Data-Driven Predictive Control Towards Multi-Agent Motion Planning With Non-Parametric Closed-Loop Behavior Learning
arxiv(2023)
摘要
In many specific scenarios, accurate and effective system identification is a commonly encountered challenge in the model predictive control (MPC) formulation. As a consequence, the overall system performance could be significantly weakened in outcome when the traditional MPC algorithm is adopted under those circumstances when such accuracy is lacking. This paper investigates a non-parametric closed-loop behavior learning method for multi-agent motion planning, which underpins a data-driven predictive control framework. Utilizing an innovative methodology with closed-loop input/output measurements of the unknown system, the behavior of the system is learned based on the collected dataset, and thus the constructed non-parametric predictive model can be used to determine the optimal control actions. This non-parametric predictive control framework alleviates the heavy computational burden commonly encountered in the optimization procedures typically in alternate methodologies requiring open-loop input/output measurement data collection and parametric system identification. The proposed data-driven approach is also shown to preserve good robustness properties. Finally, a multi-UAV system is used to demonstrate the highly effective outcome of this promising development.
更多查看译文
关键词
predictive control,chance constraints,decision making,data-driven,multi-agent
AI 理解论文
溯源树
样例
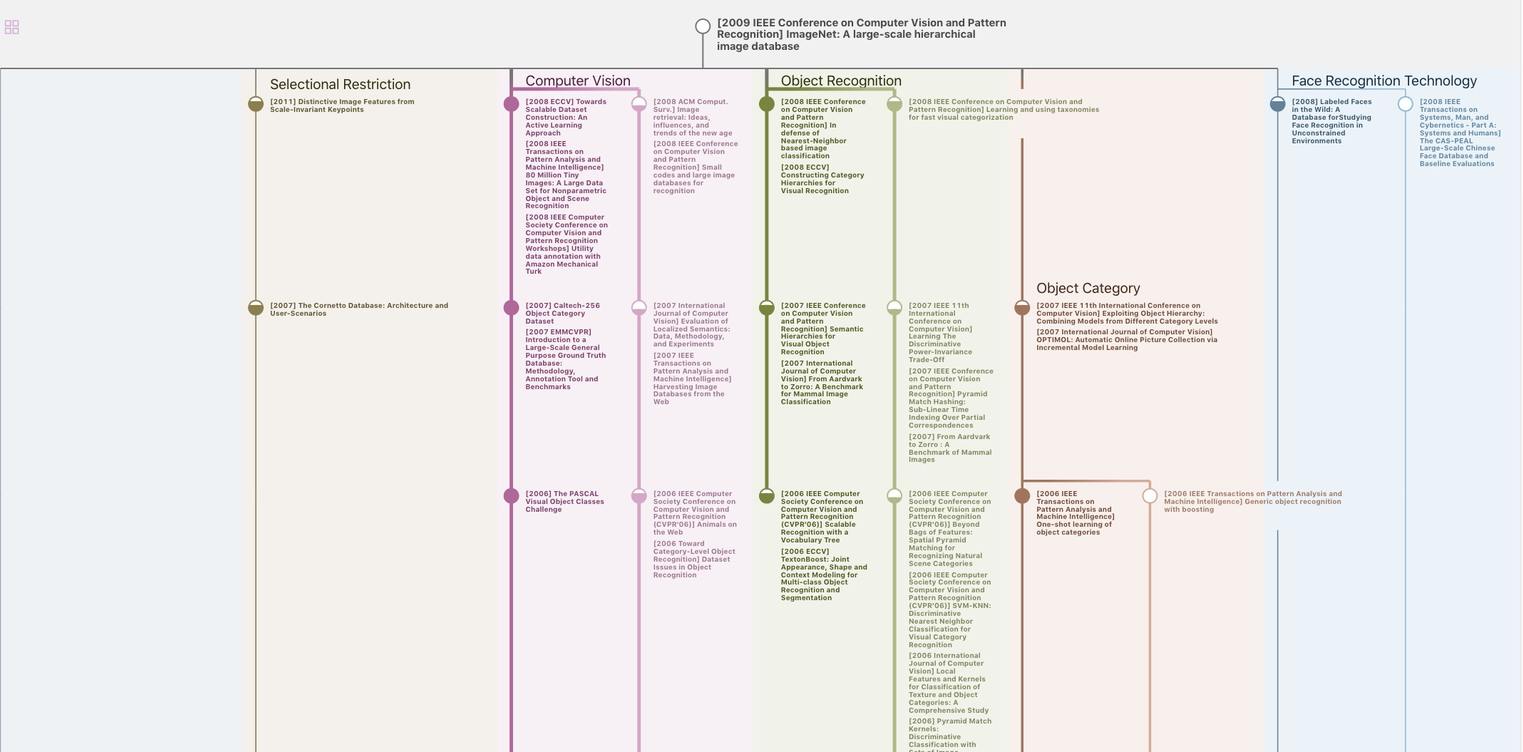
生成溯源树,研究论文发展脉络
Chat Paper
正在生成论文摘要