Provenance-Based Interpretation of Multi-Agent Information Analysis.
TaPP(2020)
摘要
Analytic software tools and workflows are increasing in capability, complexity, number, and scale, and the integrity of our workflows is as important as ever. Specifically, we must be able to inspect the process of analytic workflows to assess (1) confidence of the conclusions, (2) risks and biases of the operations involved, (3) sensitivity of the conclusions to sources and agents, (4) impact and pertinence of various sources and agents, and (5) diversity of the sources that support the conclusions. We present an approach that tracks agents' provenance with PROV-O in conjunction with agents' appraisals and evidence links (expressed in our novel DIVE ontology). Together, PROV-O and DIVE enable dynamic propagation of confidence and counter-factual refutation to improve human-machine trust and analytic integrity. We demonstrate representative software developed for user interaction with that provenance, and discuss key needs for organizations adopting such approaches. We demonstrate all of these assessments in a multi-agent analysis scenario, using an interactive web-based information validation UI.
更多查看译文
关键词
information,interpretation,analysis,provenance-based,multi-agent
AI 理解论文
溯源树
样例
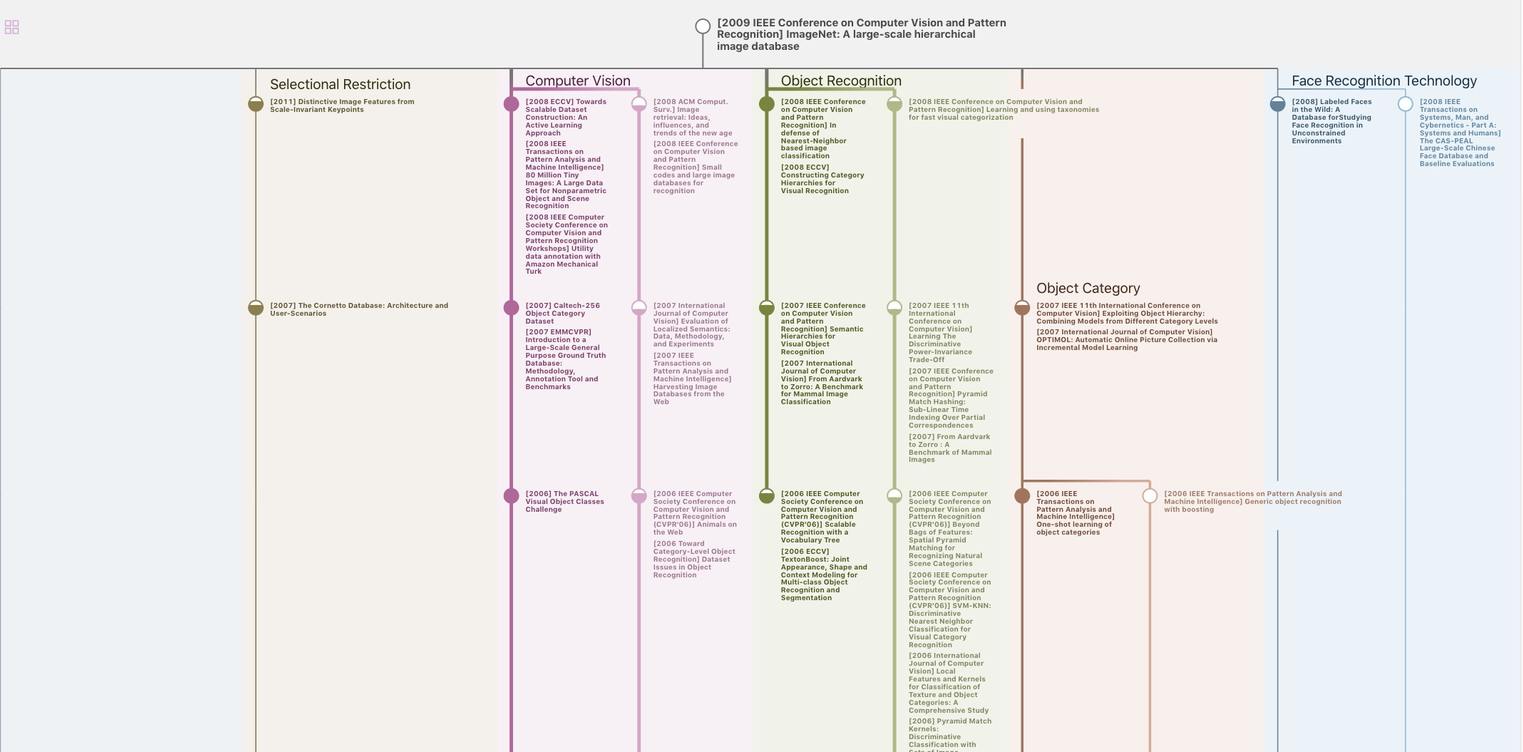
生成溯源树,研究论文发展脉络
Chat Paper
正在生成论文摘要