Structural motifs in protein cores and at protein-protein interfaces are different
PROTEIN SCIENCE(2021)
Abstract
Structures of proteins and protein-protein complexes are determined by the same physical principles and thus share a number of similarities. At the same time, there could be differences because in order to function, proteins interact with other molecules, undergo conformations changes, and so forth, which might impose different restraints on the tertiary versus quaternary structures. This study focuses on structural properties of protein-protein interfaces in comparison with the protein core, based on the wealth of currently available structural data and new structure-based approaches. The results showed that physicochemical characteristics, such as amino acid composition, residue-residue contact preferences, and hydrophilicity/hydrophobicity distributions, are similar in protein core and protein-protein interfaces. On the other hand, characteristics that reflect the evolutionary pressure, such as structural composition and packing, are largely different. The results provide important insight into fundamental properties of protein structure and function. At the same time, the results contribute to better understanding of the ways to dock proteins. Recent progress in predicting structures of individual proteins follows the advancement of deep learning techniques and new approaches to residue coevolution data. Protein core could potentially provide large amounts of data for application of the deep learning to docking. However, our results showed that the core motifs are significantly different from those at protein-protein interfaces, and thus may not be directly useful for docking. At the same time, such difference may help to overcome a major obstacle in application of the coevolutionary data to docking-discrimination of the intramolecular information not directly relevant to docking.
MoreTranslated text
Key words
protein docking,protein modeling,protein recognition,structure prediction
AI Read Science
Must-Reading Tree
Example
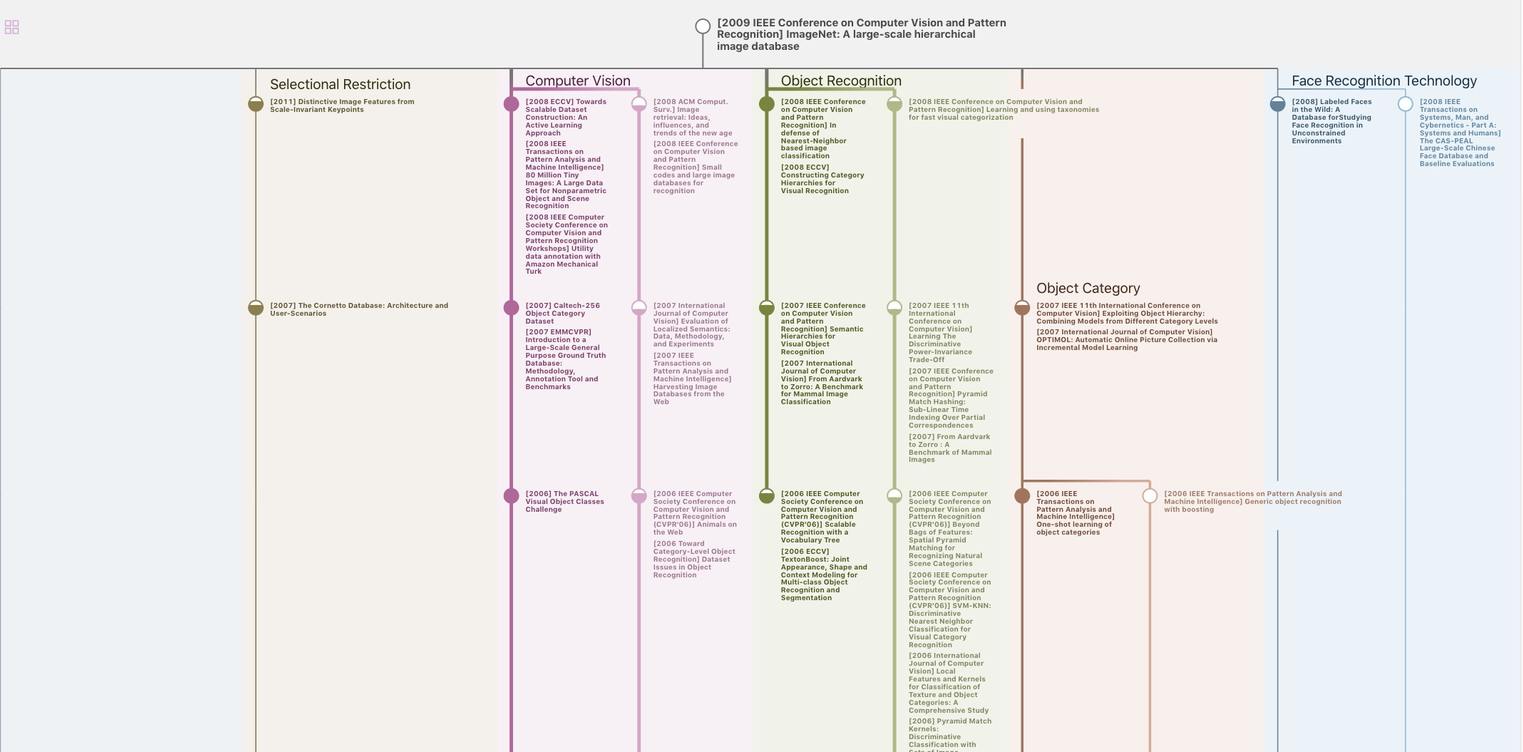
Generate MRT to find the research sequence of this paper
Chat Paper
Summary is being generated by the instructions you defined