as a special case.
更多查看译文
关键词
robust batch policy learning,markov decision processes
AI 理解论文
溯源树
样例
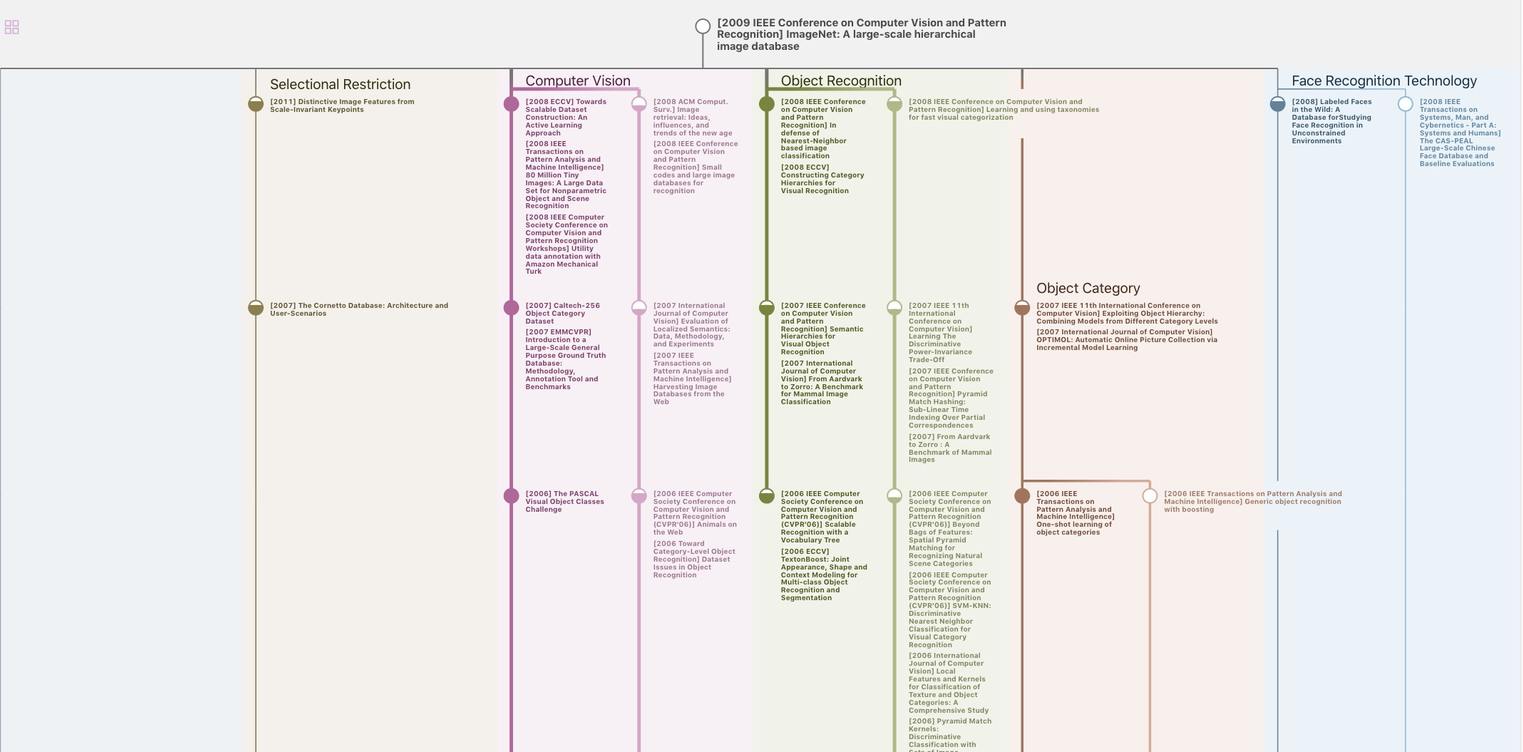
生成溯源树,研究论文发展脉络
Chat Paper
正在生成论文摘要